Ensuring Fairness under Prior Probability Shifts
AIES '21: PROCEEDINGS OF THE 2021 AAAI/ACM CONFERENCE ON AI, ETHICS, AND SOCIETY(2021)
摘要
Prior probability shift is a phenomenon where the training and test datasets differ structurally within population subgroups. This phenomenon can be observed in the yearly records of several real-world datasets, for example, recidivism records and medical expenditure surveys. If unaccounted for, such shifts can cause the predictions of a classifier to become unfair towards specific population subgroups. While the fairness notion called Proportional Equality (PE) accounts for such shifts, a procedure to ensure PE-fairness was unknown. In this work, we design an algorithm, called CAPE, that ensures fair classification under such shifts. We introduce a metric, called prevalence difference (PD), which CAPE attempts to minimize in order to achieve fairness under prior probability shifts. We theoretically establish that this metric exhibits several properties that are desirable for a fair classifier. We evaluate the efficacy of CAPE via a thorough empirical evaluation on synthetic datasets. We also compare the performance of CAPE with several state-of-the-art fair classifiers on real-world datasets like COMPAS (criminal risk assessment) and MEPS (medical expenditure panel survey). The results indicate that CAPE ensures a high degree of PE-fairness in its predictions, while performing well on other important metrics.
更多查看译文
关键词
Classification, Discrimination, Distributional shifts, Algorithmic Fairness
AI 理解论文
溯源树
样例
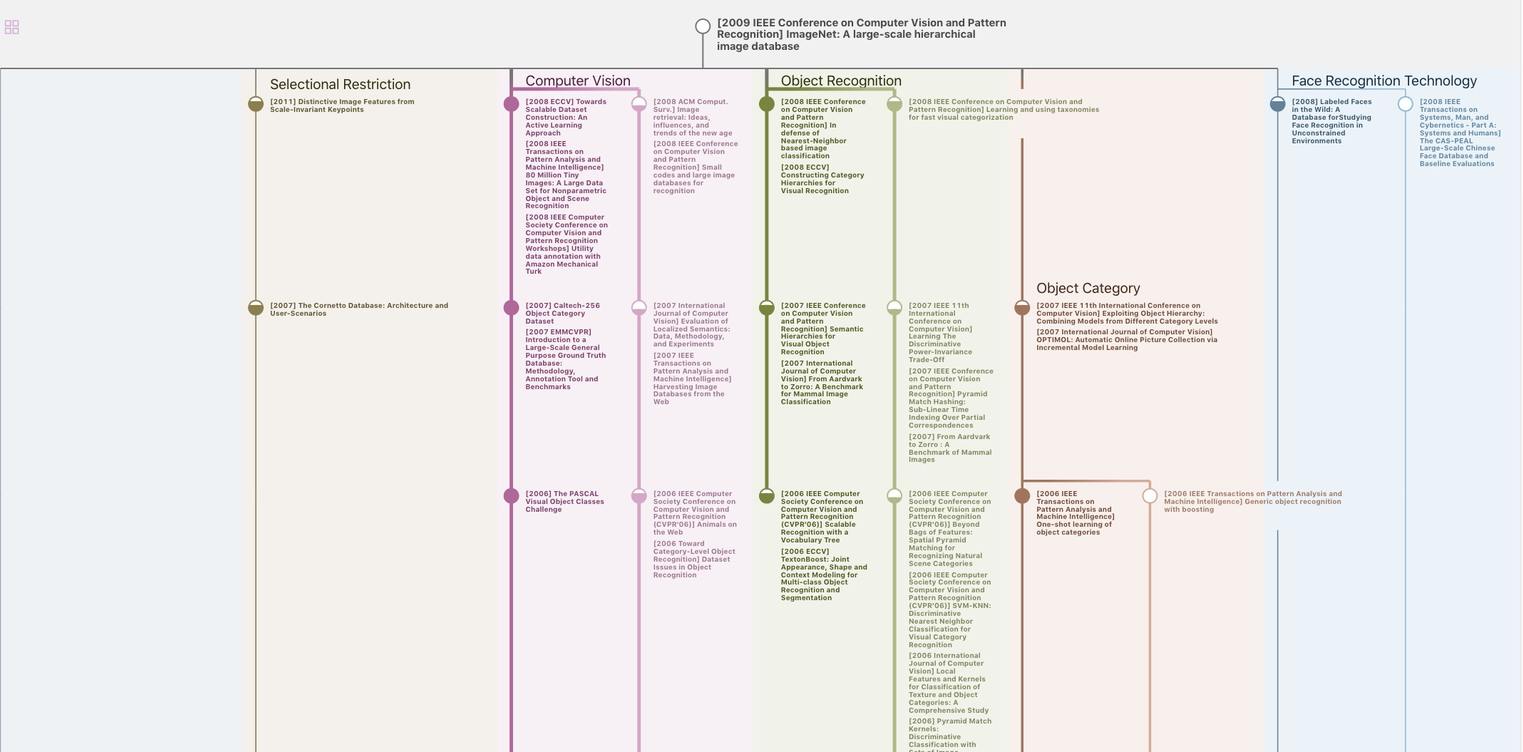
生成溯源树,研究论文发展脉络
Chat Paper
正在生成论文摘要