Supervised Feature Subset Selection and Feature Ranking for Multivariate Time Series without Feature Extraction
arxiv(2020)
摘要
We introduce supervised feature ranking and feature subset selection algorithms for multivariate time series (MTS) classification. Unlike most existing supervised/unsupervised feature selection algorithms for MTS our techniques do not require a feature extraction step to generate a one-dimensional feature vector from the time series. Instead it is based on directly computing similarity between individual time series and assessing how well the resulting cluster structure matches the labels. The techniques are amenable to heterogeneous MTS data, where the time series measurements may have different sampling resolutions, and to multi-modal data.
更多查看译文
关键词
feature subset selection,multivariate time series,feature ranking,feature extraction,time series
AI 理解论文
溯源树
样例
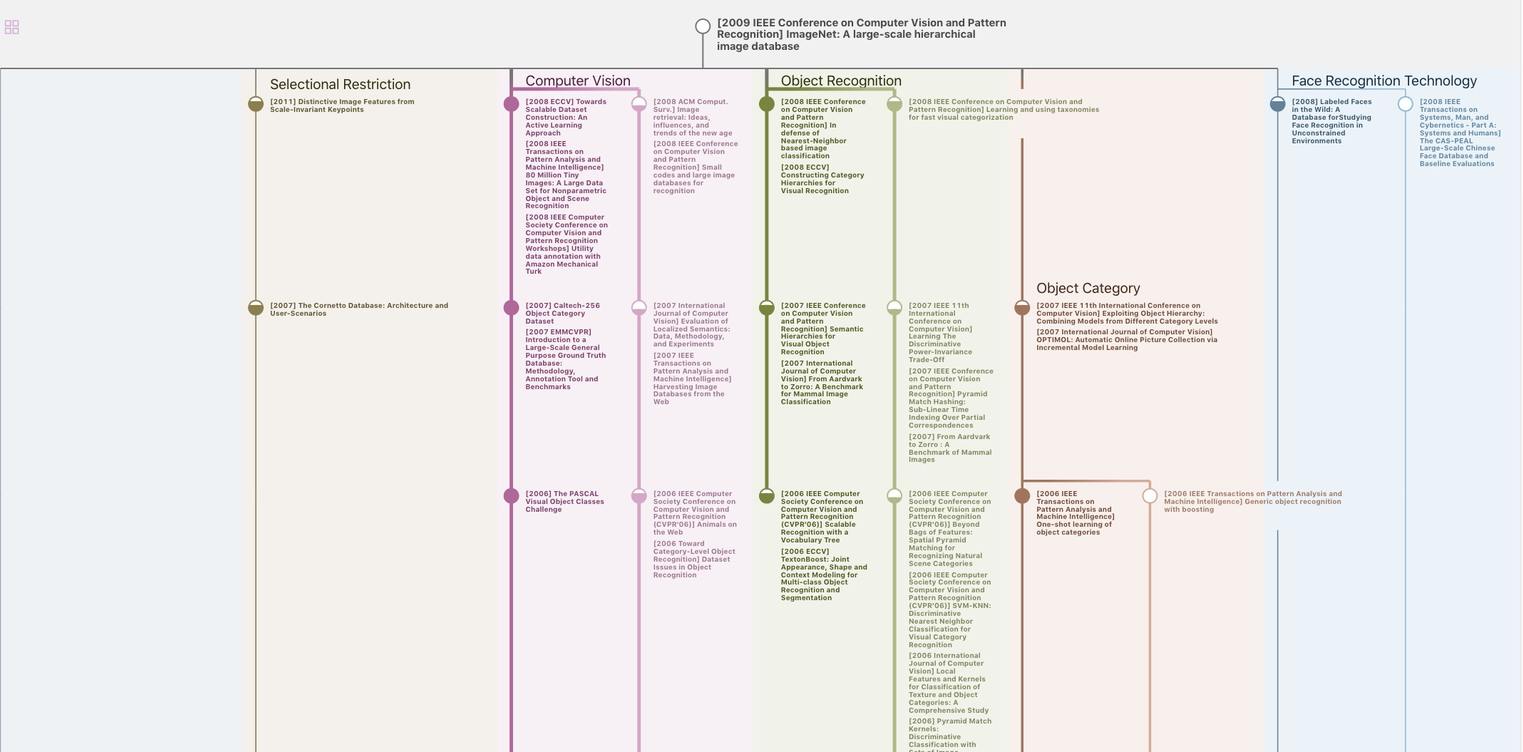
生成溯源树,研究论文发展脉络
Chat Paper
正在生成论文摘要