Partially-Typed NER Datasets Integration: Connecting Practice to Theory
arxiv(2020)
摘要
While typical named entity recognition (NER) models require the training set to be annotated with all target types, each available datasets may only cover a part of them. Instead of relying on fully-typed NER datasets, many efforts have been made to leverage multiple partially-typed ones for training and allow the resulting model to cover a full type set. However, there is neither guarantee on the quality of integrated datasets, nor guidance on the design of training algorithms. Here, we conduct a systematic analysis and comparison between partially-typed NER datasets and fully-typed ones, in both theoretical and empirical manner. Firstly, we derive a bound to establish that models trained with partially-typed annotations can reach a similar performance with the ones trained with fully-typed annotations, which also provides guidance on the algorithm design. Moreover, we conduct controlled experiments, which shows partially-typed datasets leads to similar performance with the model trained with the same amount of fully-typed annotations
更多查看译文
关键词
partially-typed
AI 理解论文
溯源树
样例
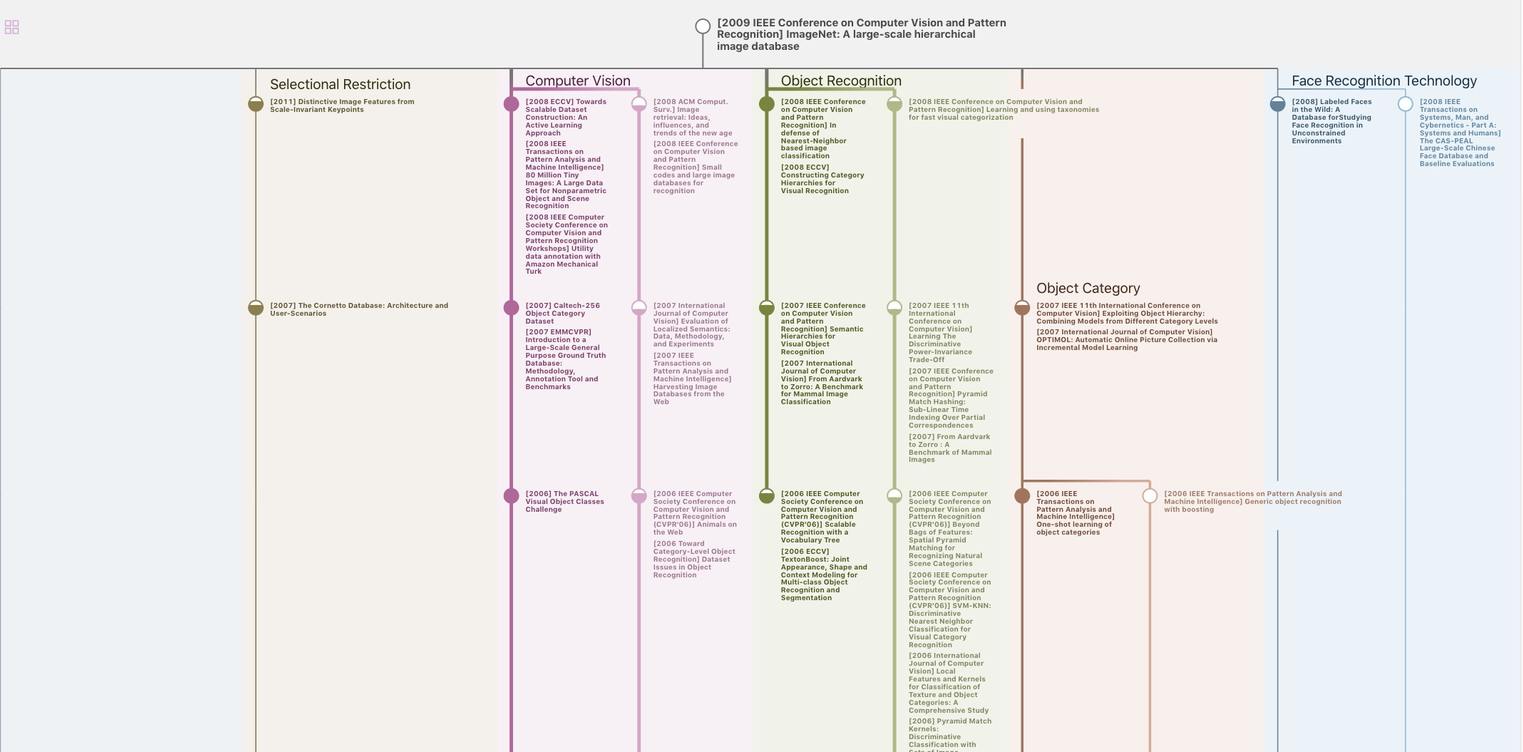
生成溯源树,研究论文发展脉络
Chat Paper
正在生成论文摘要