Inter-sequence Enhanced Framework for Personalized Sequential Recommendation
arxiv(2020)
摘要
Modeling the sequential correlation of users' historical interactions is essential in sequential recommendation. However, the majority of the approaches mainly focus on modeling the \emph{intra-sequence} item correlation within each individual sequence but neglect the \emph{inter-sequence} item correlation across different user interaction sequences. Though several studies have been aware of this issue, their method is either simple or implicit. To make better use of such information, we propose an inter-sequence enhanced framework for the Sequential Recommendation (ISSR). In ISSR, both inter-sequence and intra-sequence item correlation are considered. Firstly, we equip graph neural networks in the inter-sequence correlation encoder to capture the high-order item correlation from the user-item bipartite graph and the item-item graph. Then, based on the inter-sequence correlation encoder, we build GRU network and attention network in the intra-sequence correlation encoder to model the item sequential correlation within each individual sequence and temporal dynamics for predicting users' preferences over candidate items. Additionally, we conduct extensive experiments on three real-world datasets. The experimental results demonstrate the superiority of ISSR over many state-of-the-art methods and the effectiveness of the inter-sequence correlation encoder.
更多查看译文
关键词
personalized sequential recommendation,inter-sequence
AI 理解论文
溯源树
样例
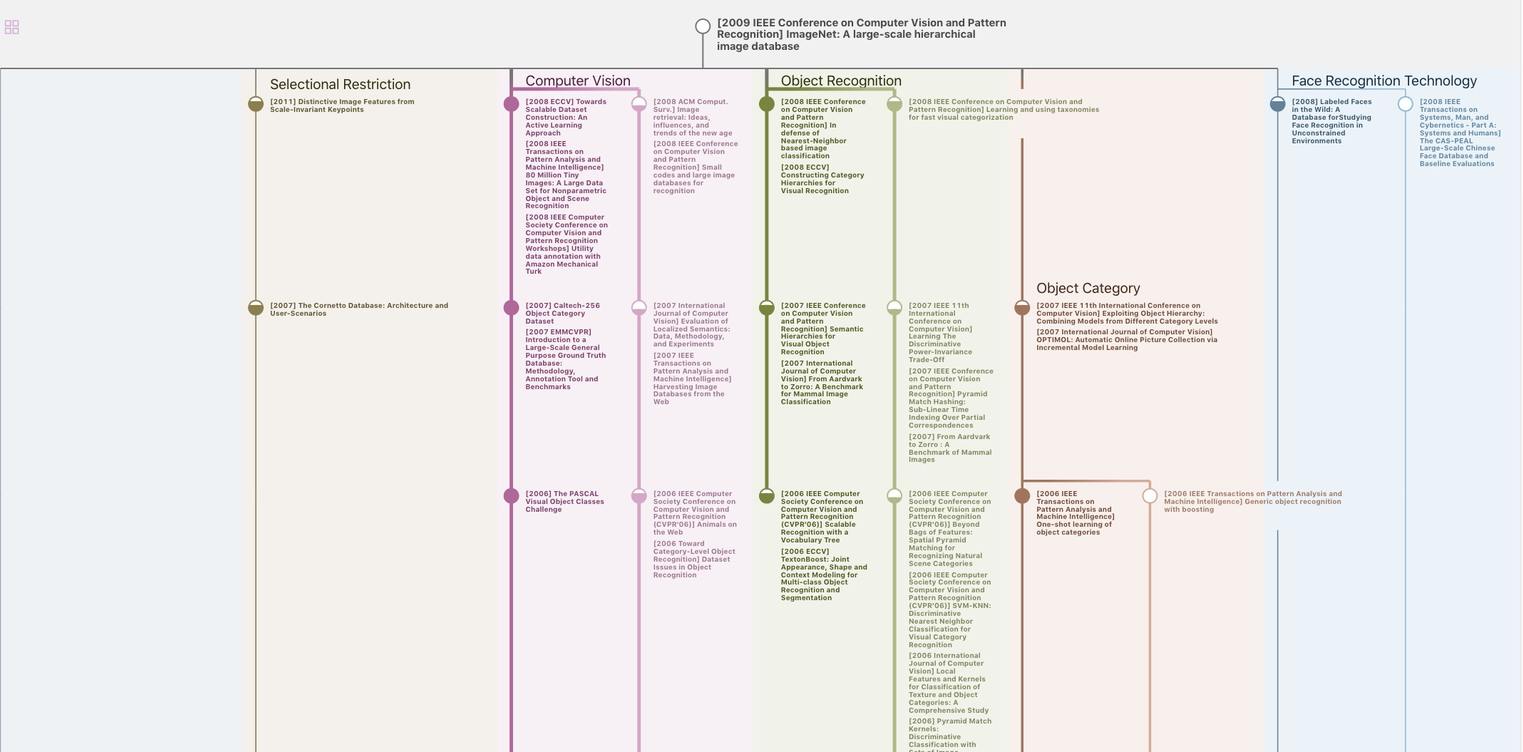
生成溯源树,研究论文发展脉络
Chat Paper
正在生成论文摘要