Rotated Spectral Principal Component Analysis (Rspca) For Identifying Dynamical Modes Of Variability In Climate Systems
JOURNAL OF CLIMATE(2021)
摘要
Spectral PCA (sPCA), in contrast to classical PCA, offers the advantage of identifying organized spatio-temporal patterns within specific frequency bands and extracting dynamical modes. However, the unavoidable trade-off between frequency resolution and robustness of the PCs leads to high sensitivity to noise and overfitting, which limits the interpretation of the sPCA results. We propose herein a simple nonparametric implementation of sPCA using the continuous analytic Morlet wavelet as a robust estimator of the cross-spectral matrices with good frequency resolution. To improve the interpretability of the results, especially when several modes of similar amplitude exist within the same frequency band, we propose a rotation of the complex-valued eigenvectors to optimize their spatial regularity (smoothness). The developed method, called rotated spectral PCA (rsPCA), is tested on synthetic data simulating propagating waves and shows impressive performance even with high levels of noise in the data. Applied to global historical geopotential height (GPH) and sea surface temperature (SST) daily time series, the method accurately captures patterns of atmospheric Rossby waves at high frequencies (3-60-day periods) in both GPH and SST and El Nino-Southern Oscillation (ENSO) at low frequencies (2-7-yr periodicity) in SST. At high frequencies the rsPCA successfully unmixes the identified waves, revealing spatially coherent patterns with robust propagation dynamics.
更多查看译文
关键词
Dynamics, Sea surface temperature, Pressure, Empirical orthogonal functions, Pattern detection, Spectral analysis/models/distribution
AI 理解论文
溯源树
样例
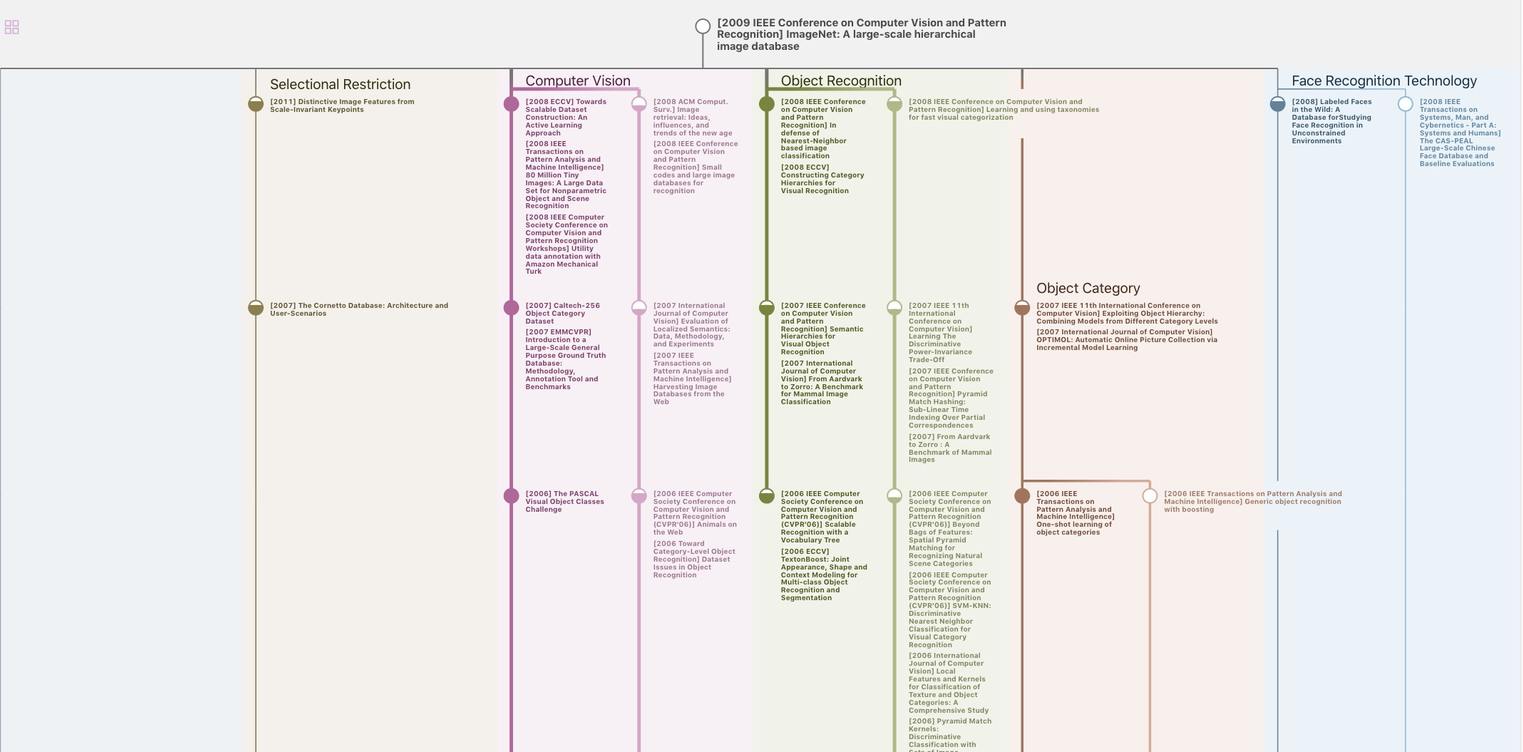
生成溯源树,研究论文发展脉络
Chat Paper
正在生成论文摘要