Nonconvex Low-Rank Kernel Sparse Subspace Learning for Keyframe Extraction and Motion Segmentation
IEEE Transactions on Neural Networks and Learning Systems(2021)
摘要
By exploiting the kernel trick, the sparse subspace model is extended to the nonlinear version with one or a combination of predefined kernels, but the high-dimensional space induced by predefined kernels is not guaranteed to be able to capture the features of the nonlinear data in theory. In this article, we propose a nonconvex low-rank learning framework in an unsupervised way to learn a kernel to replace the predefined kernel in the sparse subspace model. The learned kernel by a nonconvex relaxation of rank can better exploiting the low-rank property of nonlinear data to induce a high-dimensional Hilbert space that more closely approaches the true feature space. Furthermore, we give a global closed-form optimal solution of the nonconvex rank minimization and prove it. Considering the low-rank and sparseness characteristics of motion capture data in its feature space, we use them to verify the better representation of nonlinear data with the learned kernel via two tasks: keyframe extraction and motion segmentation. The performances on both tasks demonstrate the advantage of our model over the sparse subspace model with predefined kernels and some other related state-of-art methods.
更多查看译文
关键词
Kernel,Data models,Minimization,Motion segmentation,Feature extraction,Computer vision,Computational modeling
AI 理解论文
溯源树
样例
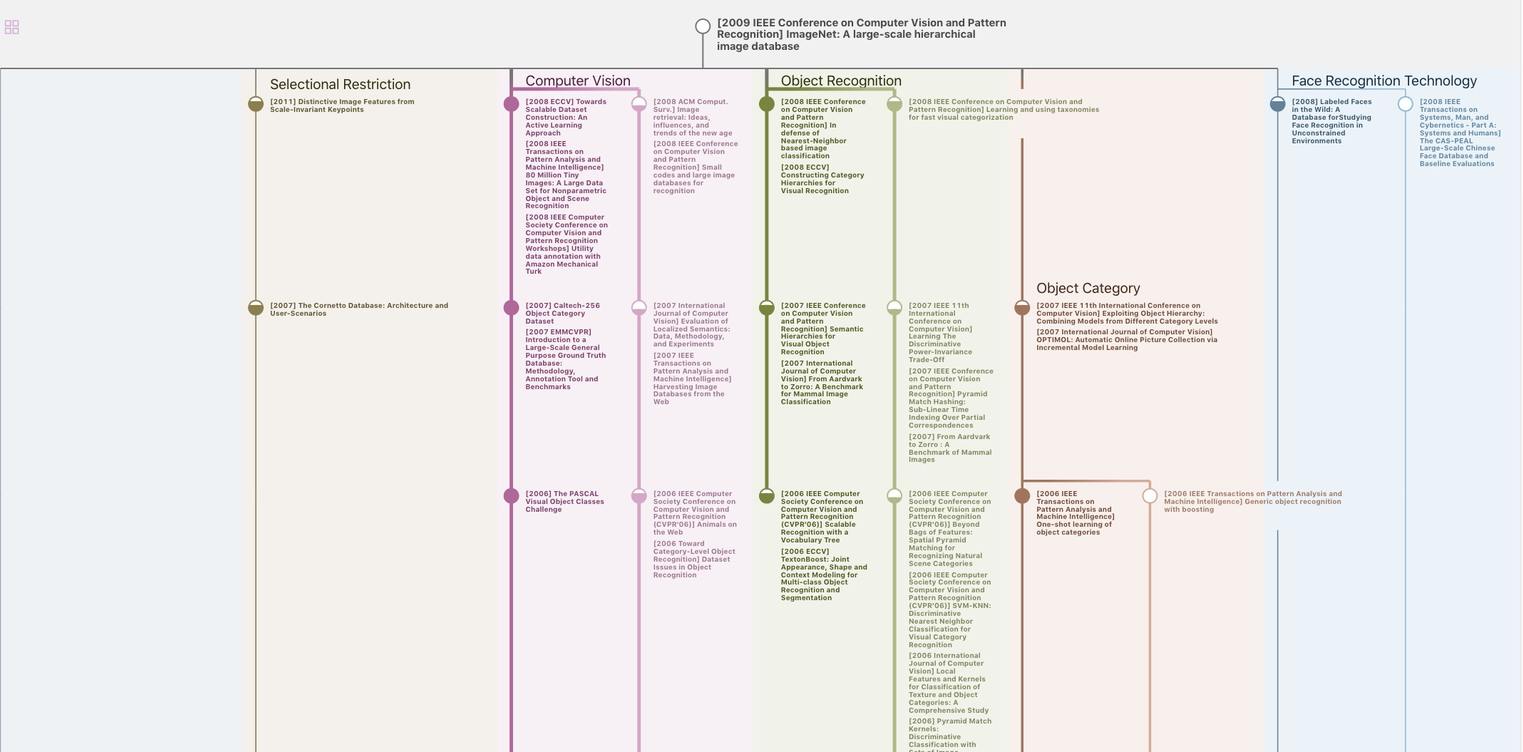
生成溯源树,研究论文发展脉络
Chat Paper
正在生成论文摘要