Unsupervised Detection Of Surface Mine Sites Using Sentinel Multi-Spectral Imagery And Dual-Polarimetric Sar Data
ELEVENTH INDIAN CONFERENCE ON COMPUTER VISION, GRAPHICS AND IMAGE PROCESSING (ICVGIP 2018)(2018)
摘要
Detecting surface mine activities has been one of the major challenges of remote sensing community to monitor ecological balance in the vicinity of mine area. Towards addressing this issue, we propose an unsupervised mine-site detection technique using multi-modal remotely sensed data from Sentinel missions. We use Sentinel-1's dual polarized SAR data, and Sentinel-2's multi-spectral data for this purpose. An initial set of seed pixels is sampled using spectral slopes based rules on multi-spectral images, which are tailored to capture mining area pixels that exhibit common characteristics. Then, we generate features using multi-spectral image indices and dual polarized SAR data Stokes' parameters. These features over locations sampled by slope based rules are used to train a one-class support vector machine to detect the land anomalies (mine activities). As observed from the experiments, multi-spectral features are efficient in separating mine region and built-up region from the rest of the land surface, and SAR image features discriminate built-up region from the rest of the image. We fuse both of these imagery features to encash their advantage in segregating mine sites in the considered study area. We demonstrate the efficiency of the proposed technique in detecting active coal mines over two study sites, Asansol region (in West bengal, India) and Jharia region (in Jharkhand, India), both of which exhibit common geographical characteristics. The average detection accuracy in our results is found to be around 94.8%.
更多查看译文
关键词
Multi-spectral imagery, synthetic aperture radar, surface mining area detection, multi-modal analysis, spectral slopes
AI 理解论文
溯源树
样例
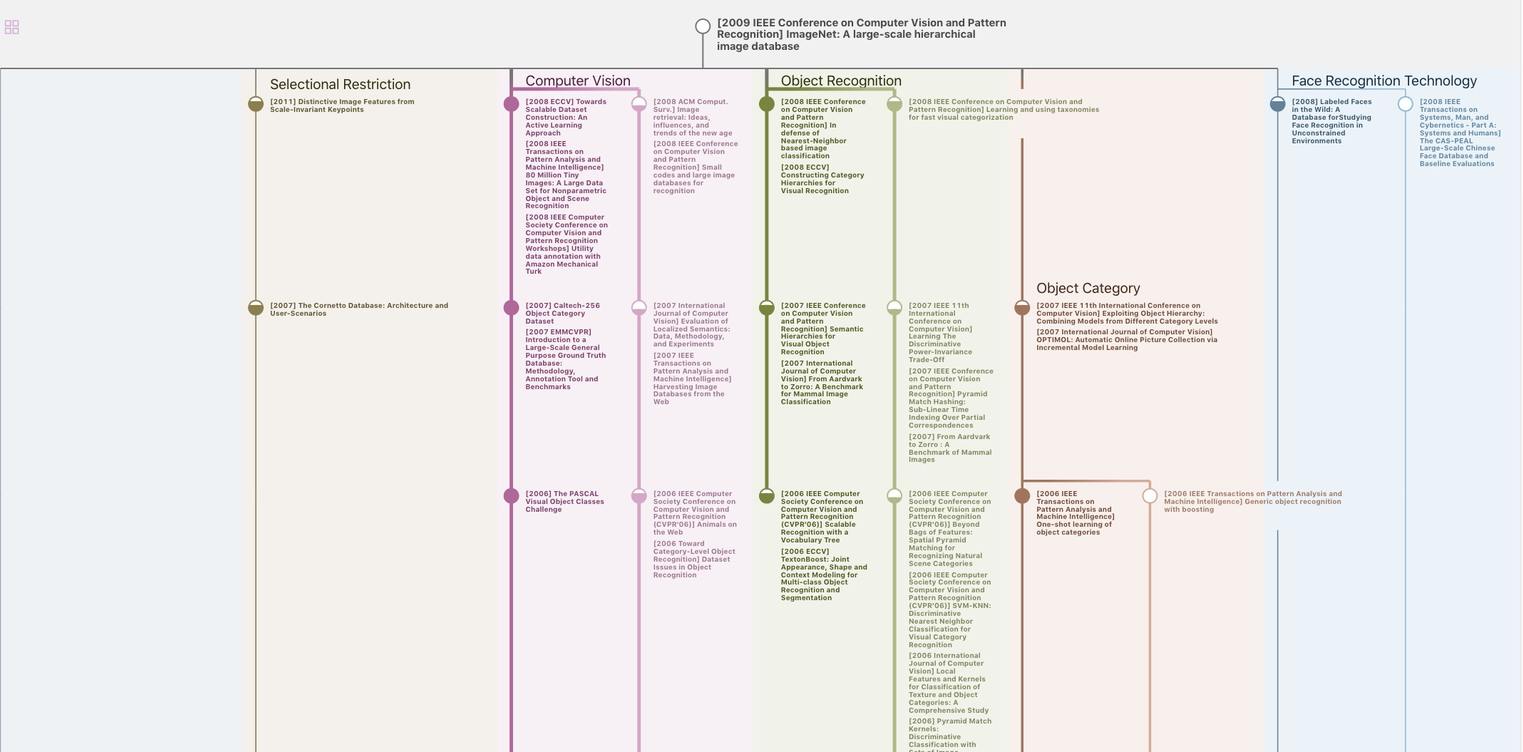
生成溯源树,研究论文发展脉络
Chat Paper
正在生成论文摘要