Combining Fractional Cover Images with One-Class Classifiers Enables Near Real-Time Monitoring of Fallows in the Northern Grains Region of Australia.
REMOTE SENSING(2020)
摘要
Fallows are widespread in dryland cropping systems. However, timely information about their spatial extent and location remains scarce. To overcome this lack of information, we propose to classify fractional cover data from Sentinel-2 with biased support vector machines. Fractional cover images describe the land surface in intuitive, biophysical terms, which reduces the spectral variability within the fallow class. Biased support vector machines are a type of one-class classifiers that require labelled data for the class of interest and unlabelled data for the other classes. They allow us to extrapolate in-situ observations collected during flowering to the rest of the growing season to generate large training data sets, thereby reducing the data collection requirements. We tested this approach to monitor fallows in the northern grains region of Australia and showed that the seasonal fallow extent can be mapped with >92% accuracy both during the summer and winter seasons. The summer fallow extent can be accurately mapped as early as mid-December (1-4 months before harvest). The winter fallow extent can be accurately mapped from mid-August (2-4 months before harvest). Our method also detected emergence dates successfully, indicating the near real-time accuracy of our method. We estimated that the extent of fallow fields across the northern grains region of Australia ranged between 50% in winter 2017 and 85% in winter 2019. Our method is scalable, sensor independent and economical to run. As such, it lays the foundations for reconstructing and monitoring the cropping dynamics in Australia.
更多查看译文
关键词
sentinel-2,biased support vector machine,cover fractions,classification,agriculture,crop emergence
AI 理解论文
溯源树
样例
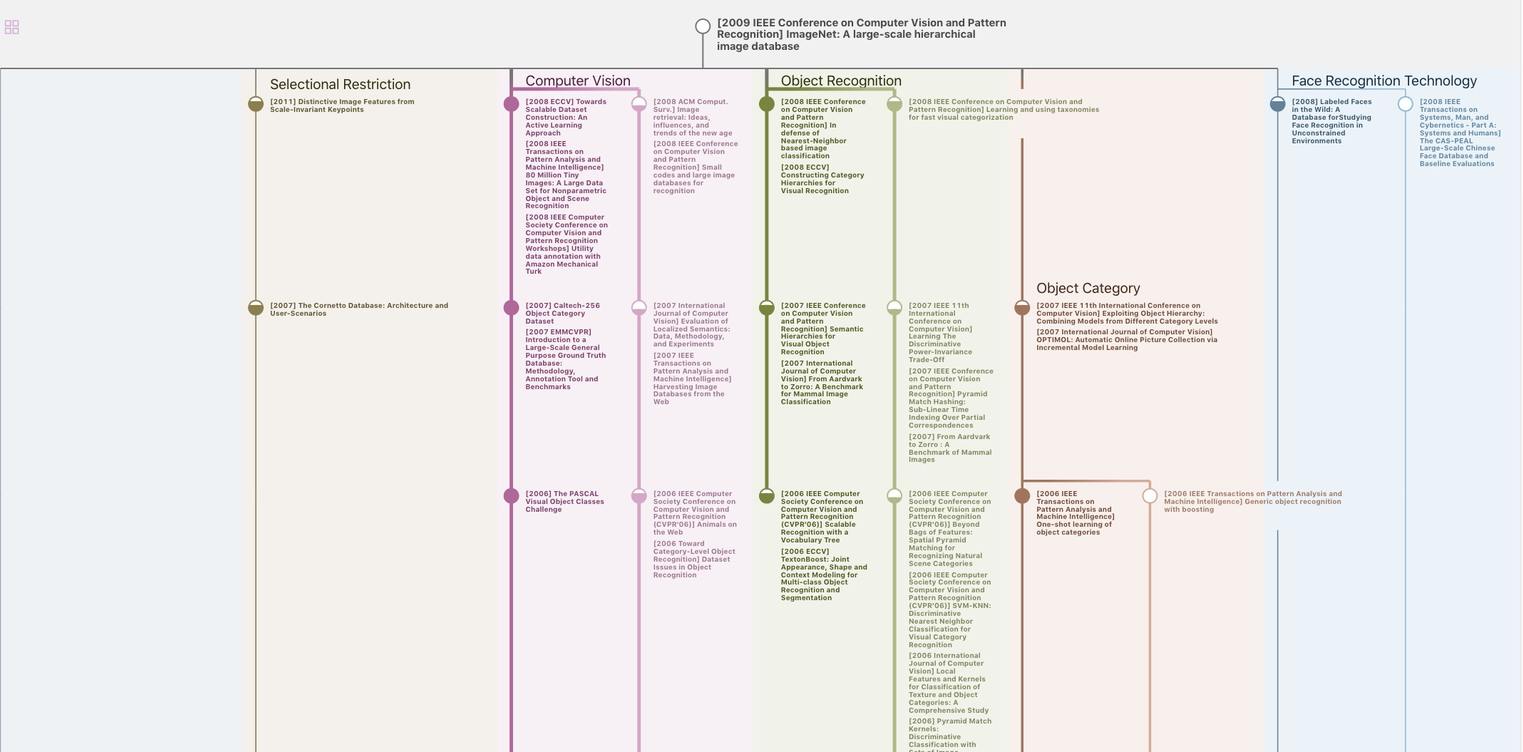
生成溯源树,研究论文发展脉络
Chat Paper
正在生成论文摘要