Fast Mapping onto Census Blocks
2020 IEEE High Performance Extreme Computing Conference (HPEC)(2020)
摘要
Pandemic measures such as social distancing and contact tracing can be enhanced by rapidly integrating dynamic location data and demographic data. Projecting billions of longitude and latitude locations onto hundreds of thousands of highly irregular demographic census block polygons is computationally challenging in both research and deployment contexts. This paper describes two approaches labeled "simple" and "fast". The simple approach can be implemented in any scripting language (Matlab/Octave, Python, Julia, R) and is easily integrated and customized to a variety of research goals. This simple approach uses a novel combination of hierarchy, sparse bounding boxes, polygon crossing-number, vectorization, and parallel processing to achieve 100,000,000+ projections per second on 100 servers. The simple approach is compact, does not increase data storage requirements, and is applicable to any country or region. The fast approach exploits the thread, vector, and memory optimizations that are possible using a low-level language (C++) and achieves similar performance on a single server. This paper details these approaches with the goal of enabling the broader community to quickly integrate location and demographic data.
更多查看译文
关键词
dynamic location data,demographic data,projecting billions,longitude latitude locations,highly irregular demographic census block polygons,deployment contexts,research goals,polygon crossing-number,data storage requirements,fast mapping,census blocks,pandemic measures,social distancing
AI 理解论文
溯源树
样例
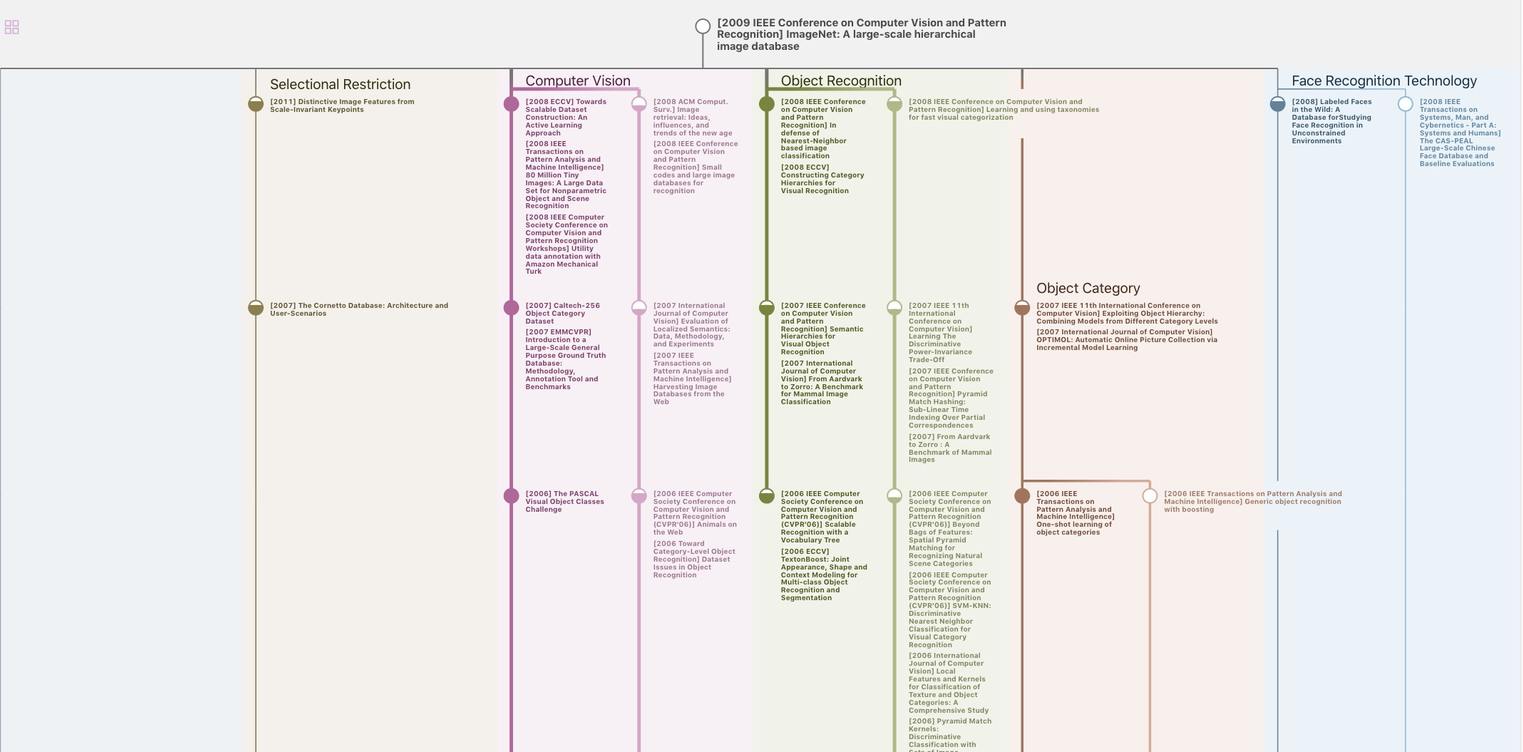
生成溯源树,研究论文发展脉络
Chat Paper
正在生成论文摘要