Semiparametric analysis of clustered interval-censored survival data using soft Bayesian additive regression trees (SBART)
BIOMETRICS(2022)
摘要
Popular parametric and semiparametric hazards regression models for clustered survival data are inappropriate and inadequate when the unknown effects of different covariates and clustering are complex. This calls for a flexible modeling framework to yield efficient survival prediction. Moreover, for some survival studies involving time to occurrence of some asymptomatic events, survival times are typically interval censored between consecutive clinical inspections. In this article, we propose a robust semiparametric model for clustered interval-censored survival data under a paradigm of Bayesian ensemble learning, called soft Bayesian additive regression trees or SBART (Linero and Yang, 2018), which combines multiple sparse (soft) decision trees to attain excellent predictive accuracy. We develop a novel semiparametric hazards regression model by modeling the hazard function as a product of a parametric baseline hazard function and a nonparametric component that uses SBART to incorporate clustering, unknown functional forms of the main effects, and interaction effects of various covariates. In addition to being applicable for left-censored, right-censored, and interval-censored survival data, our methodology is implemented using a data augmentation scheme which allows for existing Bayesian backfitting algorithms to be used. We illustrate the practical implementation and advantages of our method via simulation studies and an analysis of a prostate cancer surgery study where dependence on the experience and skill level of the physicians leads to clustering of survival times. We conclude by discussing our method's applicability in studies involving high-dimensional data with complex underlying associations.
更多查看译文
关键词
Bayesian additive regression trees, machine learning, nonproportional hazards, semiparametric, survival analysis
AI 理解论文
溯源树
样例
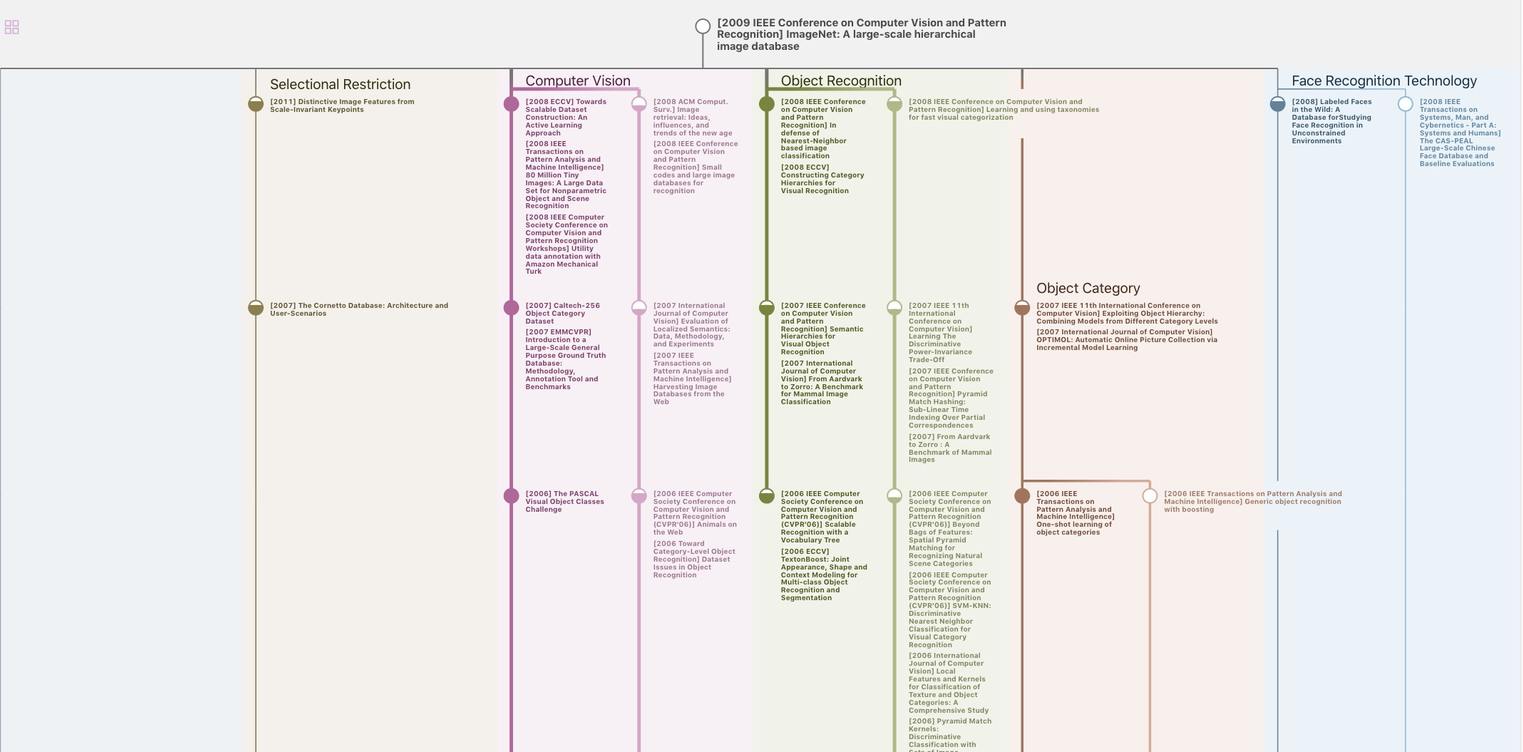
生成溯源树,研究论文发展脉络
Chat Paper
正在生成论文摘要