Revisiting Modulated Convolutions for Visual Counting and Beyond
arxiv(2020)
摘要
This paper targets at visual counting, where the setup is to estimate the total number of occurrences in a natural image given an input query (e.g. a question or a category). Most existing work for counting focuses on explicit, symbolic models that iteratively examine relevant regions to arrive at the final number, mimicking the intuitive process specifically for counting. However, such models can be computationally expensive, and more importantly place a limit on their generalization to other reasoning tasks. In this paper, we propose a simple and effective alternative for visual counting by revisiting modulated convolutions that fuse query and image locally. The modulation is performed on a per-bottleneck basis by fusing query representations with input convolutional feature maps of that residual bottleneck. Therefore, we call our method MoVie, short for Modulated conVolutional bottleneck. Notably, MoVie reasons implicitly and holistically for counting and only needs a single forward-pass during inference. Nevertheless, MoVie showcases strong empirical performance. First, it significantly advances the state-of-the-art accuracies on multiple counting-specific Visual Question Answering (VQA) datasets (i.e., HowMany-QA and TallyQA). Moreover, it also works on common object counting, outperforming prior-art on difficult benchmarks like COCO. Third, integrated as a module, MoVie can be used to improve number-related questions for generic VQA models. Finally, we find MoVie achieves similar, near-perfect results on CLEVR and competitive ones on GQA, suggesting modulated convolutions as a mechanism can be useful for more general reasoning tasks beyond counting.
更多查看译文
关键词
visual counting,modulated convolutions,movie
AI 理解论文
溯源树
样例
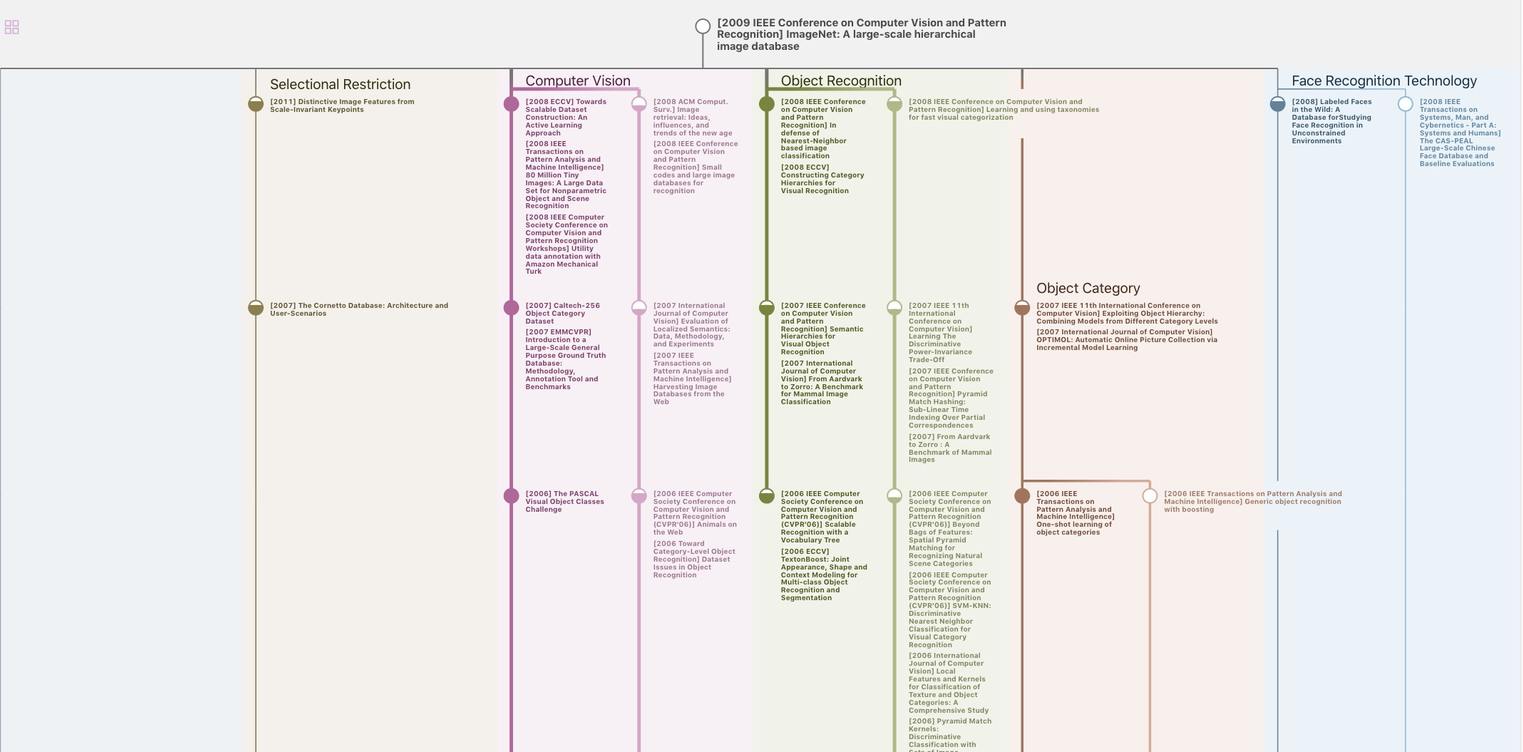
生成溯源树,研究论文发展脉络
Chat Paper
正在生成论文摘要