Evaluating Mutual Information and Chi-Square Metrics in Text Features Selection Process - A Study Case Applied to the Text Classification in PubMed.
IWBBIO(2020)
摘要
The aim of this work was to compare the behavior of mutual information and Chi-square as metrics in the evaluation of the relevance of the terms extracted from documents related to "software design" retrieved from PubMed database tested in two contexts: using a set of terms retrieved from the vectorization of the corpus of abstracts and using only the terms retrieved from the vocabulary defined by the IEEE standard ISO/IEC/IEEE 24765. A search was conducted concerning the subject "software" in the last 6 years and we used Medical Subject Headings (Mesh) term "software design" of the articles to label them. Then mutual information and Chi-square metrics were computed as metrics to sort and select features. Chi-square obtained the highest accuracy scores in documents classification by using a multinomial naive Bayes classifier. Although these results suggest that Chi-square is better than mutual information in feature relevance estimation in the context of this work, further research is necessary to obtain a consistent foundation of this conclusion.
更多查看译文
关键词
Software design, Features selection, Natural language processing, Chi-square, Mutual information
AI 理解论文
溯源树
样例
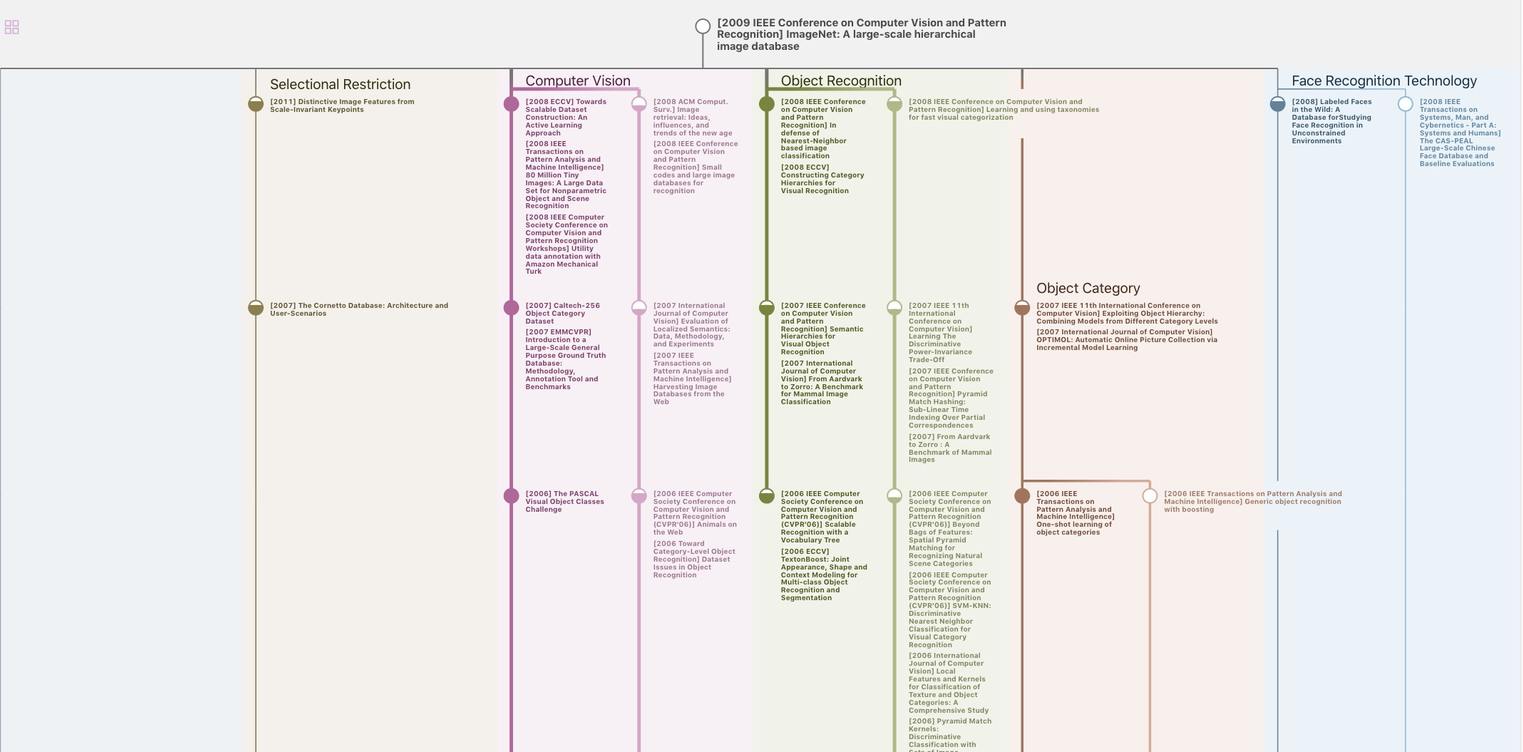
生成溯源树,研究论文发展脉络
Chat Paper
正在生成论文摘要