Sparse Generalized Canonical Correlation Analysis: Distributed Alternating Iteration based Approach
arxiv(2020)
摘要
Sparse canonical correlation analysis (CCA) is a useful statistical tool to detect latent information with sparse structures. However, sparse CCA works only for two datasets, i.e., there are only two views or two distinct objects. To overcome this limitation, in this paper, we propose a sparse generalized canonical correlation analysis (GCCA), which could detect the latent relations of multiview data with sparse structures. Moreover, the introduced sparsity could be considered as Laplace prior on the canonical variates. Specifically, we convert the GCCA into a linear system of equations and impose $\ell_1$ minimization penalty for sparsity pursuit. This results in a nonconvex problem on Stiefel manifold, which is difficult to solve. Motivated by Boyd's consensus problem, an algorithm based on distributed alternating iteration approach is developed and theoretical consistency analysis is investigated elaborately under mild conditions. Experiments on several synthetic and real world datasets demonstrate the effectiveness of the proposed algorithm.
更多查看译文
关键词
alternating iteration,correlation
AI 理解论文
溯源树
样例
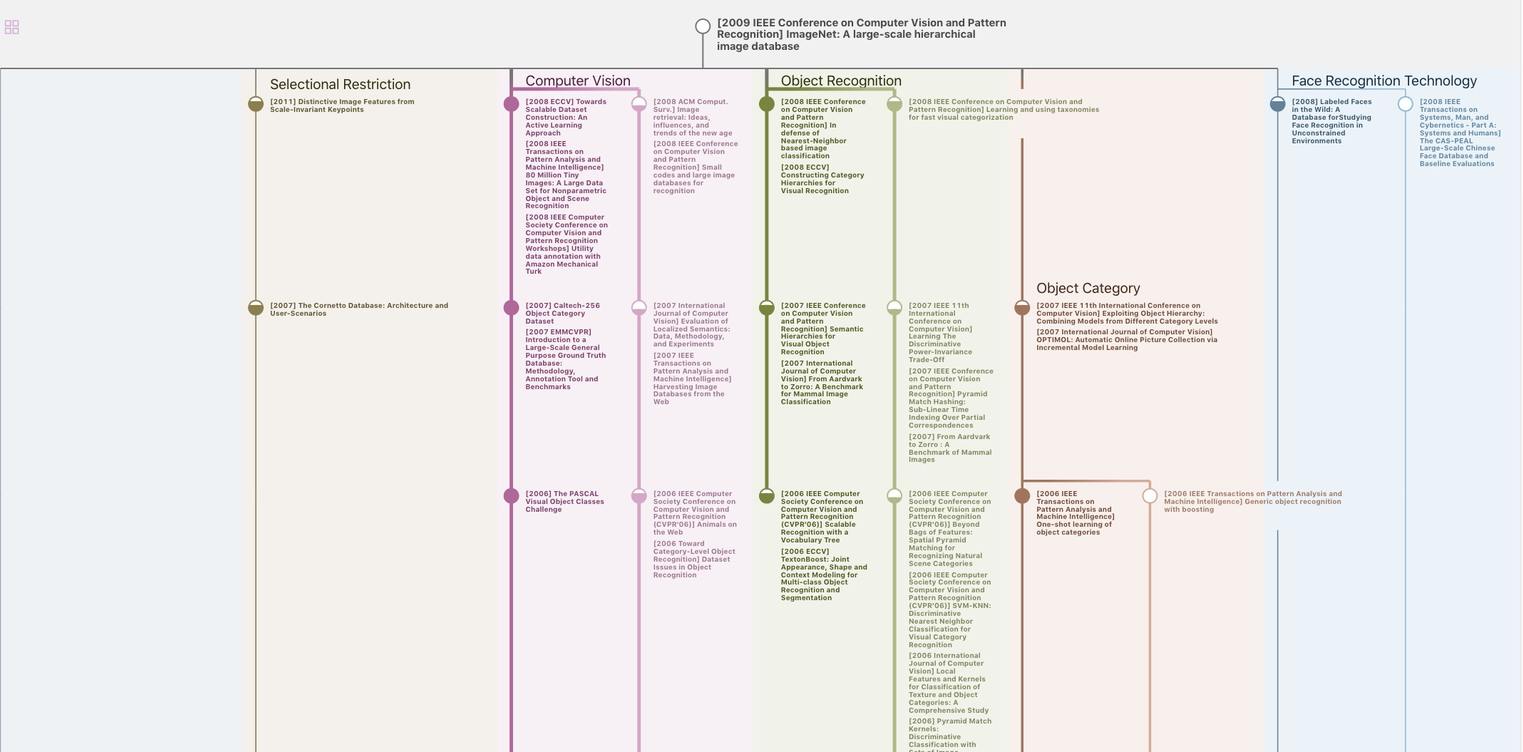
生成溯源树,研究论文发展脉络
Chat Paper
正在生成论文摘要