State Aggregations In Markov Chains And Block Models Of Networks
PHYSICAL REVIEW LETTERS(2021)
摘要
We consider state-aggregation schemes for Markov chains from an information-theoretic perspective. Specifically, we consider aggregating the states of a Markov chain such that the mutual information of the aggregated states separated by T time steps is maximized. We show that for T = 1 this recovers the maximum-likelihood estimator of the degree-corrected stochastic block model as a particular case, which enables us to explain certain features of the likelihood landscape of this generative network model from a dynamical lens. We further highlight how we can uncover coherent, long-range dynamical modules for which considering a timescale T >> 1 is essential. We demonstrate our results using synthetic flows and real-world ocean currents, where we are able to recover the fundamental features of the surface currents of the oceans.
更多查看译文
AI 理解论文
溯源树
样例
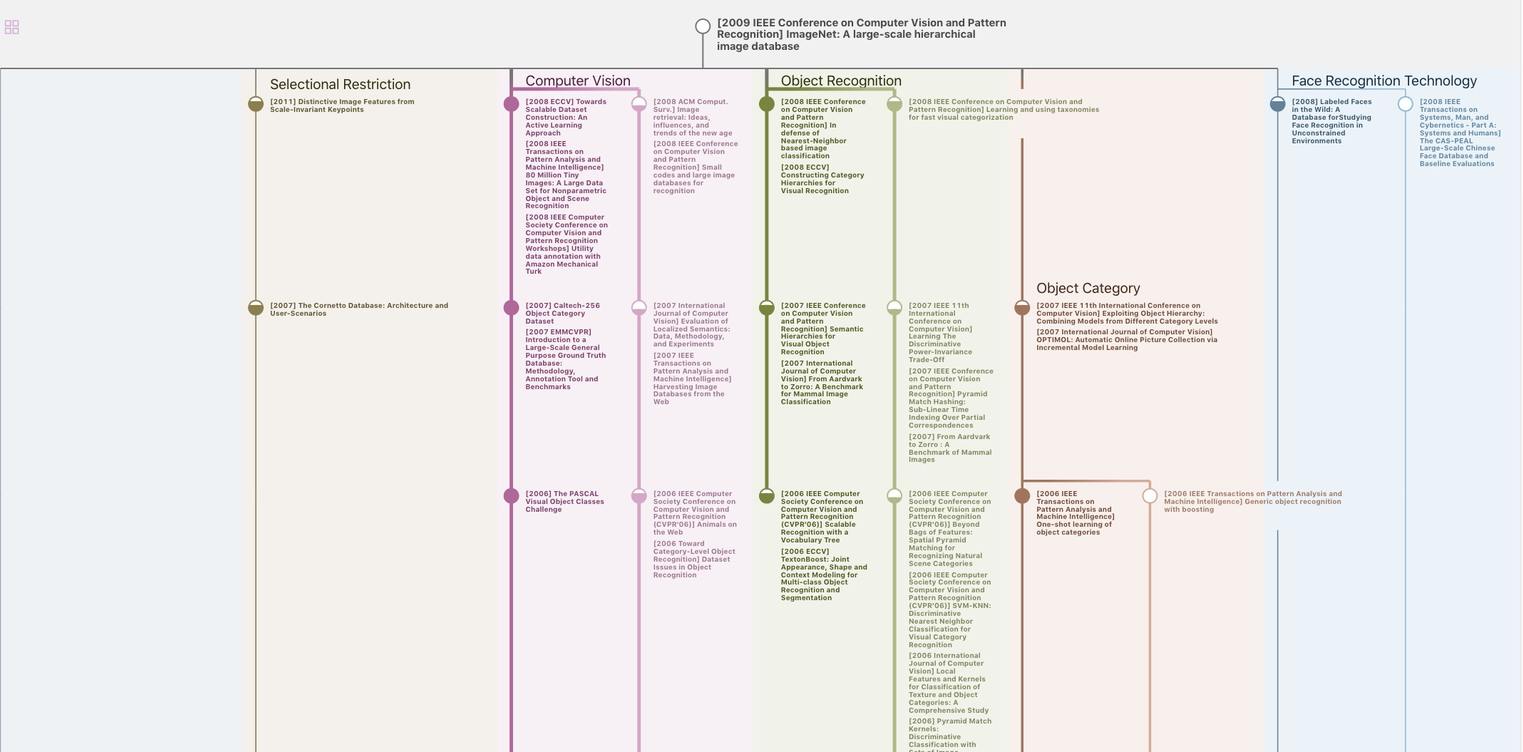
生成溯源树,研究论文发展脉络
Chat Paper
正在生成论文摘要