An improved feature extractor for the Lidar Odometry and Mapping (LOAM) algorithm
International Conference on Control Automation and Information Sciences(2019)
摘要
This work proposes an improved feature extractor for the Lidar Odometry and Mapping (LOAM) algorithm, which is currently the highest ranked algorithm in the Karlsruhe Institute of Technology and Toyota Technological Institute (KITTI) visual odometry ranking. This article proposes and justifies the substitution of LOAM's current feature extraction method with the Curvature Scale Space (CSS) based feature extraction algorithm for the processing of 3D Point Cloud Data (PCD). The justification is based on in improvement of the repeatability of the detection of robust features for LOAM and an improvement in the associated computational cost. The LOAM's feature extractor and CSS feature extractor were tested and compared with simulated and real data including the KITTI visual odometry dataset using the Optimal Sub-Pattern Assignment (OSPA) and Absolute Trajectory Error (ATE) metrics. The results showed that LOAM based on the CSS feature extractor out performed that based on the original LOAM feature extractor with respect to these metrics.
更多查看译文
关键词
3D Point Cloud Data,robust features,CSS feature extractor,KITTI visual odometry dataset,LOAM feature extractor,curvature scale space based feature extraction algorithm,lidar odometry and mapping algorithm,optimal subpattern assignment,OSPA,absolute trajectory error metrics,ATE metrics,Karlsruhe Institute of Technology and Toyota Technological Institute,KITTI visual odometry ranking
AI 理解论文
溯源树
样例
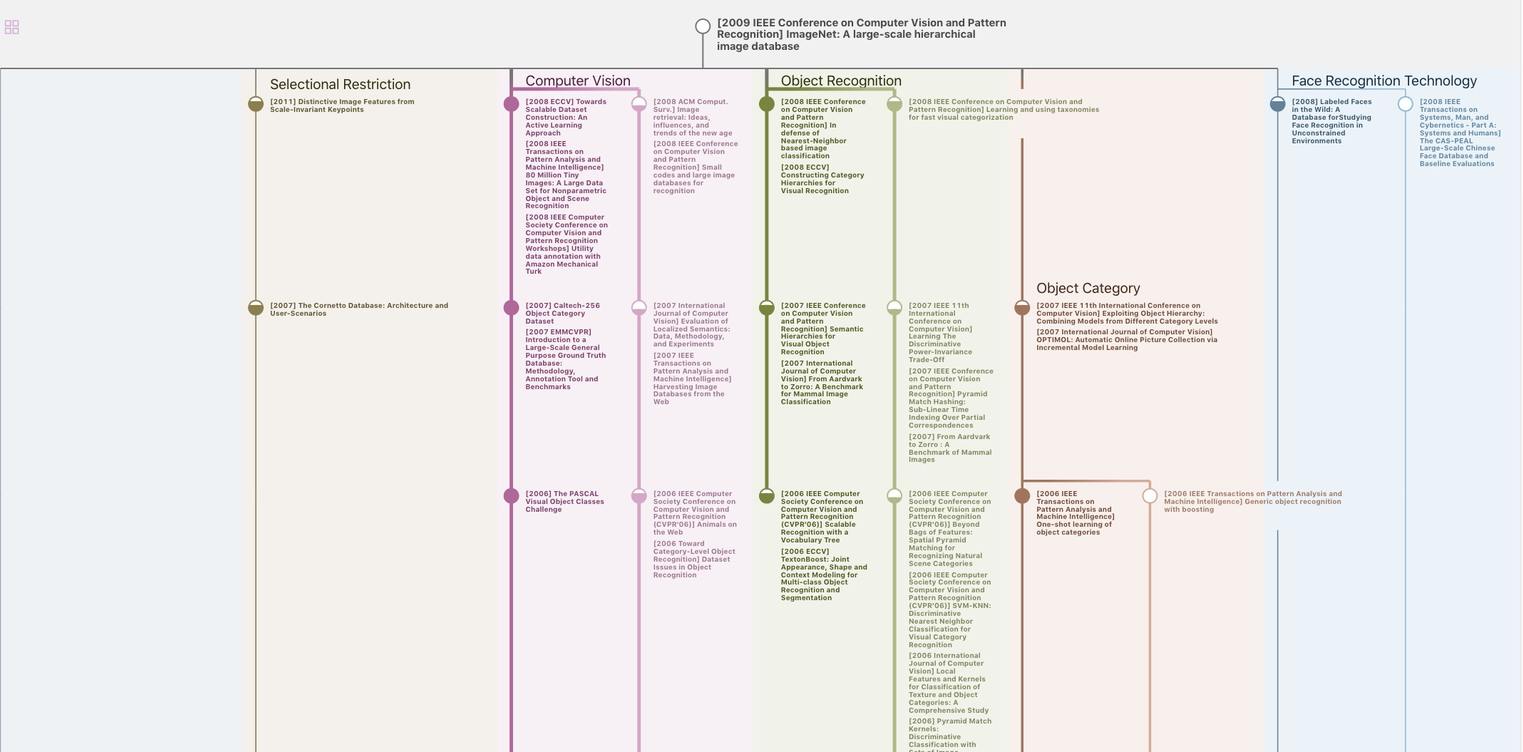
生成溯源树,研究论文发展脉络
Chat Paper
正在生成论文摘要