Adversary-Resilient Distributed and Decentralized Statistical Inference and Machine Learning: An Overview of Recent Advances Under the Byzantine Threat Model
IEEE Signal Processing Magazine(2020)
摘要
Statistical inference and machine-learning algorithms have traditionally been developed for data available at a single location. Unlike this centralized setting, modern data sets are increasingly being distributed across multiple physical entities (sensors, devices, machines, data centers, and so on) for a multitude of reasons that range from storage, memory, and computational constraints to privacy concerns and engineering needs. This has necessitated the development of inference and learning algorithms capable of operating on noncolocated data. For this article, we divide such algorithms into two broad categories, namely, distributed algorithms and decentralized algorithms (see "Is It Distributed or Is It Decentralized?").
更多查看译文
关键词
Signal processing algorithms,Servers,Inference algorithms,Distributed databases,Machine learning,Estimation,Sensors
AI 理解论文
溯源树
样例
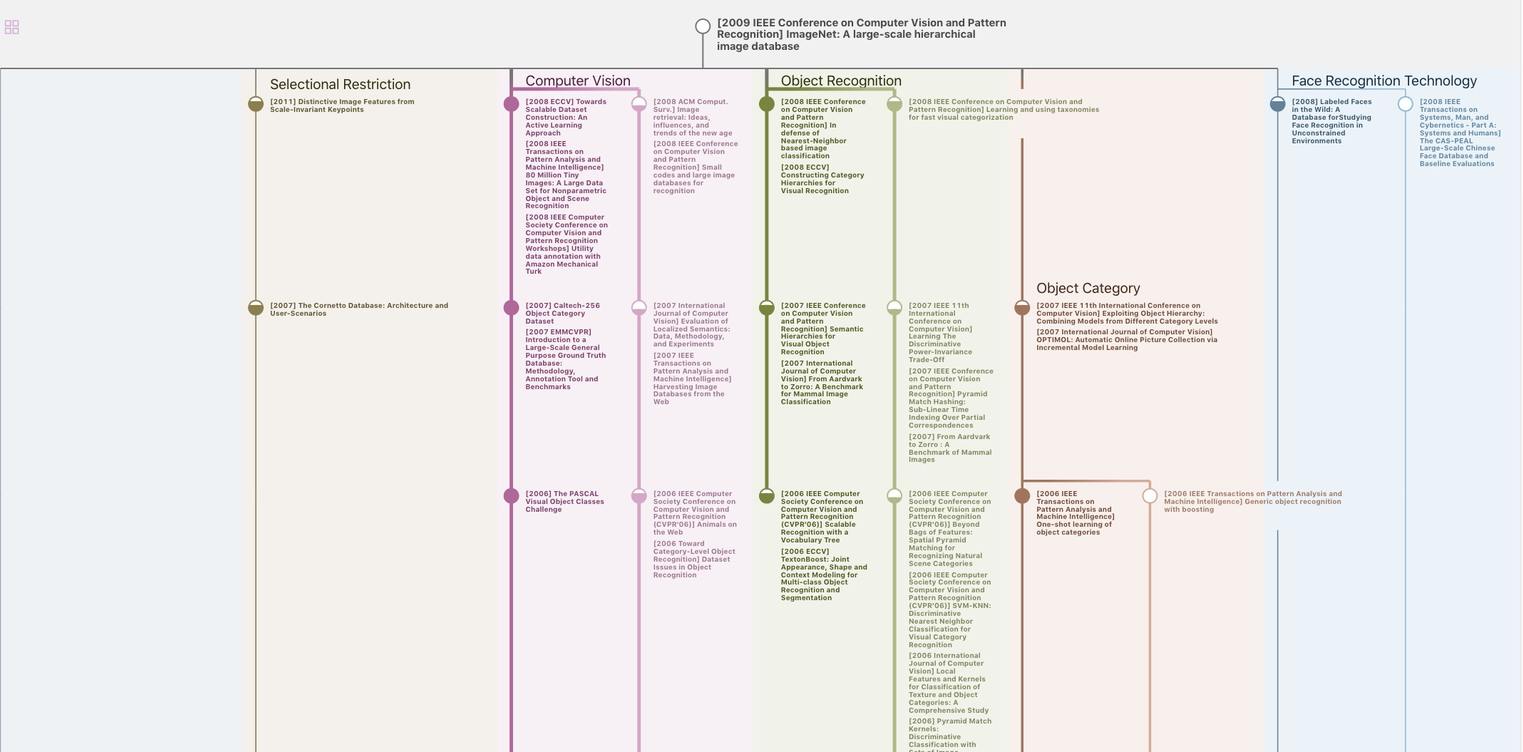
生成溯源树,研究论文发展脉络
Chat Paper
正在生成论文摘要