A Deep Neural Architecture For Sentence Semantic Matching
INTERNATIONAL JOURNAL OF COMPUTATIONAL SCIENCE AND ENGINEERING(2020)
摘要
Sentence semantic matching (SSM) is a fundamental research task in natural language processing. Most existing SSM methods take the advantage of sentence representation learning to generate a single or multi-granularity semantic representation for sentence matching. However, sentence interactions and loss function which are the two key factors for SSM still have not been fully considered. Accordingly, we propose a deep neural network architecture for SSM task with a sentence interactive matching layer and an optimised loss function. Given two input sentences, our model first encodes them to embeddings with an ordinary long short-term memory (LSTM) encoder. Then, the encoded embeddings are handled by an attention layer to find the key and important words in the sentences. Next, sentence interactions are captured with a matching layer to output a matching vector. Finally, based on the matching vector, a fully connected multi-layer perceptron outputs the similarity score. The model also distinguishes the equivocation training instances with an improved optimised loss function. We also systematically evaluate our model on a public Chinese semantic matching corpus, BQ corpus. The results demonstrate that our model outperforms the state-of-the-art methods, i.e., BiMPM, DIIN.
更多查看译文
关键词
sentence matching, representation learning, sentence interaction, loss function, deep neural model, long short-term memory, LSTM
AI 理解论文
溯源树
样例
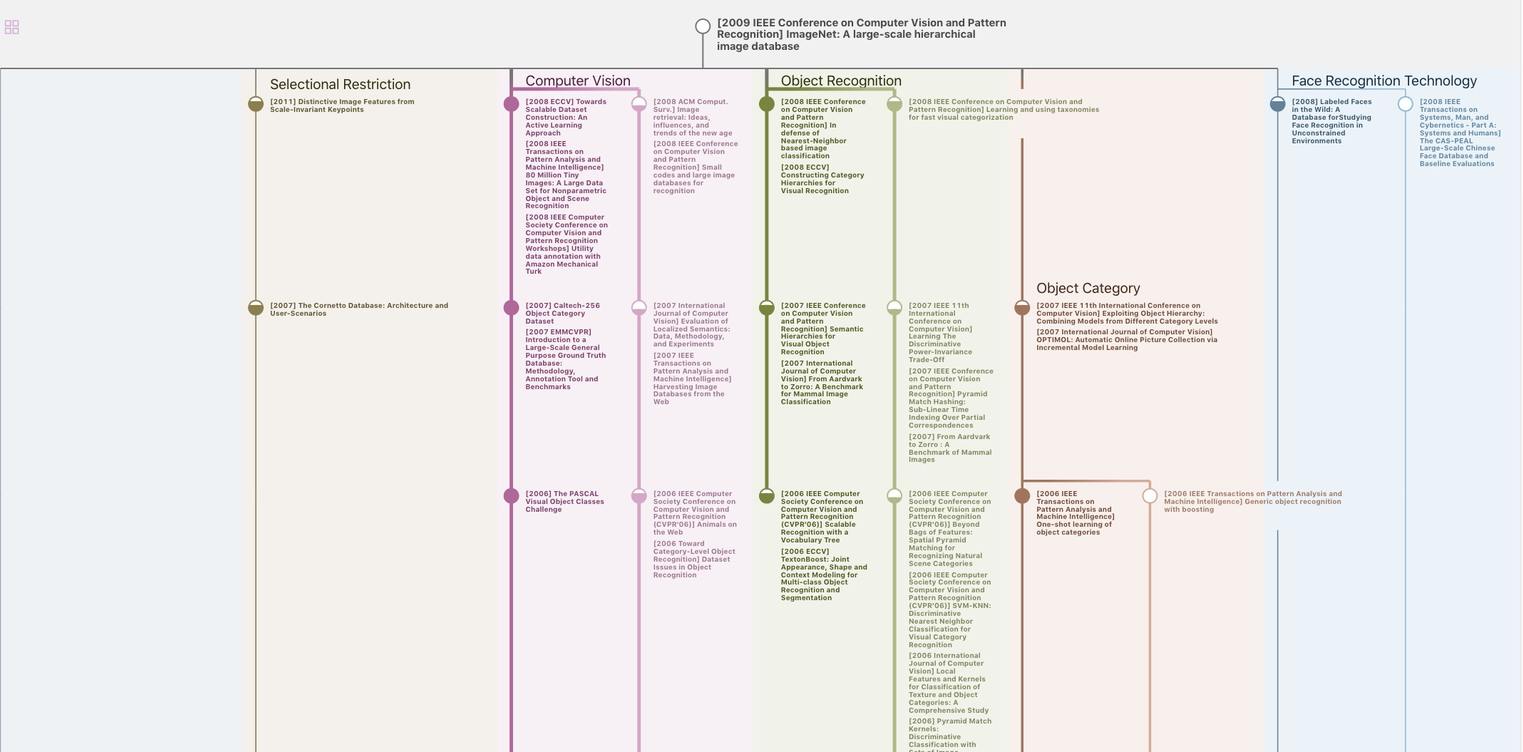
生成溯源树,研究论文发展脉络
Chat Paper
正在生成论文摘要