Unsupervised Injection of Knowledge into Dialogue Generation via Language Models
arxiv(2020)
摘要
Neural conversation models have shown the power to produce more meaningful and engaging responses given external knowledge. Specifically, the knowledge we experiment on is in textual form, for example, a personality description. Despite the success of training and testing with external knowledge, in reality, we do not always have sufficient background knowledge about the discussed topic. Therefore, it is also crucial to have the models generate captivating responses without external knowledge. To achieve this, we propose a unified training method, Decoupling, which induces a knowledge-related sentence and couples it with the dialogue history to generate a response in an unsupervised fashion. Its effect is further analyzed by testing the models with no knowledge, partial and full text of the knowledge. Empirically, we observed that the variance of the performance given different amounts of knowledge is significant. Also, our method performs more closely to the supervised method (the upper bound) than the baselines.
更多查看译文
关键词
dialogue generation,knowledge,language,models
AI 理解论文
溯源树
样例
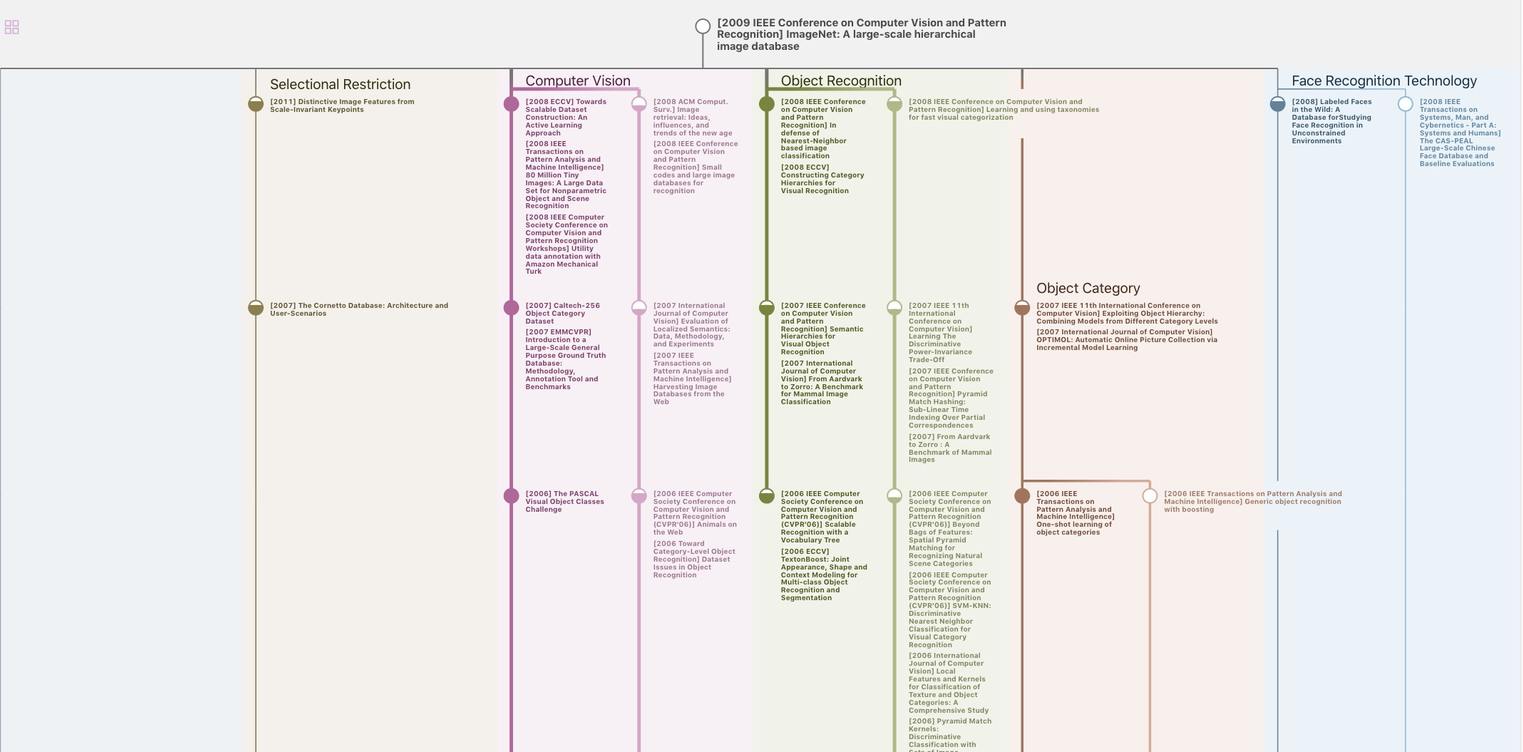
生成溯源树,研究论文发展脉络
Chat Paper
正在生成论文摘要