Detecting Gender Stereotypes: Lexicon vs. Supervised Learning Methods
CHI '20: CHI Conference on Human Factors in Computing Systems Honolulu HI USA April, 2020(2020)
摘要
Biases in language influence how we interact with each other and society at large. Language affirming gender stereotypes is often observed in various contexts today, from recommendation letters and Wikipedia entries to fiction novels and movie dialogue. Yet to date, there is little agreement on the methodology to quantify gender stereotypes in natural language (specifically the English language). Common methodology (including those adopted by companies tasked with detecting gender bias) rely on a lexicon approach largely based on the original BSRI study from 1974.
In this paper, we reexamine the role of gender stereotype detection in the context of modern tools, by comparatively analyzing efficacy of lexicon-based approaches and end-to-end, ML-based approaches prevalent in state-of-the-art natural language processing systems. Our efforts using a large dataset show that even compared to an updated lexicon-based approach, end-to-end classification approaches are significantly more robust and accurate, even when trained by moderately sized corpora.
更多查看译文
关键词
Gender Bias, Gender Stereotypes, Machine Learning, Natural Language Processing, Lexicon
AI 理解论文
溯源树
样例
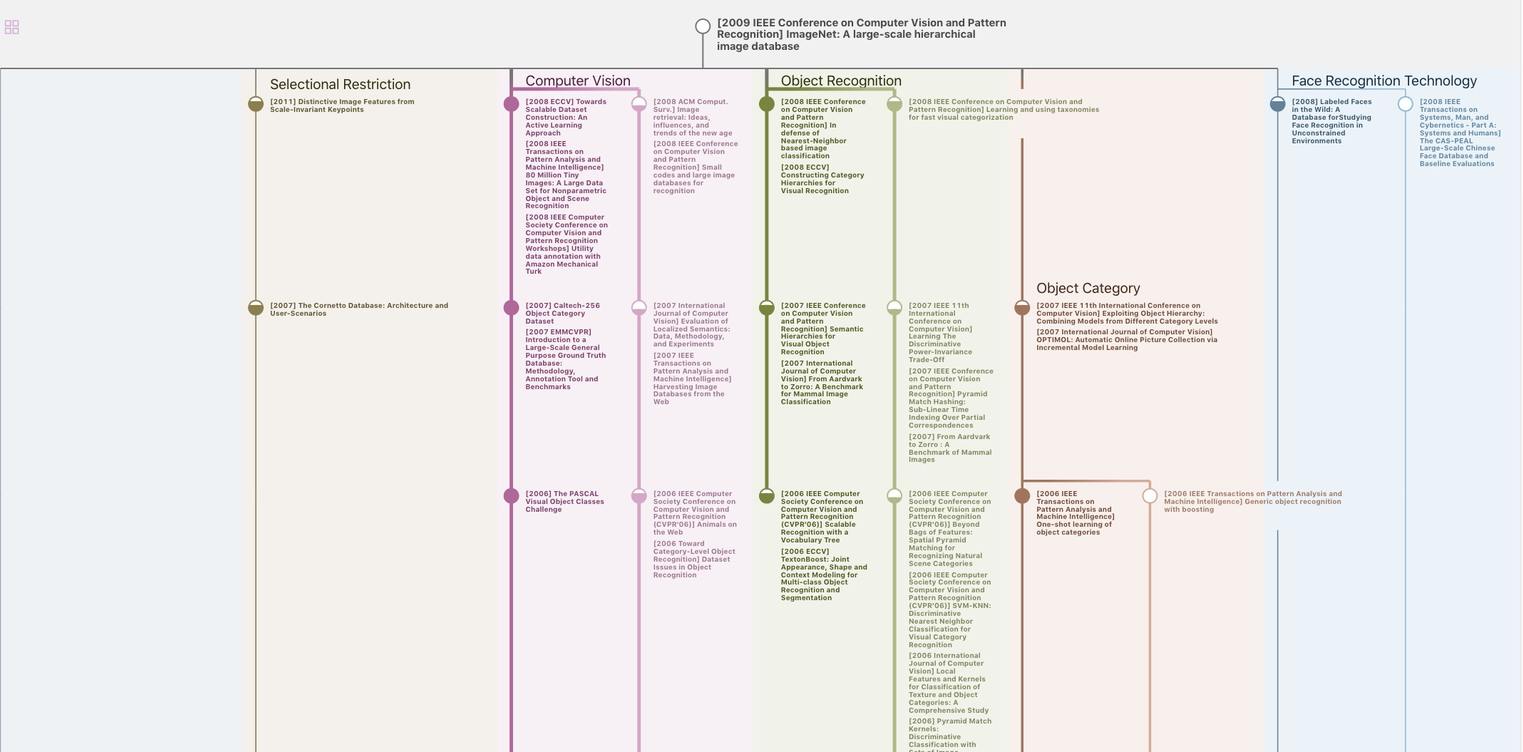
生成溯源树,研究论文发展脉络
Chat Paper
正在生成论文摘要