On Distributed Nonconvex Optimization: Projected Subgradient Method for Weakly Convex Problems in Networks
arxiv(2022)
摘要
The stochastic subgradient method is a widely used algorithm for solving large-scale optimization problems arising in machine learning. Often, these problems are neither smooth nor convex. Recently, Davis
et al.
, 2018 characterized the convergence of the stochastic subgradient method for the weakly convex case, which encompasses many important applications (e.g., robust phase retrieval, blind deconvolution, biconvex compressive sensing, and dictionary learning). In practice, distributed implementations of the projected stochastic subgradient method (stoDPSM) are used to speed up risk minimization. In this article, we propose a distributed implementation of the stochastic subgradient method with a theoretical guarantee. Specifically, we show the global convergence of stoDPSM using the Moreau envelope stationarity measure. Furthermore, under a so-called sharpness condition, we show that deterministic DPSM (with a proper initialization) converges linearly to the sharp minima, using geometrically diminishing step size. We provide numerical experiments to support our theoretical analysis.
更多查看译文
关键词
Distributed optimization,multiagent systems,nonconvex optimization,projected stochastic subgradient method
AI 理解论文
溯源树
样例
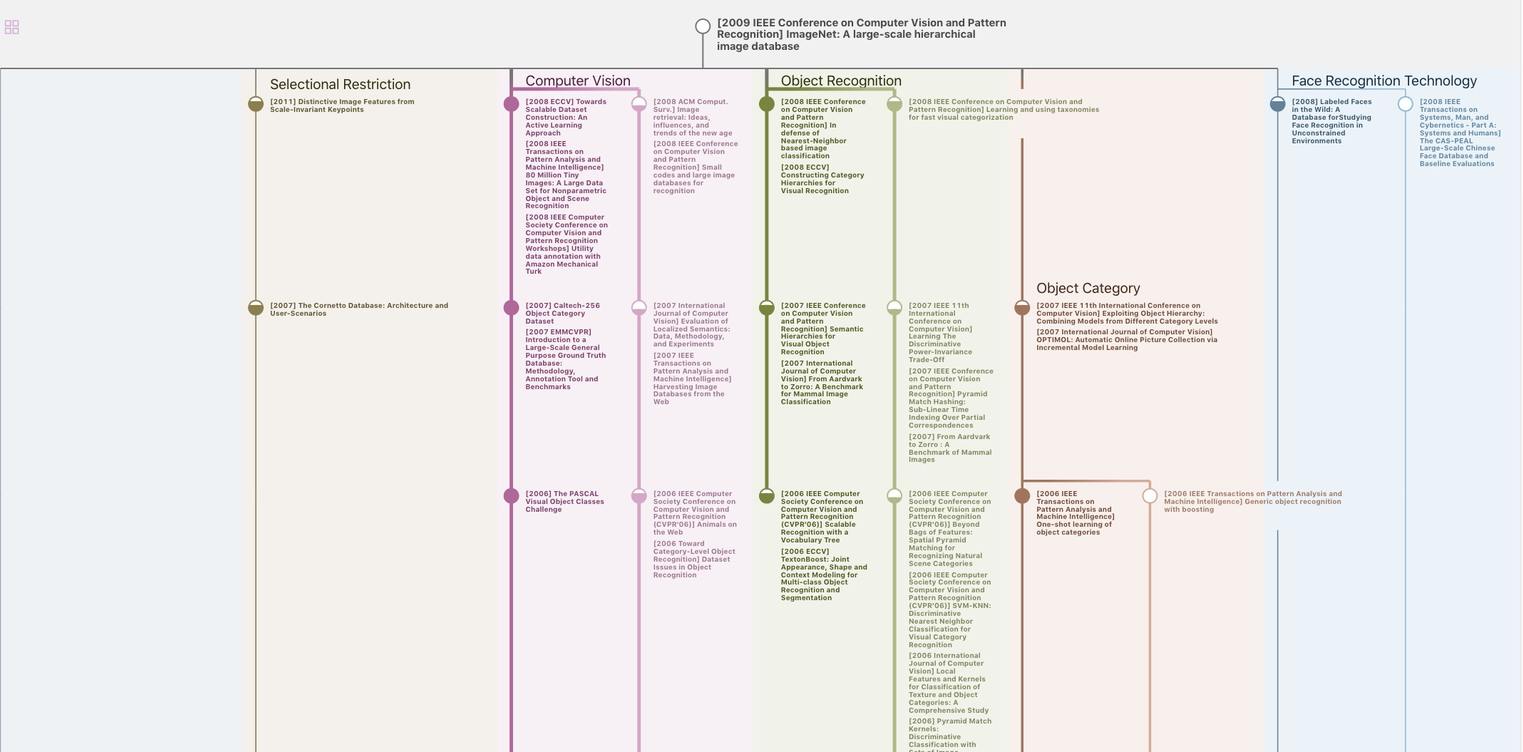
生成溯源树,研究论文发展脉络
Chat Paper
正在生成论文摘要