Numerical Solution of the Parametric Diffusion Equation by Deep Neural Networks
J. Sci. Comput.(2021)
摘要
We perform a comprehensive numerical study of the effect of approximation-theoretical results for neural networks on practical learning problems in the context of numerical analysis. As the underlying model, we study the machine-learning-based solution of parametric partial differential equations. Here, approximation theory for fully-connected neural networks predicts that the performance of the model should depend only very mildly on the dimension of the parameter space and is determined by the intrinsic dimension of the solution manifold of the parametric partial differential equation. We use various methods to establish comparability between test-cases by minimizing the effect of the choice of test-cases on the optimization and sampling aspects of the learning problem. We find strong support for the hypothesis that approximation-theoretical effects heavily influence the practical behavior of learning problems in numerical analysis. Turning to practically more successful and modern architectures, at the end of this study we derive improved error bounds by focusing on convolutional neural networks.
更多查看译文
关键词
Neural networks,Parametric diffusion equation,Numerical approximation,Neural network capacity,35J99,41A25,41A30,68T05,65N30
AI 理解论文
溯源树
样例
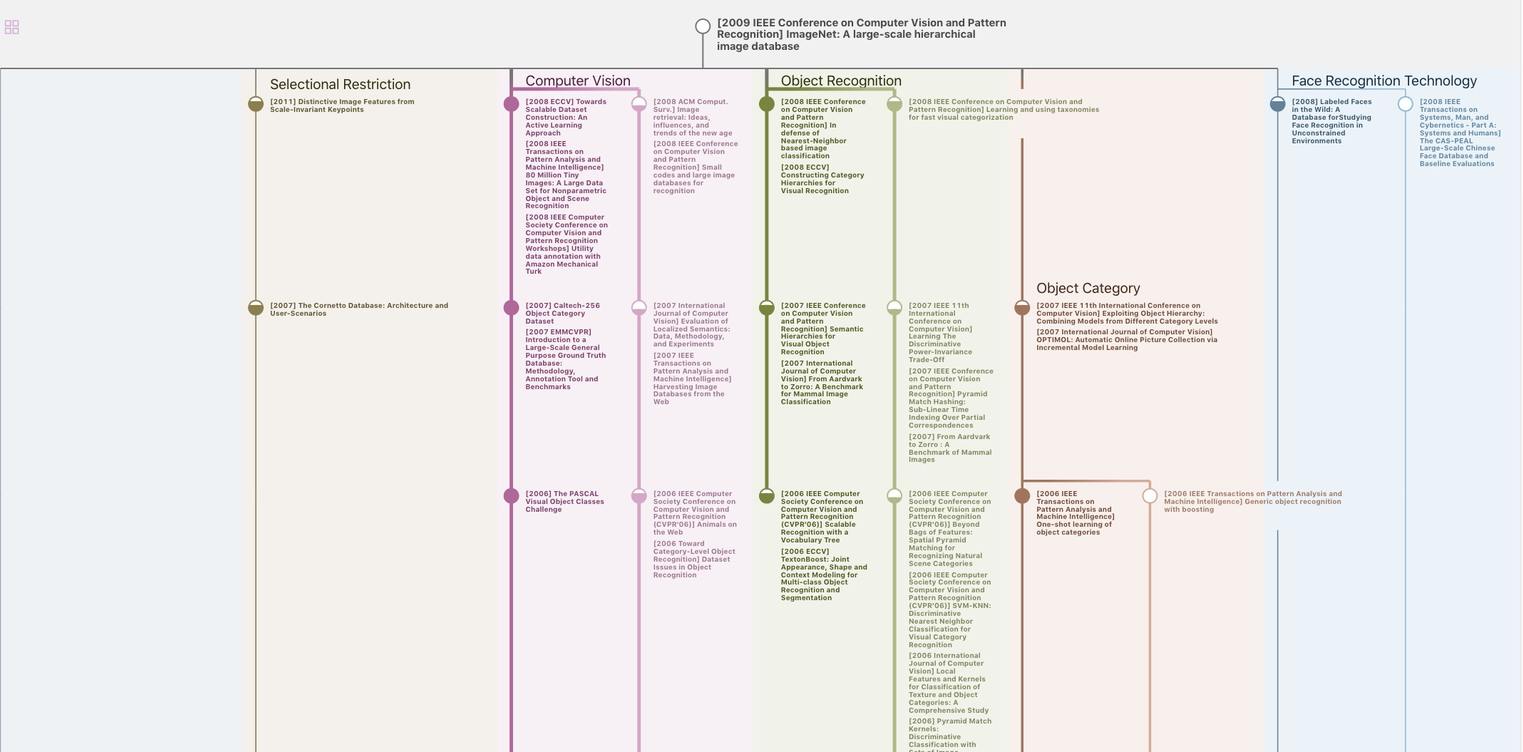
生成溯源树,研究论文发展脉络
Chat Paper
正在生成论文摘要