Stability of Feature Selection Methods - A Study of Metrics Across Different Gene Expression Datasets.
IWBBIO(2020)
摘要
Analysis of gene-expression data often requires that a gene (feature) subset is selected and many feature selection (FS) methods have been devised. However, FS methods often generate different lists of features for the same dataset and users then have to choose which list to use. One approach to support this choice is to apply stability metrics on the generated lists and selecting lists on that base. The aim of this study is to investigate the behavior of stability metrics applied to feature subsets generated by FS methods. The experiments in this work explore a plethora of gene expression datasets, FS methods, and expected number of features to compare several stability metrics. The stability metrics have been used to compare five feature selection methods (SVM, SAM, ReliefF, RFE + RF and LIMMA) on gene expression datasets from the EBI repository. Results show that the studied stability metrics display a high amount of variability. The reason behind this is not clear yet and is being further investigated. The final objective of the research, that is to define how to select a FS method, is an ongoing work whose partial findings are reported herein.
更多查看译文
关键词
feature selection methods,different gene expression datasets,selection methods,gene expression
AI 理解论文
溯源树
样例
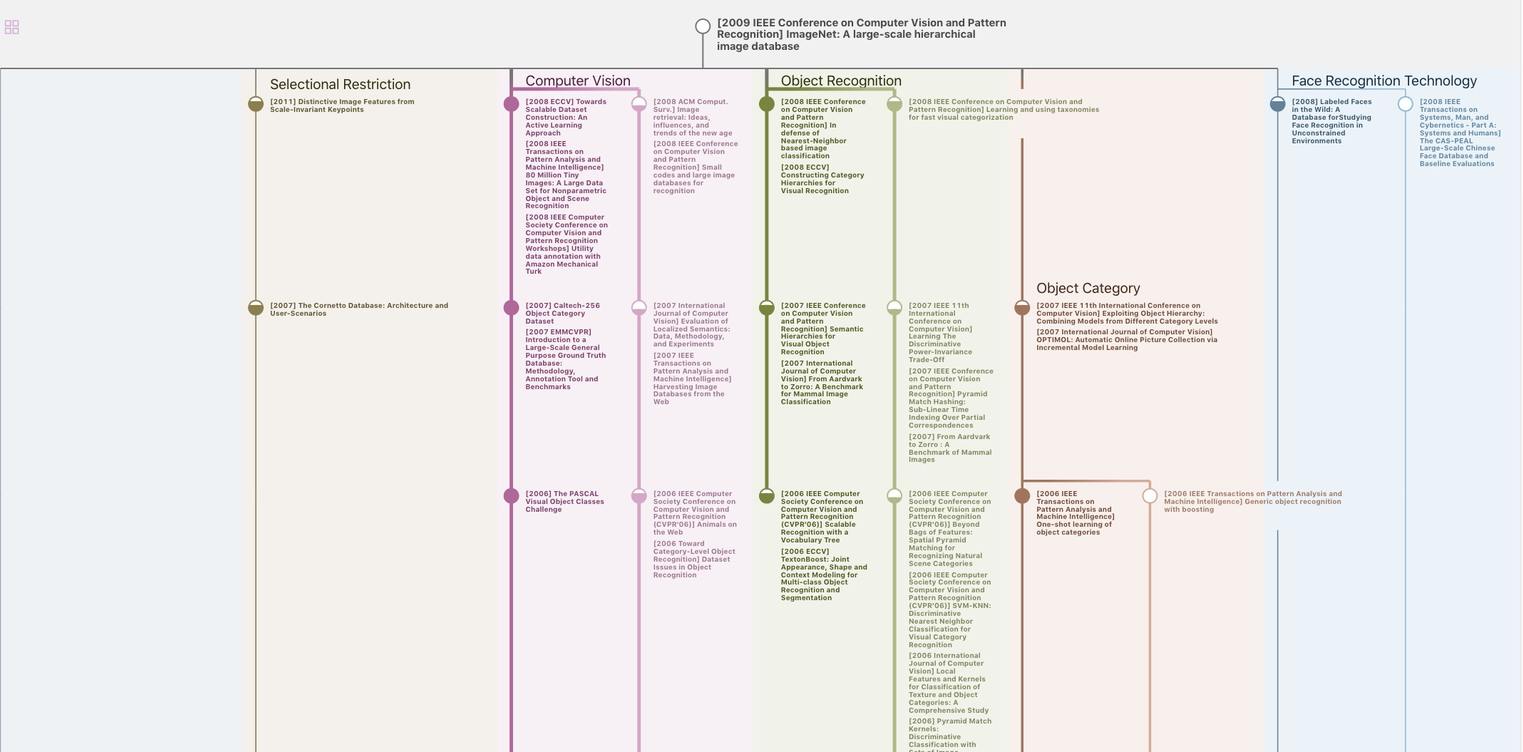
生成溯源树,研究论文发展脉络
Chat Paper
正在生成论文摘要