Automatic Machine Translation Evaluation in Many Languages via Zero-Shot Paraphrasing
Conference on Empirical Methods in Natural Language Processing(2020)
摘要
We frame the task of machine translation evaluation as one of scoring machine translation output with a sequence-to-sequence paraphraser, conditioned on a human reference. We propose training the paraphraser as a multilingual NMT system, treating paraphrasing as a zero-shot translation task (e.g., Czech to Czech). This results in the paraphraser’s output mode being centered around a copy of the input sequence, which represents the best case scenario where the MT system output matches a human reference. Our method is simple and intuitive, and does not require human judgements for training. Our single model (trained in 39 languages) outperforms or statistically ties with all prior metrics on the WMT 2019 segment-level shared metrics task in all languages (excluding Gujarati where the model had no training data). We also explore using our model for the task of quality estimation as a metric—conditioning on the source instead of the reference—and find that it significantly outperforms every submission to the WMT 2019 shared task on quality estimation in every language pair.
更多查看译文
关键词
automatic machine translation evaluation,many languages,zero-shot
AI 理解论文
溯源树
样例
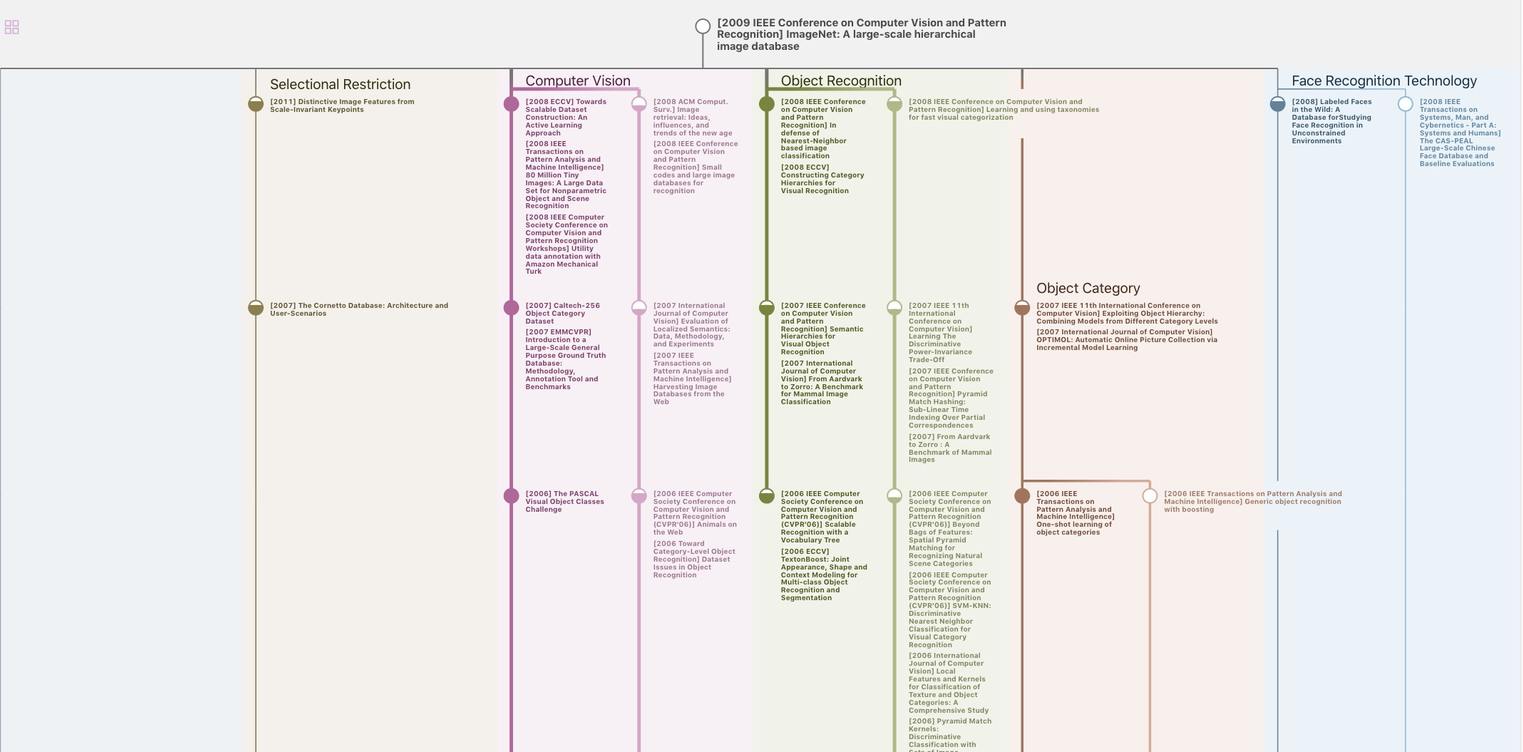
生成溯源树,研究论文发展脉络
Chat Paper
正在生成论文摘要