A Novel Data Cleansing Approach for Sensitive Applications of Wireless Sensor Networks
2019 International Conference on Smart Applications, Communications and Networking (SmartNets)(2019)
摘要
In many applications of wireless sensor networks, data collection happens to be generally unreliable and inaccurate. In order to improve the quality and the accuracy of this collected data, a deep analysis should be applied in order to spot and remove abnormalities that this data may contain, generally referred to as data outliers. This paper tackles this problem and presents a novel Distributed Outlier Detection Approach, dubbed DODA, that works in two steps: (1) first, it models the problem of detecting outliers with a Naïve Bayesian network within each cluster of sensors, and (2) then detects these outliers using a Mahalanobis distance at the cluster heads. Through extensive experiments, conducted on real datasets from Intel-Berkeley Laboratory, we demonstrated that the proposal performs better than the recent state-of-the-art algorithms under several metrics such energy consumption, false alarm rate and detection accuracy.
更多查看译文
关键词
Wireless sensor networks,naïve Bayesian network,Mahalanobis distance,outlier detection
AI 理解论文
溯源树
样例
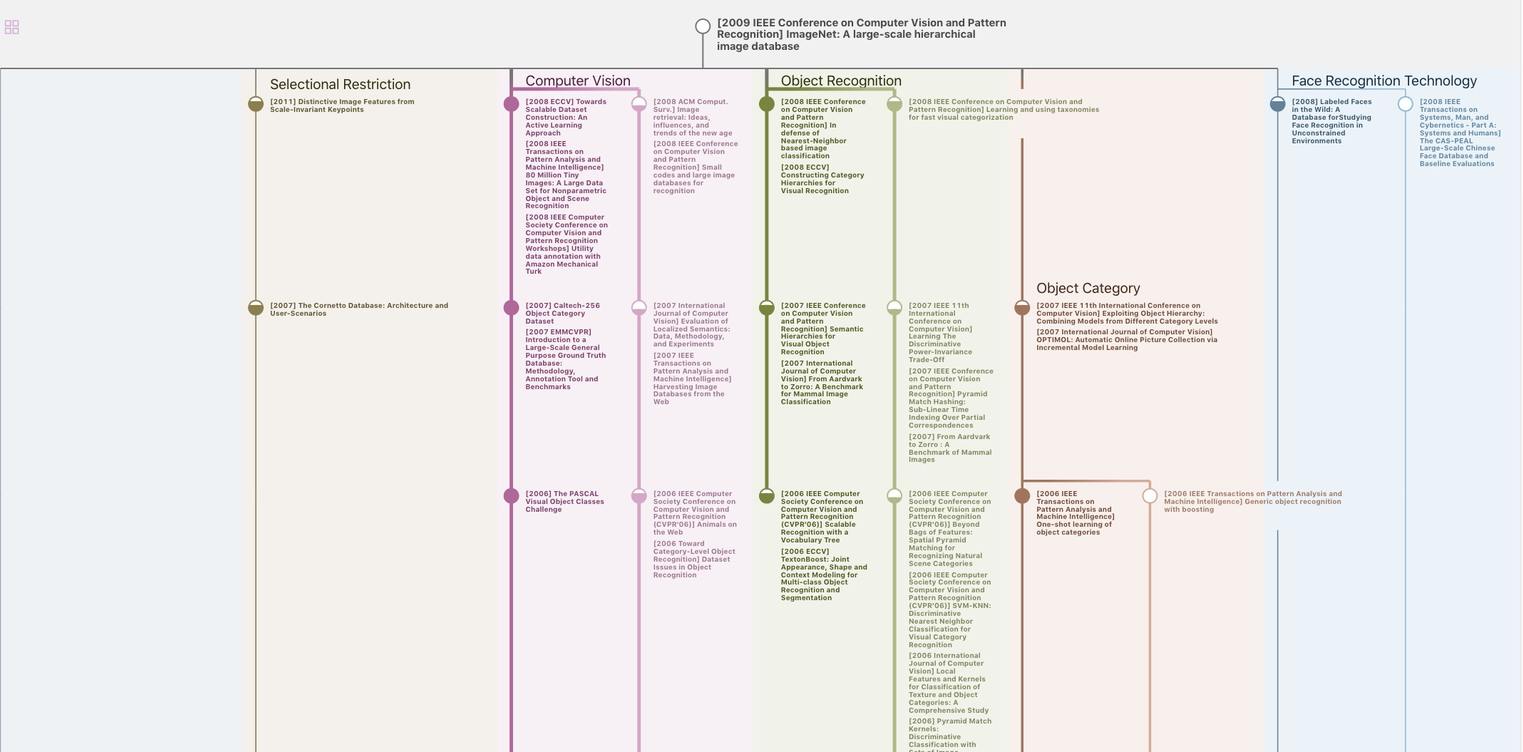
生成溯源树,研究论文发展脉络
Chat Paper
正在生成论文摘要