CODA-19: Reliably Annotating Research Aspects on 10,000+ CORD-19 Abstracts Using Non-Expert Crowd
arxiv(2020)
摘要
This paper introduces CODA-19, a human-annotated dataset that denotes the Background, Purpose, Method, Finding/Contribution, and Other for 10,966 English abstracts in the COVID-19 Open Research Dataset. This dataset was created by 248 crowd workers from Amazon Mechanical Turk collectively within ten days, achieving a label quality comparable to experts. Each abstract was annotated by nine different workers and the final labels were obtained by majority voting. The inter-annotator agreement (Cohen's kappa) between the crowd and the biomedical expert (0.741) is comparable to inter-expert agreement (0.788). CODA-19's labels have an accuracy of 82.2% when compared against the biomedical expert's labels, while the accuracy between experts was 85.0%. Reliable human annotations help scientists to understand the rapidly accelerating coronavirus literature and also serves as the battery of AI/NLP research. While obtaining expert annotations can be slow, CODA-19 demonstrated that non-expert crowd can be employed at scale rapidly to join the combat against COVID-19.
更多查看译文
AI 理解论文
溯源树
样例
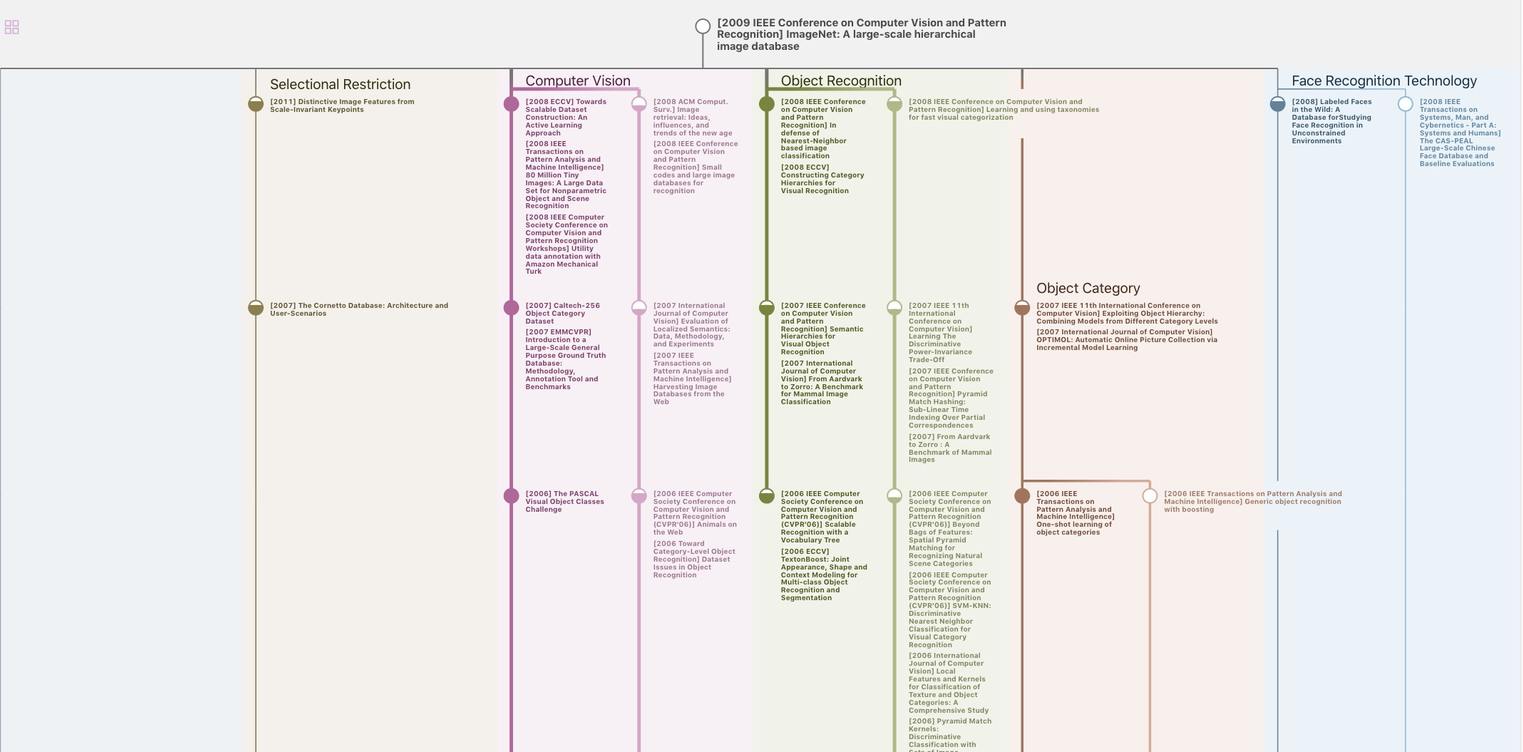
生成溯源树,研究论文发展脉络
Chat Paper
正在生成论文摘要