RSeiz: A Channel Selection Based Approach for Rapid Seizure Detection in the IoMT
2019 IEEE International Symposium on Smart Electronic Systems (iSES) (Formerly iNiS)(2019)
摘要
Epilepsy affects 1% of the world population, which necessitates a fast seizure detection system for practical epilepsy solutions. The reduction of seizure detection delay is a critical problem which needs to be addressed as rapid detection provides effective treatment. In this paper an electroencephalogram (EEG) based, patient-specific seizure detection system is proposed in the Internet of Medical Things (IoMT) framework which can detect seizures at a minimum delay. The proposed system uses neighborhood component analysis (NCA) for channel selection, statistical features for optimal feature extraction, and a ReliefFbased optimization (RBO) in conjunction with a k-nearest neighbor classifier for feature classification. A publicly available database (CHB-MIT EEG) has been used for evaluation of the proposed algorithm. The simulation results show that the proposed algorithm provides a sensitivity of 100% while maintaining a low average latency of 1.49 sec, which may be useful for practical epilepsy treatment and biomedical applications.
更多查看译文
关键词
Internet of Medical Things (IoMT), Smart Healthcare, Seizure Detection, Seizure Early Detection, Electroencephalogram (EEG), Epilepsy
AI 理解论文
溯源树
样例
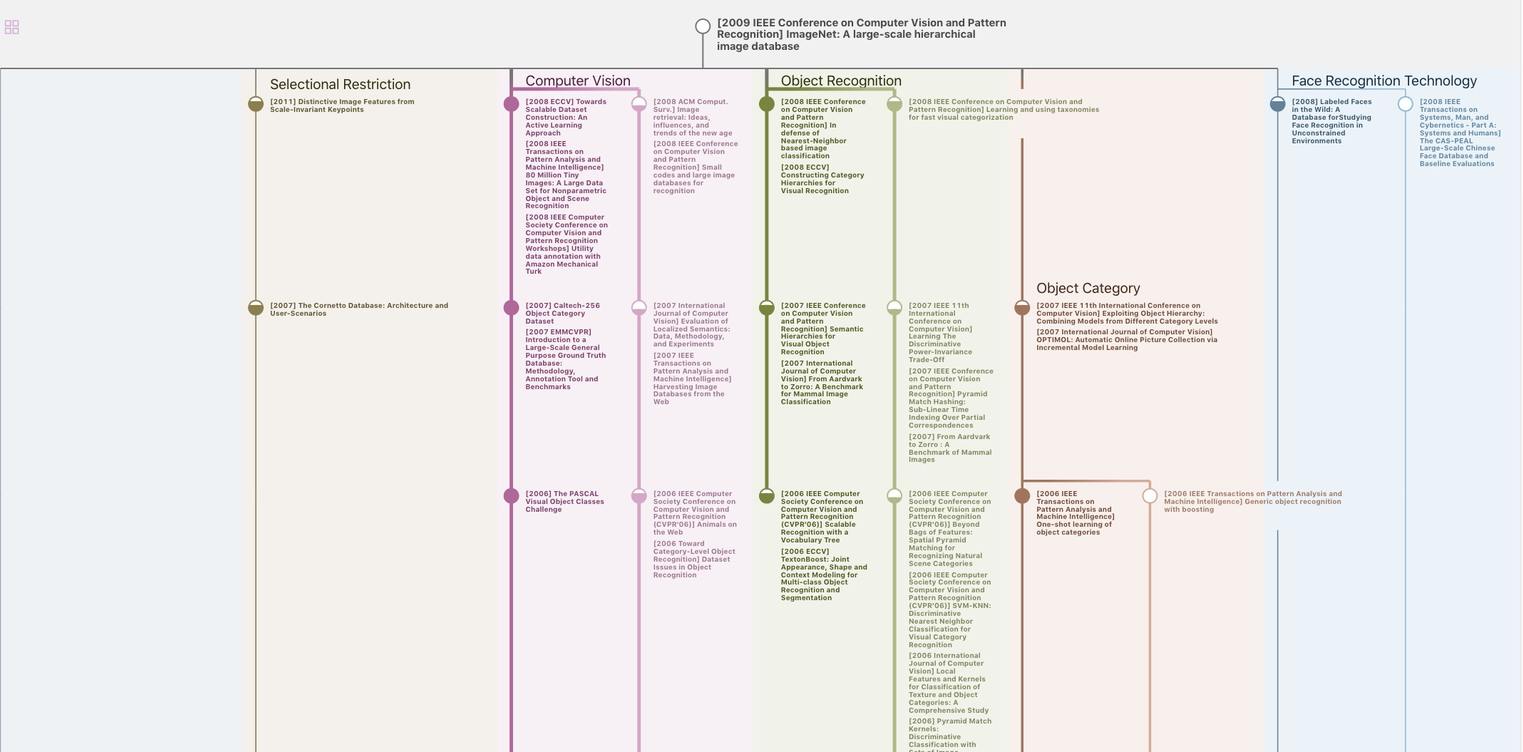
生成溯源树,研究论文发展脉络
Chat Paper
正在生成论文摘要