AutoCorrect - Deep Inductive Alignment of Noisy Geometric Annotations.
BMVC(2019)
摘要
We propose AutoCorrect, a method to automatically learn object-annotation alignments from a dataset with annotations affected by geometric noise. The method is based on a consistency loss that enables deep neural networks to be trained, given only noisy annotations as input, to correct the annotations. When some noise-free annotations are available, we show that the consistency loss reduces to a stricter self-supervised loss. We also show that the method can implicitly leverage object symmetries to reduce the ambiguity arising in correcting noisy annotations. When multiple object-annotation pairs are present in an image, we introduce a spatial memory map that allows the network to correct annotations sequentially, one at a time, while accounting for all other annotations in the image and corrections performed so far. Through ablation, we show the benefit of these contributions, demonstrating excellent results on geo-spatial imagery. Specifically, we show results using a new Railway tracks dataset as well as the public INRIA Buildings benchmarks, achieving new state-of-the-art results for the latter.
更多查看译文
AI 理解论文
溯源树
样例
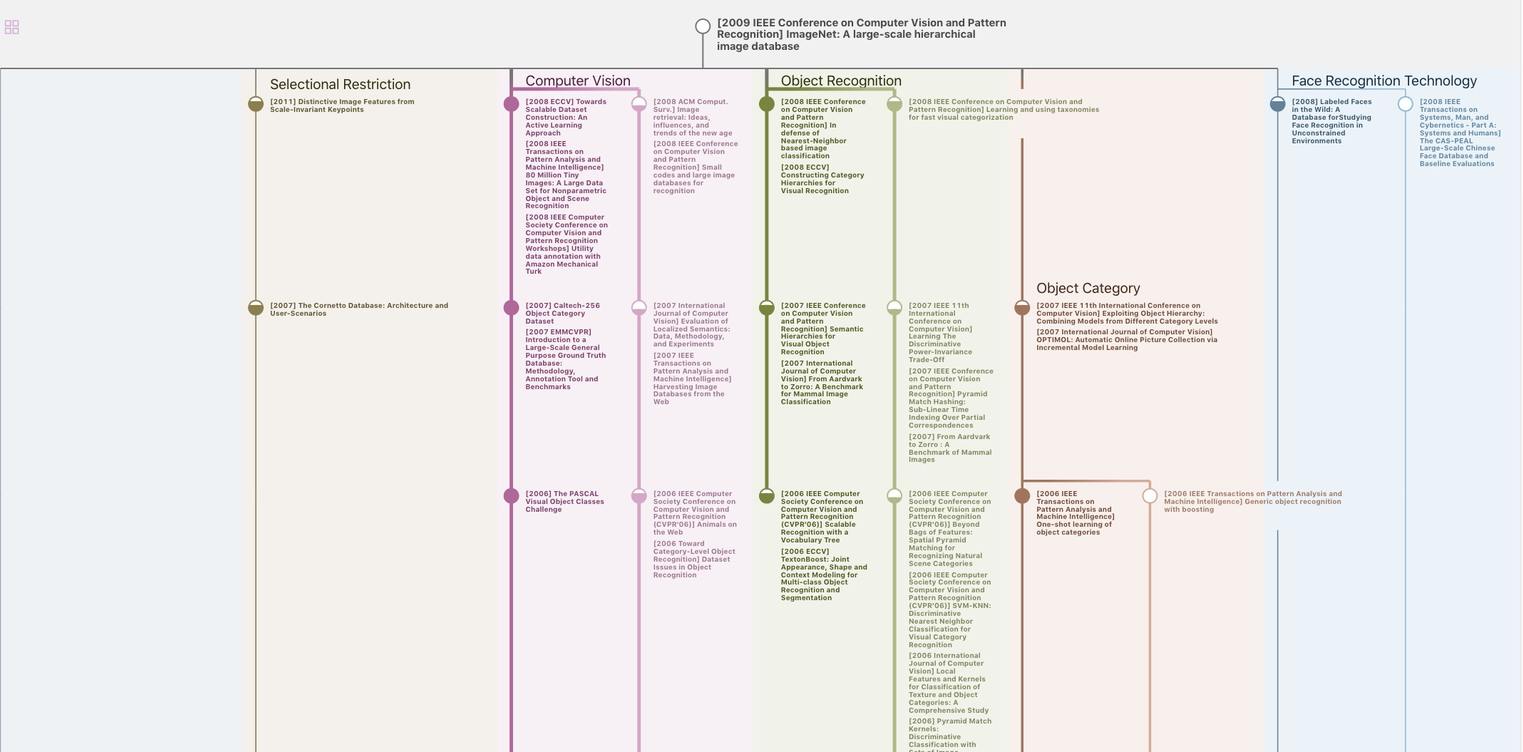
生成溯源树,研究论文发展脉络
Chat Paper
正在生成论文摘要