MeshfreeFlowNet: a physics-constrained deep continuous space-time super-resolution framework
The International Conference for High Performance Computing, Networking, Storage, and Analysis(2020)
摘要
ABSTRACTWe propose MeshfreeFlowNet, a novel deep learning-based super-resolution framework to generate continuous (grid-free) spatio-temporal solutions from the low-resolution inputs. While being computationally efficient, MeshfreeFlowNet accurately recovers the fine-scale quantities of interest. MeshfreeFlowNet allows for: (i) the output to be sampled at all spatio-temporal resolutions, (ii) a set of Partial Differential Equation (PDE) constraints to be imposed, and (iii) training on fixed-size inputs on arbitrarily sized spatio-temporal domains owing to its fully convolutional encoder. We empirically study the performance of MeshfreeFlowNet on the task of super-resolution of turbulent flows in the Rayleigh-Bénard convection problem. Across a diverse set of evaluation metrics, we show that MeshfreeFlowNet significantly outperforms existing baselines. Furthermore, we provide a large scale implementation of MeshfreeFlowNet and show that it efficiently scales across large clusters, achieving 96.80% scaling efficiency on up to 128 GPUs and a training time of less than 4 minutes. We provide an open-source implementation of our method that supports arbitrary combinations of PDE constraints 1.
更多查看译文
关键词
Super-Resolution,PDEs,Physics-Constrained,Deep Neural Networks
AI 理解论文
溯源树
样例
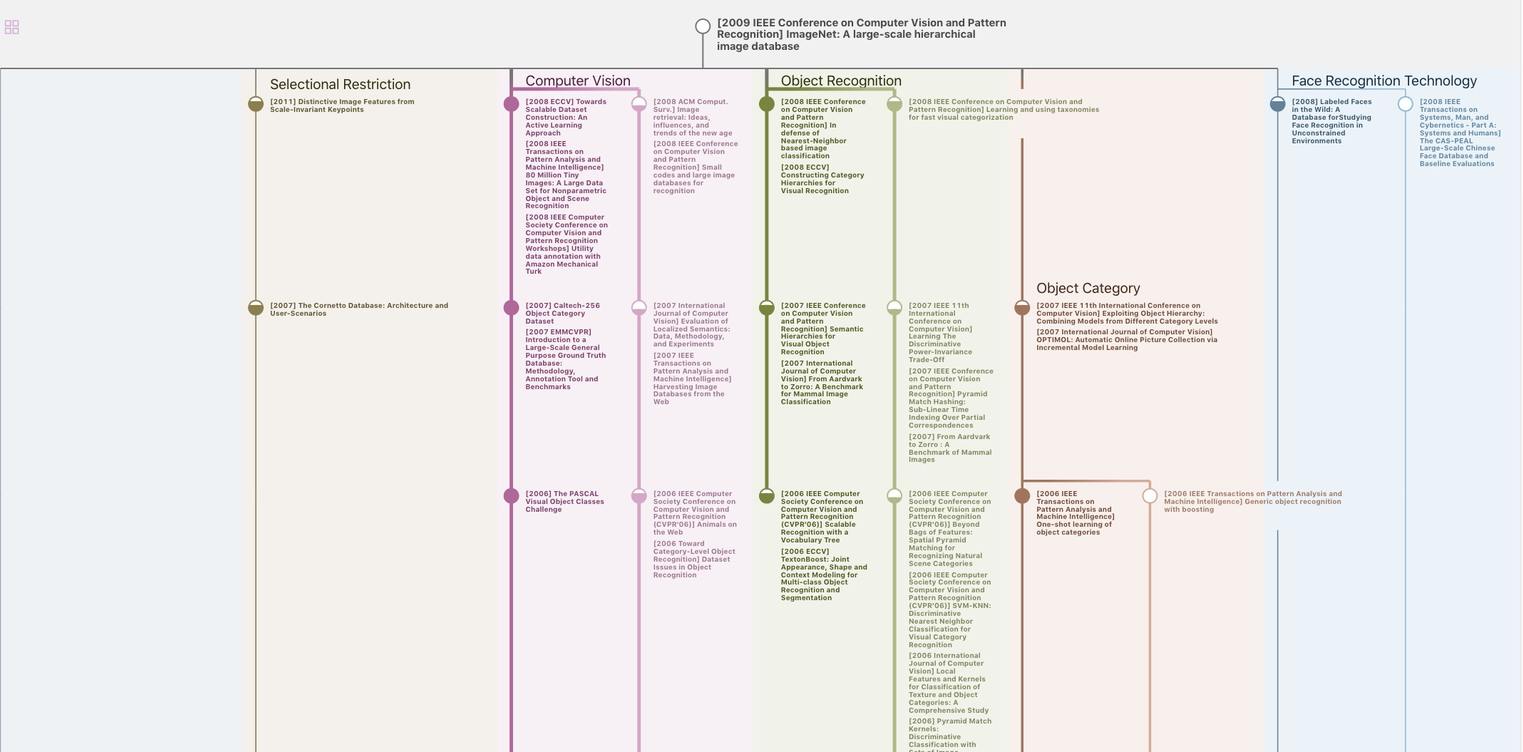
生成溯源树,研究论文发展脉络
Chat Paper
正在生成论文摘要