Temporal data classification and forecasting using a memristor-based reservoir computing system
NATURE ELECTRONICS(2019)
摘要
Time-series analysis including forecasting is essential in a range of fields from finance to engineering. However, long-term forecasting is difficult, particularly for cases where the underlying models and parameters are complex and unknown. Neural networks can effectively process features in temporal units and are attractive for such purposes. Reservoir computing, in particular, can offer efficient temporal processing of recurrent neural networks with a low training cost, and is thus well suited to time-series analysis and forecasting tasks. Here, we report a reservoir computing hardware system based on dynamic tungsten oxide (WO x ) memristors that can efficiently process temporal data. The internal short-term memory effects of the WO x memristors allow the memristor-based reservoir to nonlinearly map temporal inputs into reservoir states, where the projected features can be readily processed by a linear readout function. We use the system to experimentally demonstrate two standard benchmarking tasks: isolated spoken-digit recognition with partial inputs, and chaotic system forecasting. A high classification accuracy of 99.2% is obtained for spoken-digit recognition, and autonomous chaotic time-series forecasting has been demonstrated over the long term.
更多查看译文
关键词
Electrical and electronic engineering,Engineering,Nanoscience and technology,Electrical Engineering
AI 理解论文
溯源树
样例
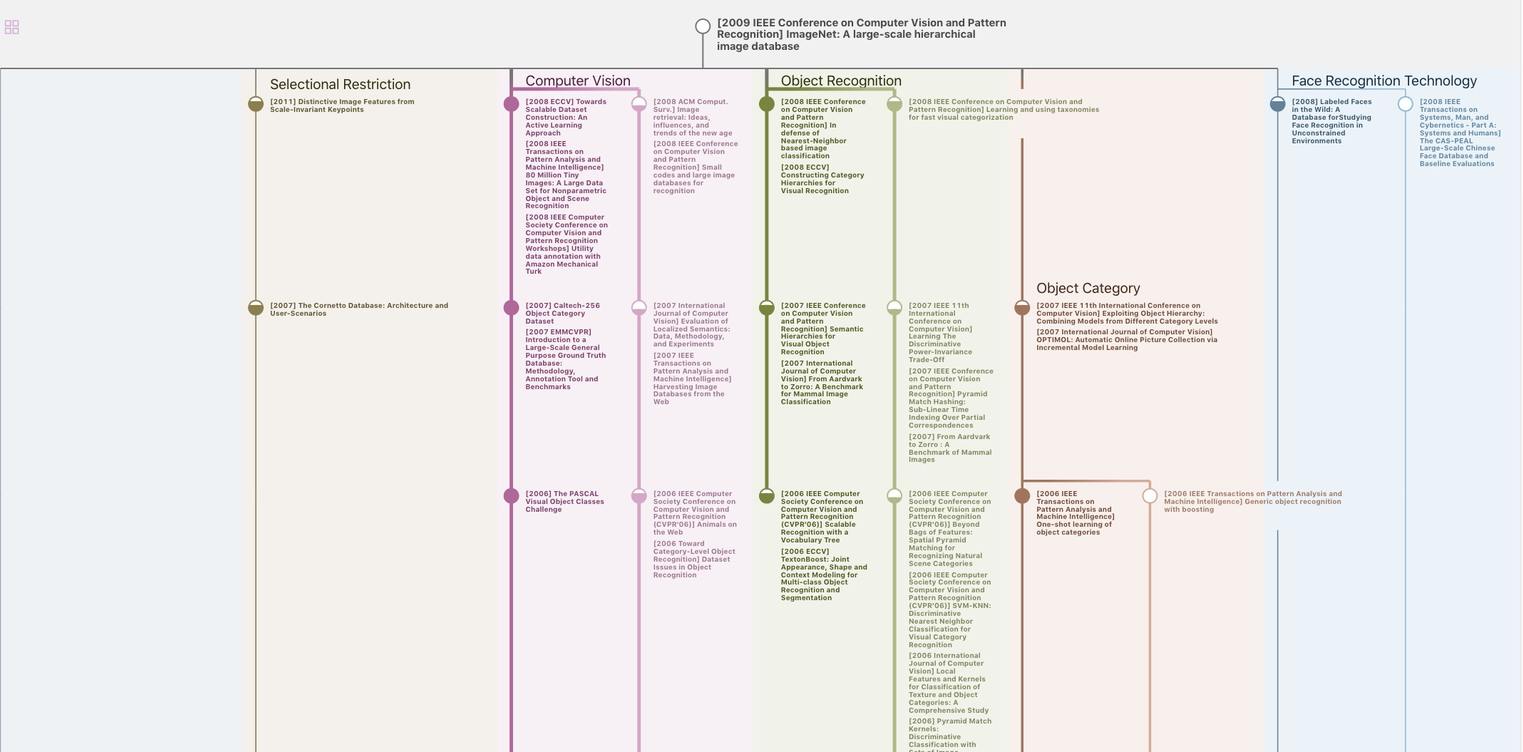
生成溯源树,研究论文发展脉络
Chat Paper
正在生成论文摘要