Thresholded Adaptive Validation: Tuning the Graphical Lasso for Graph Recovery
arXiv (Cornell University)(2020)
摘要
The graphical lasso is the most popular estimator in Gaussian graphical models, but its performance hinges on a regularization parameter that needs to be calibrated to each application at hand. In this paper, we propose a novel calibration scheme for this parameter. The scheme is equipped with theoretical guarantees and motivates a thresholding pipeline that can improve graph recovery. Moreover, requiring at most one line search over the regularization path of the graphical lasso, the calibration scheme is computationally more efficient than competing schemes that are based on resampling. Finally, we show in simulations that our approach can improve on the graph recovery of other approaches considerably.
更多查看译文
关键词
graphical recovery,graphical lasso,adaptive validation
AI 理解论文
溯源树
样例
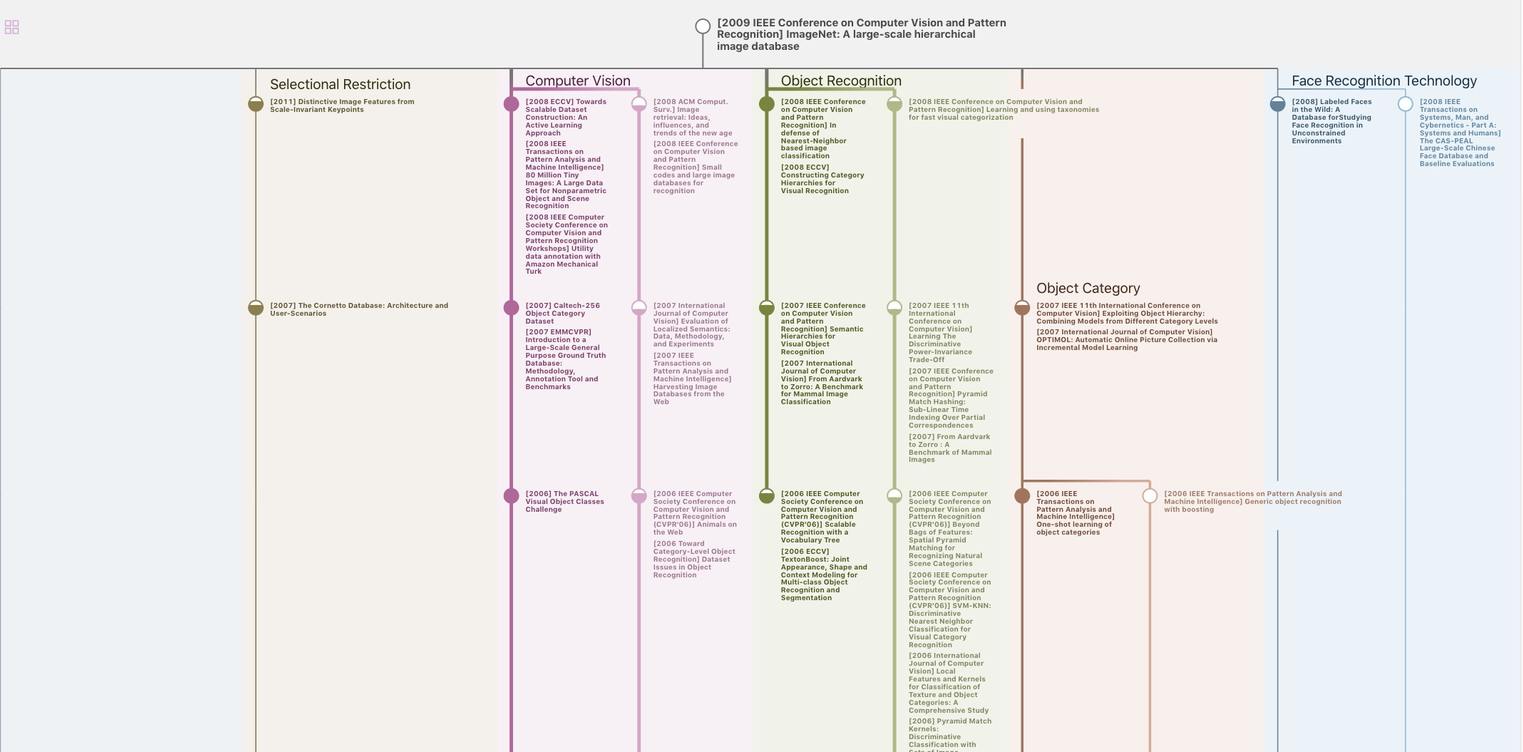
生成溯源树,研究论文发展脉络
Chat Paper
正在生成论文摘要