Knowledge Graph Empowered Entity Description Generation
arxiv(2020)
摘要
Existing works on KG-to-text generation take as input a few RDF triples or key-value pairs conveying the knowledge of some entities to generate a natural language description. Existing datasets, such as WikiBIO, WebNLG, and E2E, basically have a good alignment between an input triple/pair set and its output text. However in practice, the input knowledge could be more than enough, because the output description may only want to cover the most significant knowledge. In this paper, we introduce a large-scale and challenging dataset to facilitate the study of such practical scenario in KG-to-text. Our dataset involves exploring large knowledge graphs (KG) to retrieve abundant knowledge of various types of main entities, which makes the current graph-to-sequence models severely suffered from the problems of information loss and parameter explosion while generating the description text. We address these challenges by proposing a multi-graph structure that is able to represent the original graph information more comprehensively. Furthermore, we also incorporate aggregation methods that learn to ensemble the rich graph information. Extensive experiments demonstrate the effectiveness of our model architecture.
更多查看译文
关键词
description,knowledge
AI 理解论文
溯源树
样例
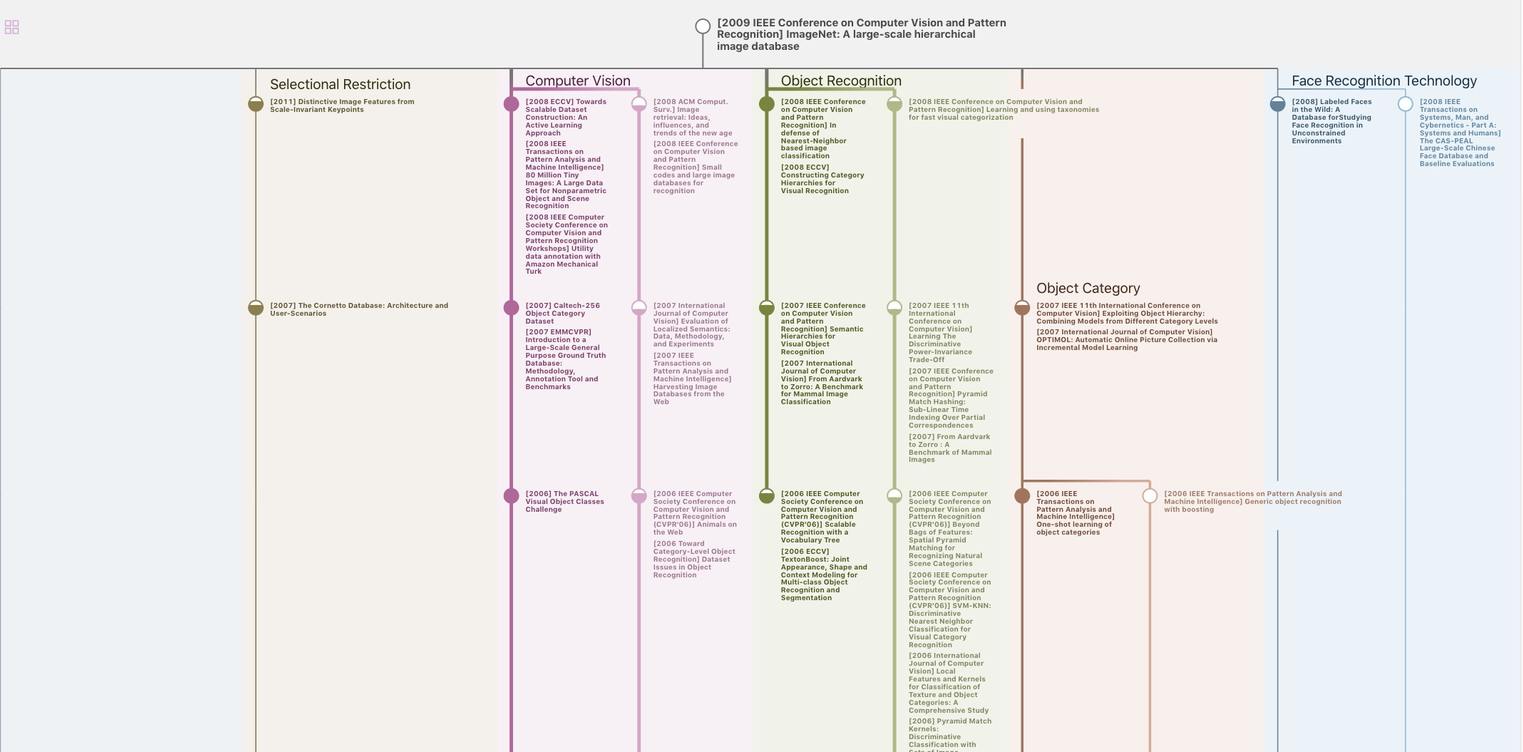
生成溯源树,研究论文发展脉络
Chat Paper
正在生成论文摘要