Artemis: A Novel Annotation Methodology for Indicative Single Document Summarization
Proceedings of the First Workshop on Evaluation and Comparison of NLP Systems(2020)
摘要
We describe Artemis (Annotation methodology for Rich, Tractable, Extractive, Multi-domain, Indicative Summarization), a novel hierarchical annotation process that produces indicative summaries for documents from multiple domains. Current summarization evaluation datasets are single-domain and focused on a few domains for which naturally occurring summaries can be easily found, such as news and scientific articles. These are not sufficient for training and evaluation of summarization models for use in document management and information retrieval systems, which need to deal with documents from multiple domains. Compared to other annotation methods such as Relative Utility and Pyramid, Artemis is more tractable because judges don’t need to look at all the sentences in a document when making an importance judgment for one of the sentences, while providing similarly rich sentence importance annotations. We describe the annotation process in detail and compare it with other similar evaluation systems. We also present analysis and experimental results over a sample set of 532 annotated documents.
更多查看译文
关键词
novel annotation methodology,indicative single document
AI 理解论文
溯源树
样例
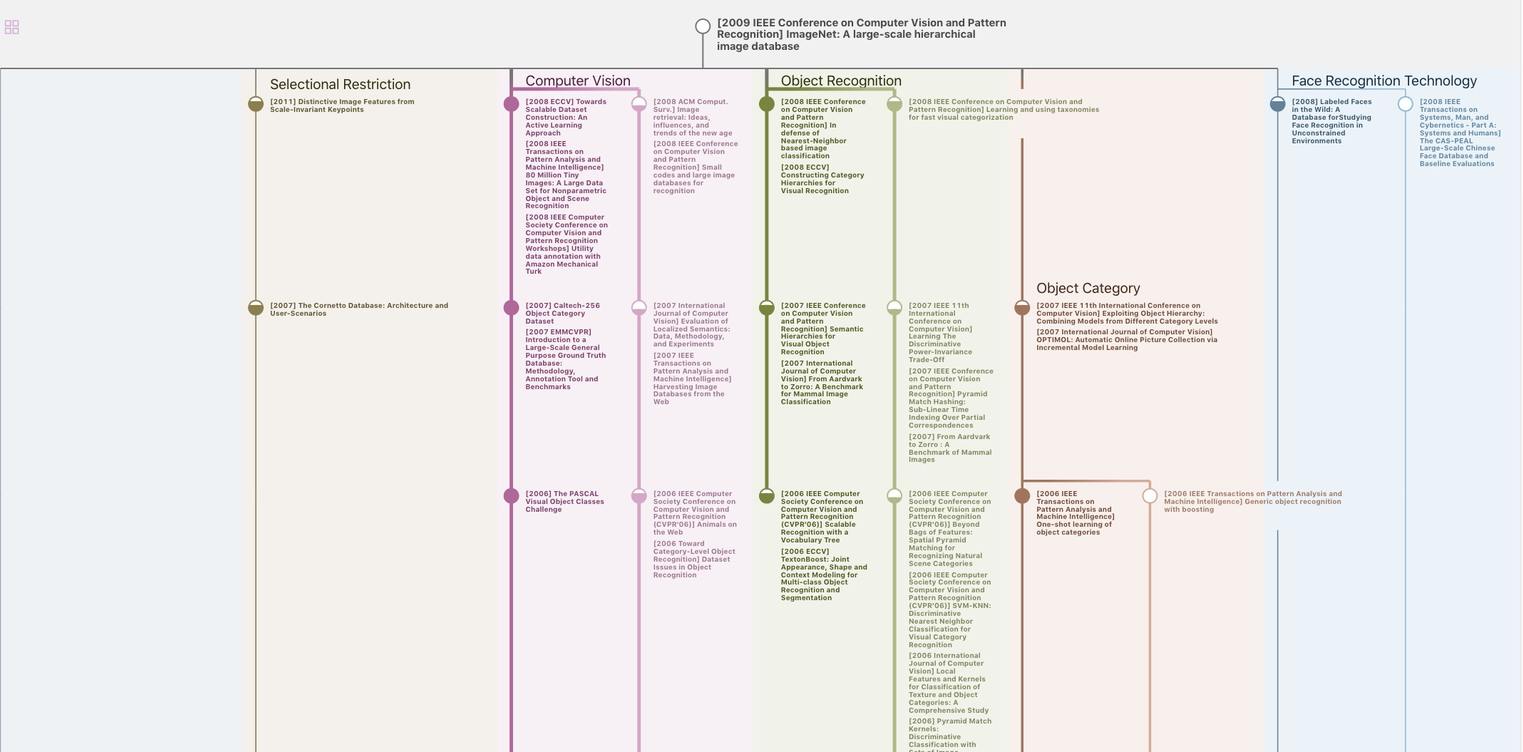
生成溯源树,研究论文发展脉络
Chat Paper
正在生成论文摘要