A deterministic equation to predict the accuracy of multi-population genomic prediction with multiple genomic relationship matrices
Genetics Selection Evolution(2020)
摘要
Background A multi-population genomic prediction (GP) model in which important pre-selected single nucleotide polymorphisms (SNPs) are differentially weighted (MPMG) has been shown to result in better prediction accuracy than a multi-population, single genomic relationship matrix ( 𝐆𝐑𝐌 ) GP model (MPSG) in which all SNPs are weighted equally. Our objective was to underpin theoretically the advantages and limits of the MPMG model over the MPSG model, by deriving and validating a deterministic prediction equation for its accuracy. Methods Using selection index theory, we derived an equation to predict the accuracy of estimated total genomic values of selection candidates from population A ( r_𝐄𝐆𝐕_A_T ), when individuals from two populations, A and B , are combined in the training population and two 𝐆𝐑𝐌 , made respectively from pre-selected and remaining SNPs, are fitted simultaneously in MPMG. We used simulations to validate the prediction equation in scenarios that differed in the level of genetic correlation between populations, heritability, and proportion of genetic variance explained by the pre-selected SNPs. Empirical accuracy of the MPMG model in each scenario was calculated and compared to the predicted accuracy from the equation. Results In general, the derived prediction equation resulted in accurate predictions of r_𝐄𝐆𝐕_A_T for the scenarios evaluated. Using the prediction equation, we showed that an important advantage of the MPMG model over the MPSG model is its ability to benefit from the small number of independent chromosome segments ( M_e ) due to the pre-selected SNPs, both within and across populations, whereas for the MPSG model, there is only a single value for M_e , calculated based on all SNPs, which is very large. However, this advantage is dependent on the pre-selected SNPs that explain some proportion of the total genetic variance for the trait. Conclusions We developed an equation that gives insight into why, and under which conditions the MPMG outperforms the MPSG model for GP. The equation can be used as a deterministic tool to assess the potential benefit of combining information from different populations, e.g., different breeds or lines for GP in livestock or plants, or different groups of people based on their ethnic background for prediction of disease risk scores.
更多查看译文
AI 理解论文
溯源树
样例
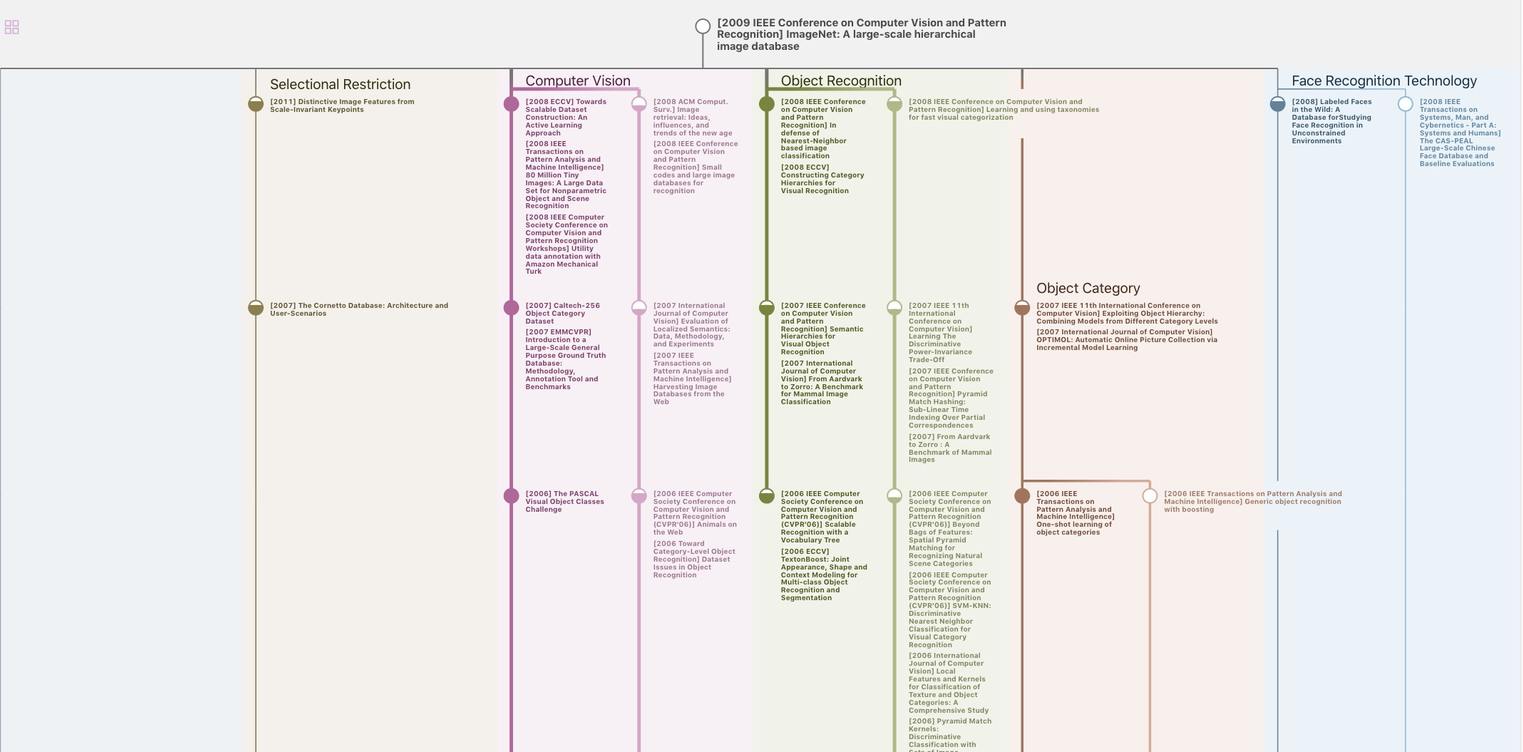
生成溯源树,研究论文发展脉络
Chat Paper
正在生成论文摘要