Video Captioning With Object-Aware Spatio-Temporal Correlation And Aggregation
IEEE TRANSACTIONS ON IMAGE PROCESSING(2020)
摘要
Video captioning is a significant challenging task in computer vision and natural language processing, aiming to automatically describe video content by natural language sentences. Comprehensive understanding of video is the key for accurate video captioning, which needs to not only capture the global content and salient objects in video, but also understand the spatio-temporal relations of objects, including their temporal trajectories and spatial relationships. Thus, it is important for video captioning to capture the objects' relationships both within and across frames. Therefore, in this paper, we propose an object-aware spatio-temporal graph (OSTG) approach for video captioning. It constructs spatio-temporal graphs to depict objects with their relations, where the temporal graphs represent objects' inter-frame dynamics, and the spatial graphs represent objects' intra-frame interactive relationships. The main novelties and advantages are: (1) Bidirectional temporal alignment: Bidirectional temporal graph is constructed along and reversely along the temporal order to perform bidirectional temporal alignment for objects across different frames, which provides complementary clues to capture the inter-frame temporal trajectories for each salient object. (2) Graph based spatial relation learning: Spatial relation graph is constructed among objects in each frame by considering their relative spatial locations and semantic correlations, which is exploited to learn relation features that encode intra-frame relationships for salient objects. (3) Object-aware feature aggregation: Trainable VLAD (vector of locally aggregated descriptors) models are deployed to perform object-aware feature aggregation on objects' local features, which learn discriminative aggregated representations for better video captioning. A hierarchical attention mechanism is also developed to distinguish contributions of different object instances. Experiments on two widely-used datasets, MSR-VTT and MSVD, demonstrate our proposed approach achieves state-of-the-art performances in terms of BLEU@4, METEOR and CIDEr metrics.
更多查看译文
关键词
Video captioning, spatio-temporal graph, bidirectional temporal graph, spatial relation graph, object-aware feature aggregation
AI 理解论文
溯源树
样例
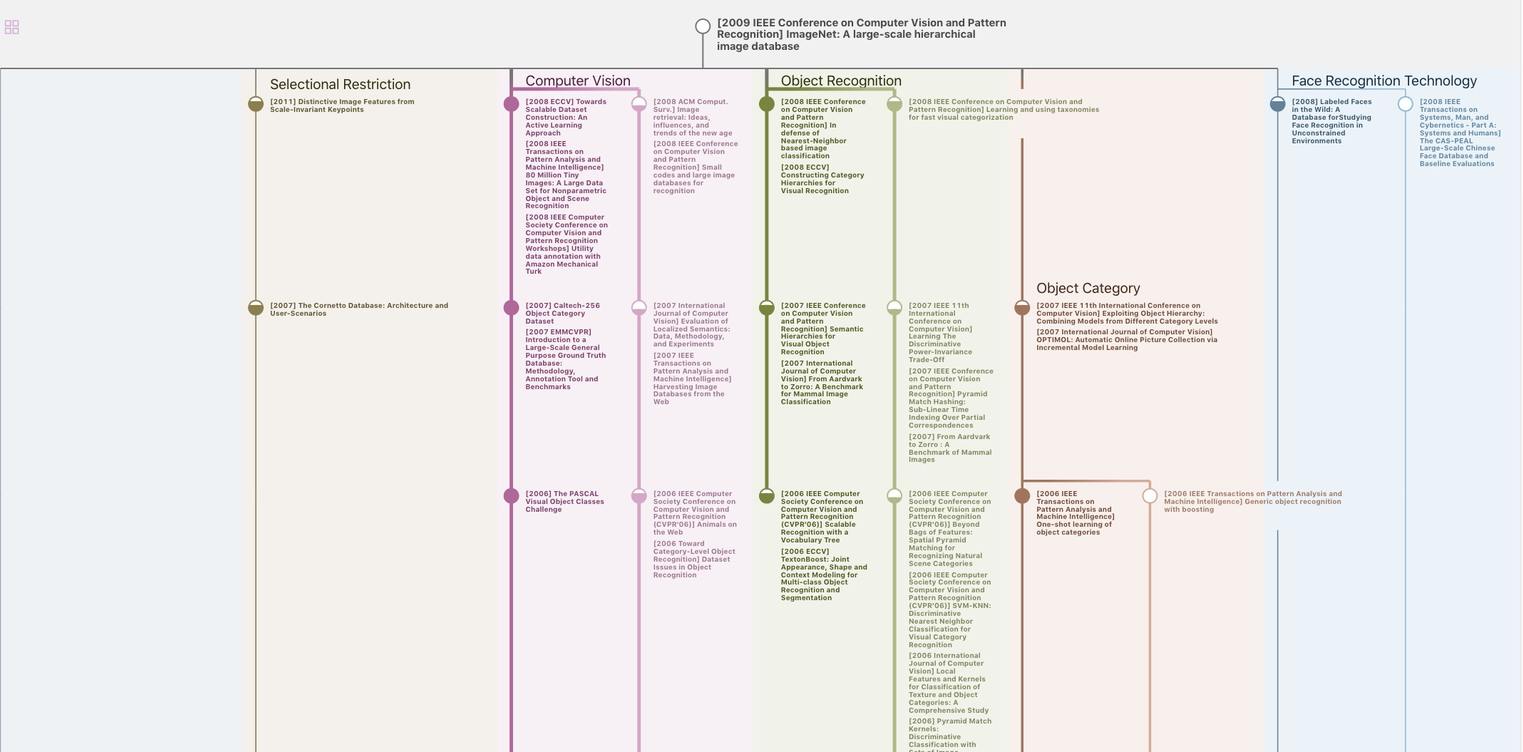
生成溯源树,研究论文发展脉络
Chat Paper
正在生成论文摘要