Towards Fast and Accurate Streaming End-to-End ASR
ICASSP(2020)
摘要
End-to-end (E2E) models fold the acoustic, pronunciation and language models of a conventional speech recognition model into one neural network with a much smaller number of parameters than a conventional ASR system, thus making it suitable for on-device applications. For example, recurrent neural network transducer (RNN-T) as a streaming E2E model has shown promising potential for on-device ASR. For such applications, quality and latency are two critical factors. We propose to reduce E2E model's latency by extending the RNN-T endpointer (RNN-T EP) model with additional early and late penalties. By further applying the minimum word error rate (MWER) training technique, we achieved 8.0% relative word error rate (WER) reduction and 130ms 90-percentile latency reduction over on a Voice Search test set. We also experimented with a second-pass Listen, Attend and Spell (LAS) rescorer . Although it did not directly improve the first pass latency, the large WER reduction provides extra room to trade WER for latency. RNN-T EP+LAS, together with MWER training brings in 18.7% relative WER reduction and 160ms 90-percentile latency reductions compared to the original proposed RNN-T EP model.
更多查看译文
关键词
RNN-T,Endpointer,Latency
AI 理解论文
溯源树
样例
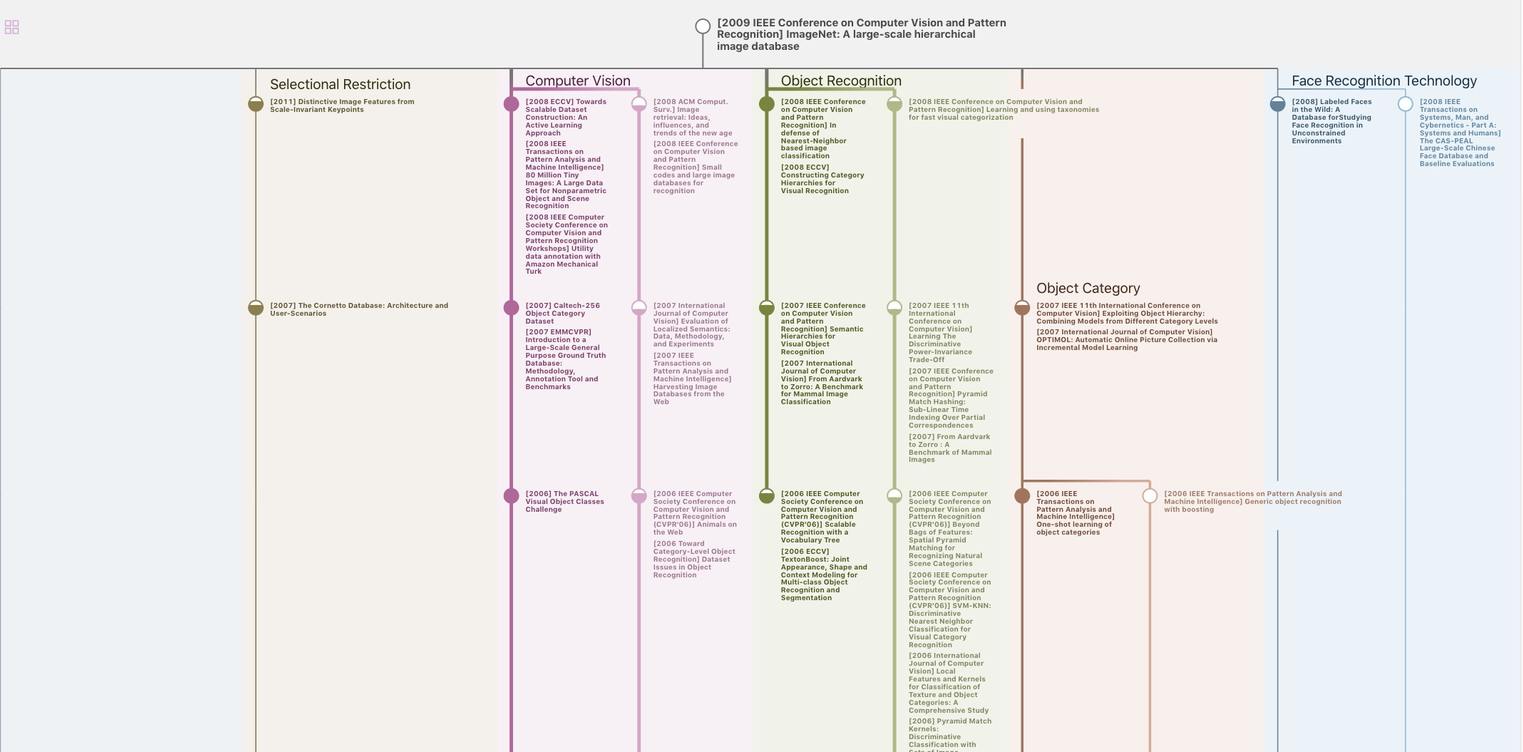
生成溯源树,研究论文发展脉络
Chat Paper
正在生成论文摘要