Asymptotic Network Independence in Distributed Stochastic Optimization for Machine Learning: Examining Distributed and Centralized Stochastic Gradient Descent
IEEE Signal Processing Magazine(2020)
摘要
We provide a discussion of several recent results which, in certain scenarios, are able to overcome a barrier in distributed stochastic optimization for machine learning (ML). Our focus is the so-called asymptotic network independence property, which is achieved whenever a distributed method executed over a network of n nodes asymptotically converges to the optimal solution at a comparable rate to a centralized method with the same computational power as the entire network. We explain this property through an example involving the training of ML models and sketch a short mathematical analysis for comparing the performance of distributed stochastic gradient descent (DSGD) with centralized SGD.
更多查看译文
关键词
Optimization,Signal processing algorithms,Convergence,Stochastic processes,Peer-to-peer computing,Machine learning,Computational modeling
AI 理解论文
溯源树
样例
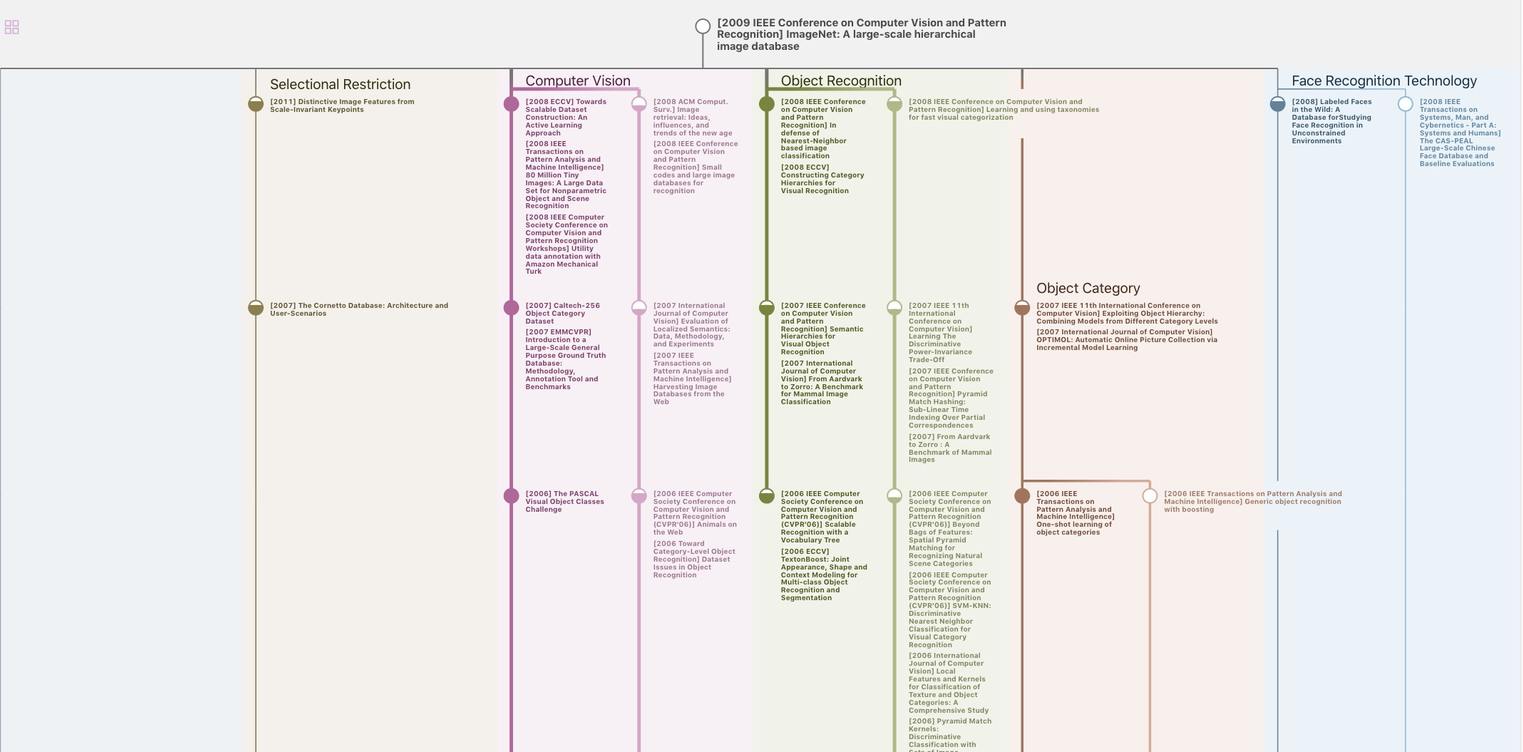
生成溯源树,研究论文发展脉络
Chat Paper
正在生成论文摘要