A Multi-hypothesis Learning Algorithm for Human and Mouse miRNA Target Prediction.
ICCABS(2019)
摘要
MicroRNAs (miRNAs) are small non-coding RNAs that play a key role in regulating gene expression and thus in many cellular activities. Dysfunction of cells in these tasks is correlated with the development of several kinds of cancer. As the functionality of miRNAs depends on the location of their binding on their targets, binding site prediction has received a lot of attention in the last several years. Despite its importance, the mechanisms of miRNA targeting are still unknown. In this paper, we introduce an algorithm that partitions miRNA target duplexes according to hypotheses that each represents a different mechanism of targeting. The algorithm, called multi-hypothesis learner, examines all possible hypotheses to find out the optimum data partitions according to the performance of these hypotheses for miRNA target prediction. These hypotheses were then utilized to build a superior target predictor for miRNAs. Our method exploited biologically meaningful features for recognizing targets, which enables establishment of hypotheses that can be correlated with target recognition mechanisms. Test results show that the algorithm can provide comparable performance to state-of-the-art machine learning tools such as RandomForest in predicting miRNA binding sites. Moreover, feature selection on the partitions in our method confirms that the partitioning mechanism is closely related to biological mechanisms of miRNA targeting. The resulting data partitions can potentially be used for in vivo experiments to aid in discovery of the targeting mechanisms.
更多查看译文
AI 理解论文
溯源树
样例
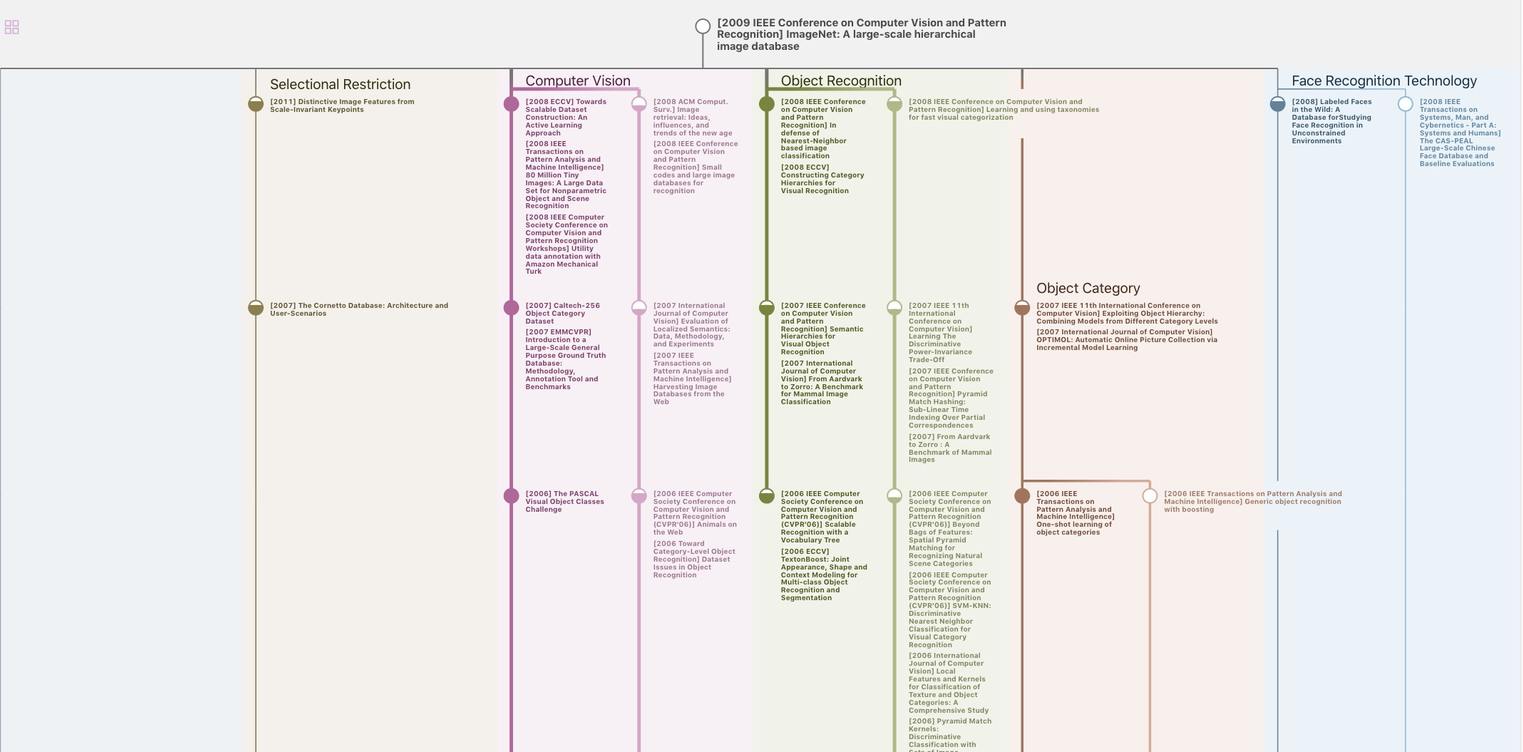
生成溯源树,研究论文发展脉络
Chat Paper
正在生成论文摘要