A Solution for Large Scale Nonlinear Regression with High Rank and Degree at Constant Memory Complexity via Latent Tensor Reconstruction
arxiv(2020)
摘要
This paper proposes a novel method for learning highly nonlinear, multivariate functions from examples. Our method takes advantage of the property that continuous functions can be approximated by polynomials, which in turn are representable by tensors. Hence the function learning problem is transformed into a tensor reconstruction problem, an inverse problem of the tensor decomposition. Our method incrementally builds up the unknown tensor from rank-one terms, which lets us control the complexity of the learned model and reduce the chance of overfitting. For learning the models, we present an efficient gradient-based algorithm that can be implemented in linear time in the sample size, order, rank of the tensor and the dimension of the input. In addition to regression, we present extensions to classification, multi-view learning and vector-valued output as well as a multi-layered formulation. The method can work in an online fashion via processing mini-batches of the data with constant memory complexity. Consequently, it can fit into systems equipped only with limited resources such as embedded systems or mobile phones. Our experiments demonstrate a favorable accuracy and running time compared to competing methods.
更多查看译文
关键词
large scale nonlinear regression,latent tensor reconstruction,constant memory complexity
AI 理解论文
溯源树
样例
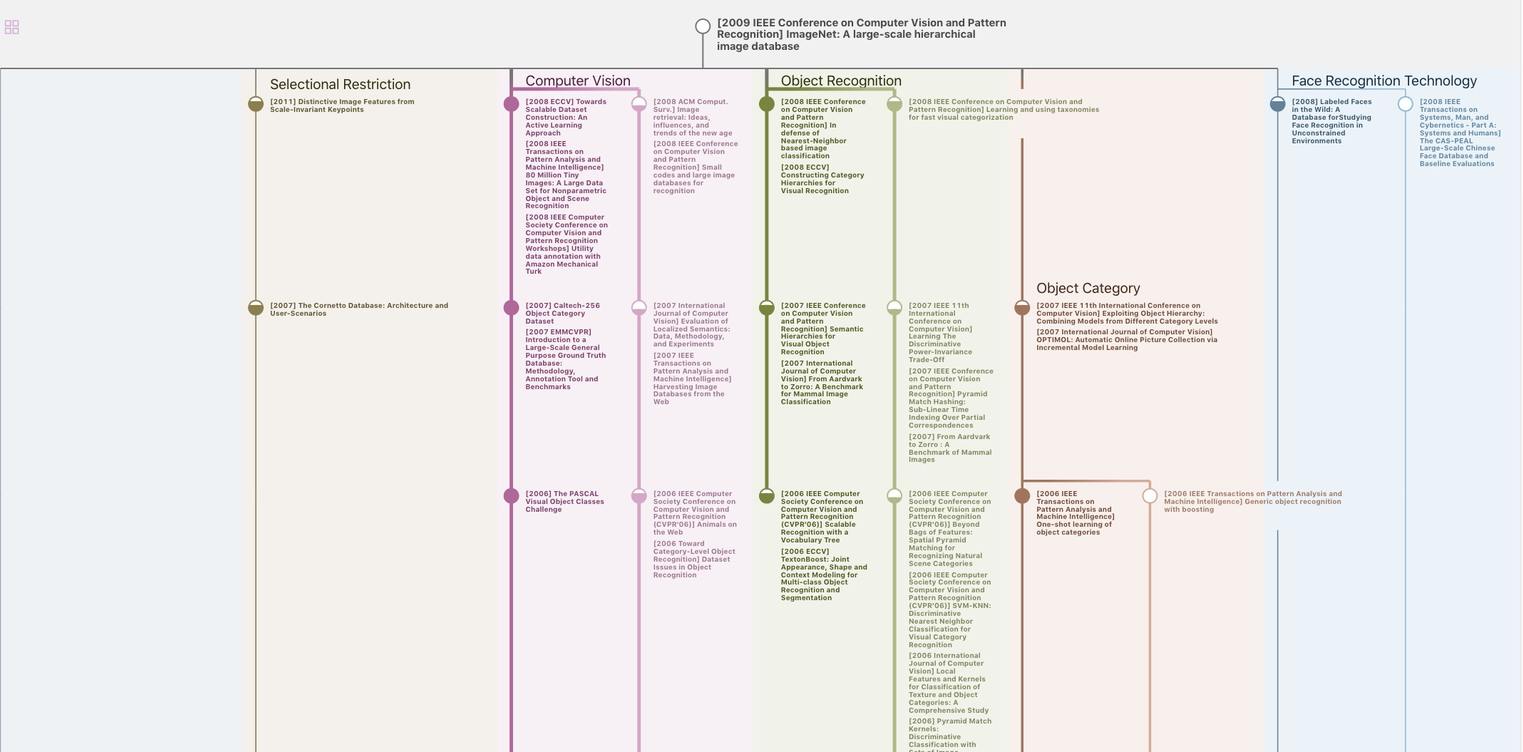
生成溯源树,研究论文发展脉络
Chat Paper
正在生成论文摘要