Revisiting Sequence-to-Sequence Video Object Segmentation with Multi-Task Loss and Skip-Memory.
2020 25TH INTERNATIONAL CONFERENCE ON PATTERN RECOGNITION (ICPR)(2020)
摘要
Video Object Segmentation (VOS) is an active research area of the visual domain. One of its fundamental subtasks is semi-supervised / one-shot learning: given only the segmentation mask for the first frame, the task is to provide pixel-accurate masks for the object over the rest of the sequence. Despite much progress in the last years, we noticed that many of the existing approaches lose objects in longer sequences, especially when the object is small or briefly occluded. In this work, we build upon a sequence-to-sequence approach that employs an encoder-decoder architecture together with a memory module for exploiting the sequential data. We further improve this approach by proposing a model that manipulates multiscale spatio-temporal information using memory-equipped skip connections. Furthermore, we incorporate an auxiliary task based on distance classification which greatly enhances the quality of edges in segmentation masks. We compare our approach to the state of the art and show considerable improvement in the contour accuracy metric and the overall segmentation accuracy. Our source code and the pre-trained weights are publicly available
11
https://github.com/fatemehazimi990/RS2S.
更多查看译文
关键词
sequence-to-sequence video Object Segmentation,multitask loss,skip-memory,visual domain,segmentation mask,pixel-accurate masks,sequence-to-sequence approach,memory module,multiscale spatio-temporal information,memory-equipped skip connections,auxiliary task,segmentation accuracy
AI 理解论文
溯源树
样例
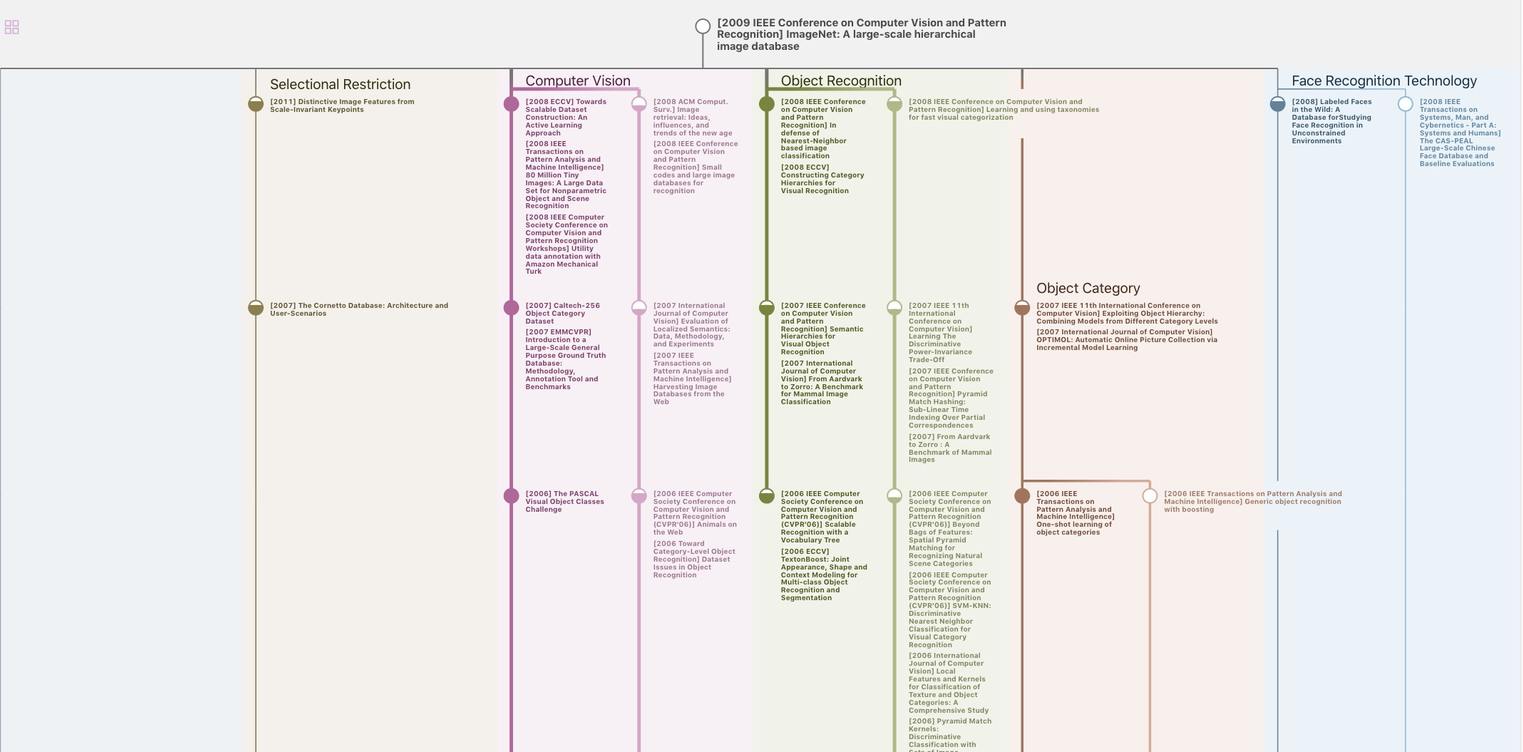
生成溯源树,研究论文发展脉络
Chat Paper
正在生成论文摘要