Binarized graph neural network
WORLD WIDE WEB-INTERNET AND WEB INFORMATION SYSTEMS(2021)
摘要
Recently, there have been some breakthroughs in graph analysis by applying the graph neural networks (GNNs) following a neighborhood aggregation scheme, which demonstrate outstanding performance in many tasks. However, we observe that the parameters of the network and the embedding of nodes are represented in real-valued matrices in existing GNN-based graph embedding approaches which may limit the efficiency and scalability of these models. It is well-known that binary vector is usually much more space and time efficient than the real-valued vector. This motivates us to develop a binarized graph neural network to learn the binary representations of the nodes with binary network parameters following the GNN-based paradigm. Our proposed method can be seamlessly integrated into the existing GNN-based embedding approaches to binarize the model parameters and learn the compact embedding. Extensive experiments indicate that the proposed binarized graph neural network, namely BGN, is orders of magnitude more efficient in terms of both time and space while matching the state-of-the-art performance.
更多查看译文
关键词
Graph neural network, Binarized neural network, Classification
AI 理解论文
溯源树
样例
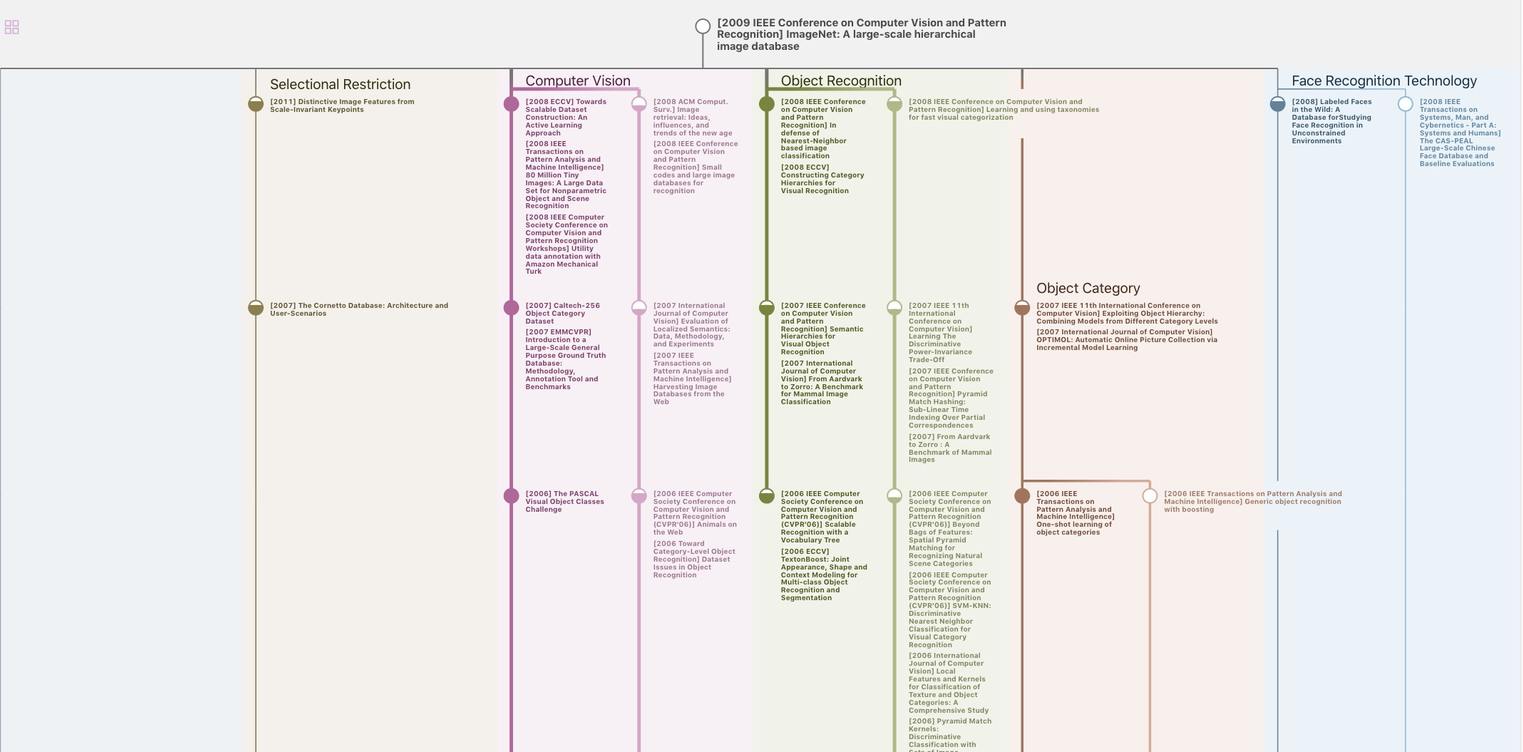
生成溯源树,研究论文发展脉络
Chat Paper
正在生成论文摘要