Identifying User Communities Using Deep Learning and Its Application to Opportunistic Networking
2019 IEEE 16th International Conference on Mobile Ad Hoc and Sensor Systems (MASS)(2019)
摘要
Opportunistic networking has been proposed to address episodic connectivity, common in so-called "challenged" or "extreme" networking environments where arbitrarily frequent and long-lived connectivity disruptions are the norm, instead of the exception. Examples of extreme networking environments and applications include interplanetary communication, disaster relief, and emergency response, (semi-)autonomous driving, to name a few. This paper proposes a novel community-based opportunistic routing approach that identifies user communities based on mobility features extracted from real traces of user mobility. The proposed Deep AutoenCoder Community-based Opportunistic Routing protocol, or DACCOR, employs deep learning to identify user communities based on data extracted from user mobility traces and uses user community information to make forwarding decisions in opportunistic networking scenarios. Through extensive simulations, we evaluate DACCOR's performance and show that it outperforms well-known opportunistic forwarding protocols in terms of delivery probability, latency, and communication overhead. We also show that DACCOR's lower communication overhead yields considerable energy efficiency, increasing mobile devices' battery lifetime.
更多查看译文
关键词
Opportunistic routing protocols,Machine learning,Community identification
AI 理解论文
溯源树
样例
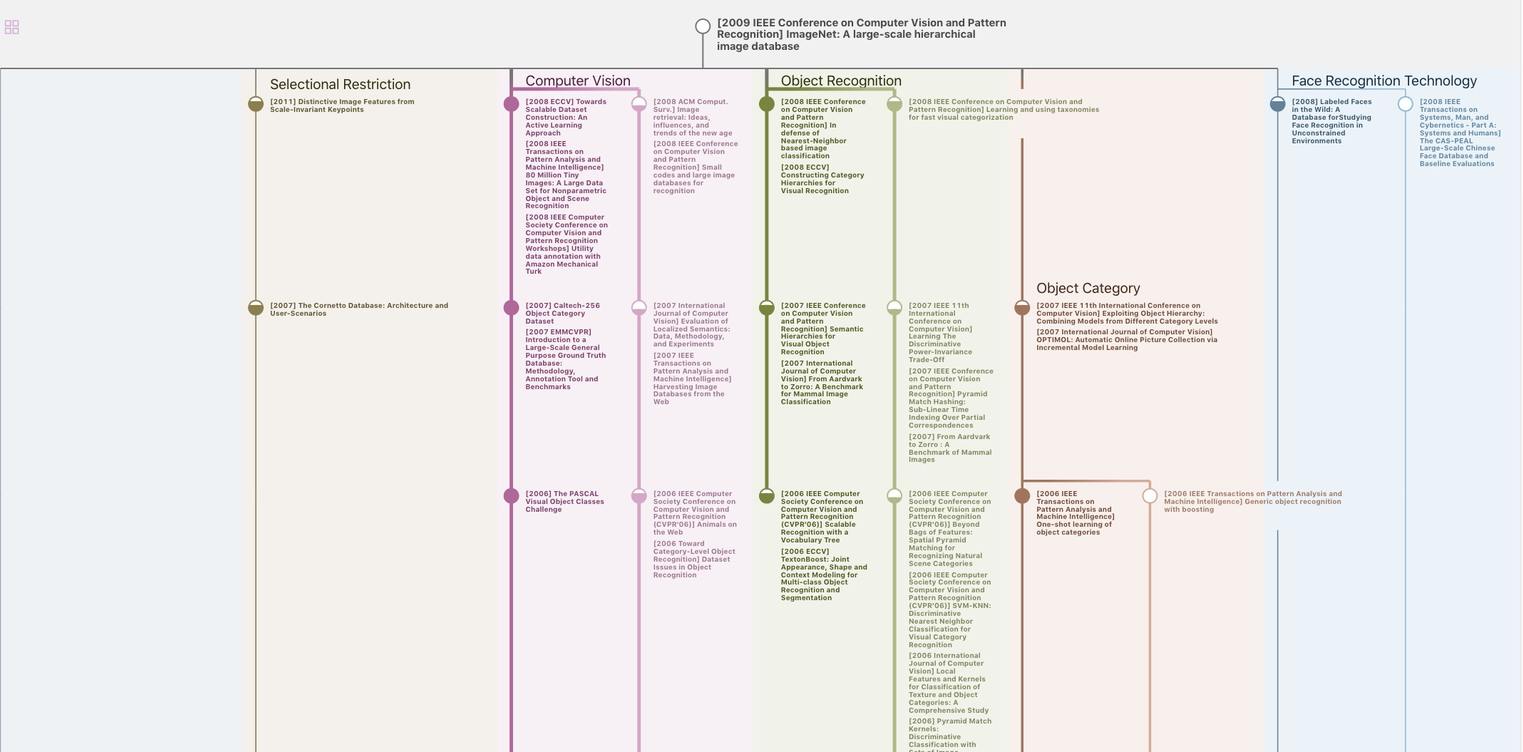
生成溯源树,研究论文发展脉络
Chat Paper
正在生成论文摘要