Quantifying the Contextualization of Word Representations with Semantic Class Probing
EMNLP(2020)
摘要
Pretrained language models have achieved a new state of the art on many NLP tasks, but there are still many open questions about how and why they work so well. We investigate the contextualization of words in BERT. We quantify the amount of contextualization, i.e., how well words are interpreted in context, by studying the extent to which semantic classes of a word can be inferred from its contextualized embeddings. Quantifying contextualization helps in understanding and utilizing pretrained language models. We show that top layer representations achieve high accuracy inferring semantic classes; that the strongest contextualization effects occur in the lower layers; that local context is mostly sufficient for semantic class inference; and that top layer representations are more task-specific after finetuning while lower layer representations are more transferable. Finetuning uncovers task related features, but pretrained knowledge is still largely preserved.
更多查看译文
关键词
word representations,contextualization,class probing,semantic
AI 理解论文
溯源树
样例
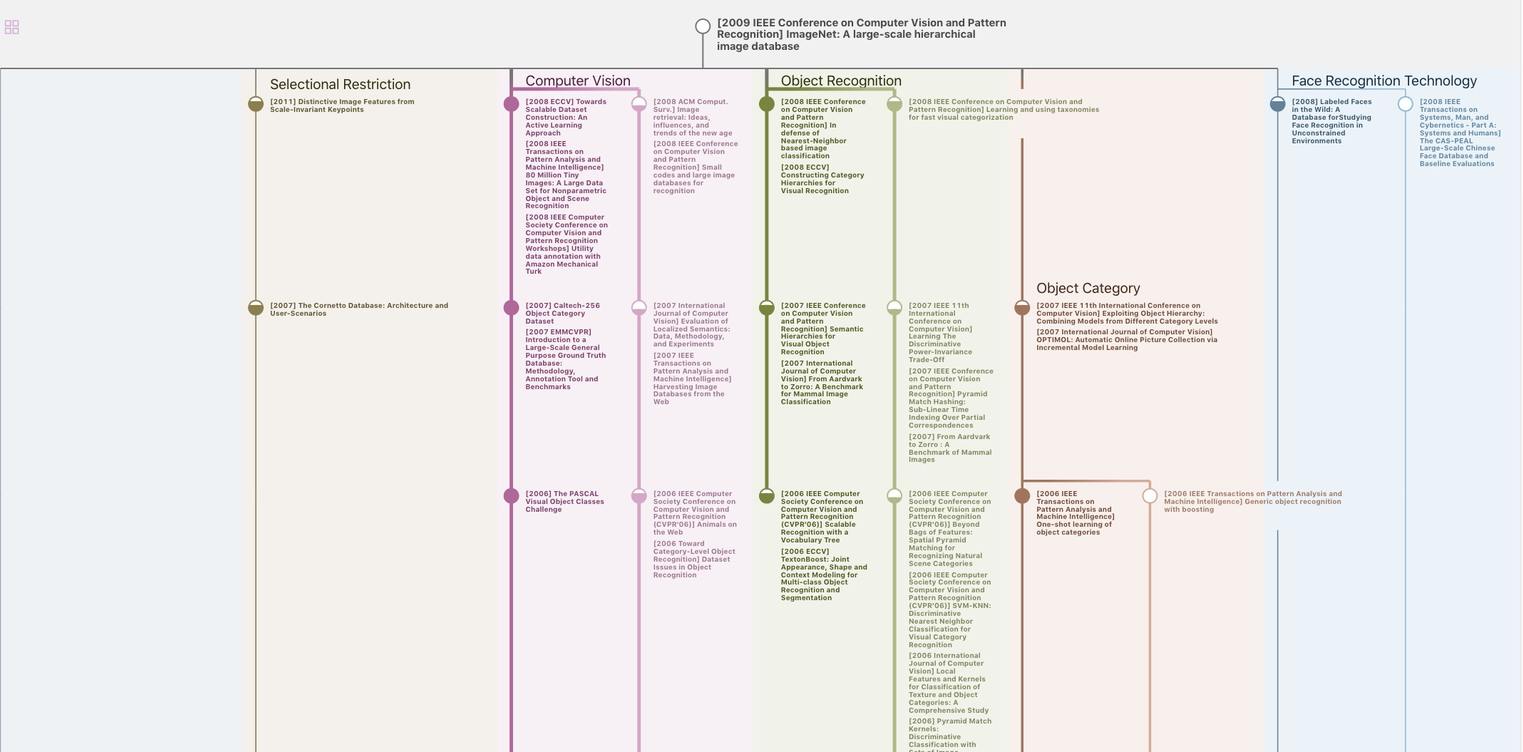
生成溯源树,研究论文发展脉络
Chat Paper
正在生成论文摘要