Toward Adversarial Robustness by Diversity in an Ensemble of Specialized Deep Neural Networks.
Canadian Conference on AI(2020)
摘要
We aim at demonstrating the influence of diversity in the ensemble of CNNs on the detection of black-box adversarial instances and hardening the generation of white-box adversarial attacks. To this end, we propose an ensemble of diverse specialized CNNs along with a simple voting mechanism. The diversity in this ensemble creates a gap between the predictive confidences of adversaries and those of clean samples, making adversaries detectable. We then analyze how diversity in such an ensemble of specialists may mitigate the risk of the black-box and white-box adversarial examples. Using MNIST and CIFAR-10, we empirically verify the ability of our ensemble to detect a large portion of well-known black-box adversarial examples, which leads to a significant reduction in the risk rate of adversaries, at the expense of a small increase in the risk rate of clean samples. Moreover, we show that the success rate of generating white-box attacks by our ensemble is remarkably decreased compared to a vanilla CNN and an ensemble of vanilla CNNs, highlighting the beneficial role of diversity in the ensemble for developing more robust models.
更多查看译文
关键词
adversarial robustness,diversity,ensemble,neural networks
AI 理解论文
溯源树
样例
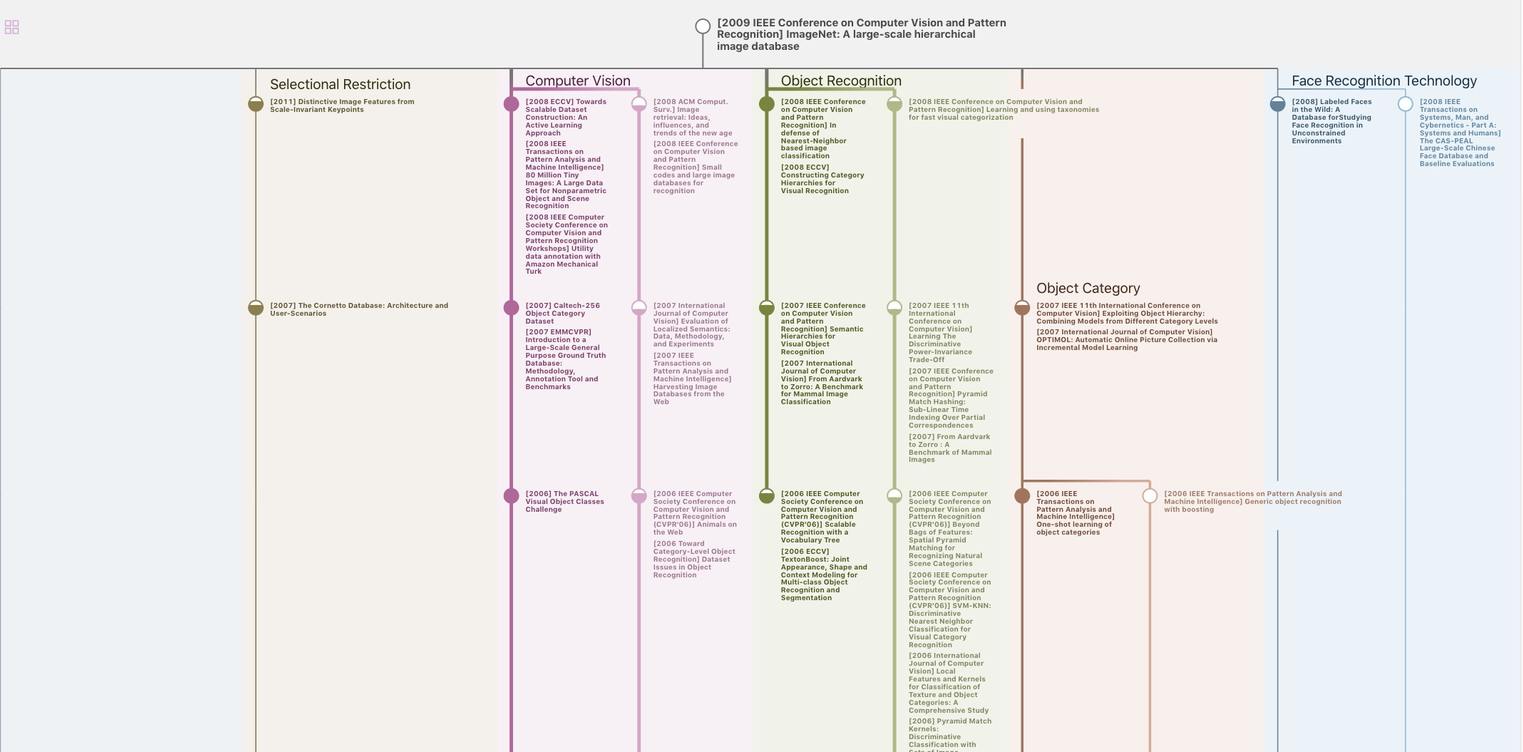
生成溯源树,研究论文发展脉络
Chat Paper
正在生成论文摘要