Bayesian Nonparametric Modeling for Predicting Dynamic Dependencies in Multiple Object Tracking
SENSORS(2022)
摘要
The paper considers the problem of tracking an unknown and time-varying number of unlabeled moving objects using multiple unordered measurements with unknown association to the objects. The proposed tracking approach integrates Bayesian nonparametric modeling with Markov chain Monte Carlo methods to estimate the parameters of each object when present in the tracking scene. In particular, we adopt the dependent Dirichlet process (DDP) to learn the multiple object state prior by exploiting inherent dynamic dependencies in the state transition using the dynamic clustering property of the DDP. Using the DDP to draw the mixing measures, Dirichlet process mixtures are used to learn and assign each measurement to its associated object identity. The Bayesian posterior to estimate the target trajectories is efficiently implemented using a Gibbs sampler inference scheme. A second tracking approach is proposed that replaces the DDP with the dependent Pitman-Yor process in order to allow for a higher flexibility in clustering. The improved tracking performance of the new approaches is demonstrated by comparison to the generalized labeled multi-Bernoulli filter.
更多查看译文
关键词
multiple object tracking, Monte Carlo sampling method, Bayesian nonparametric modeling, dependent Dirichlet process, dependent Pitman-Yor process
AI 理解论文
溯源树
样例
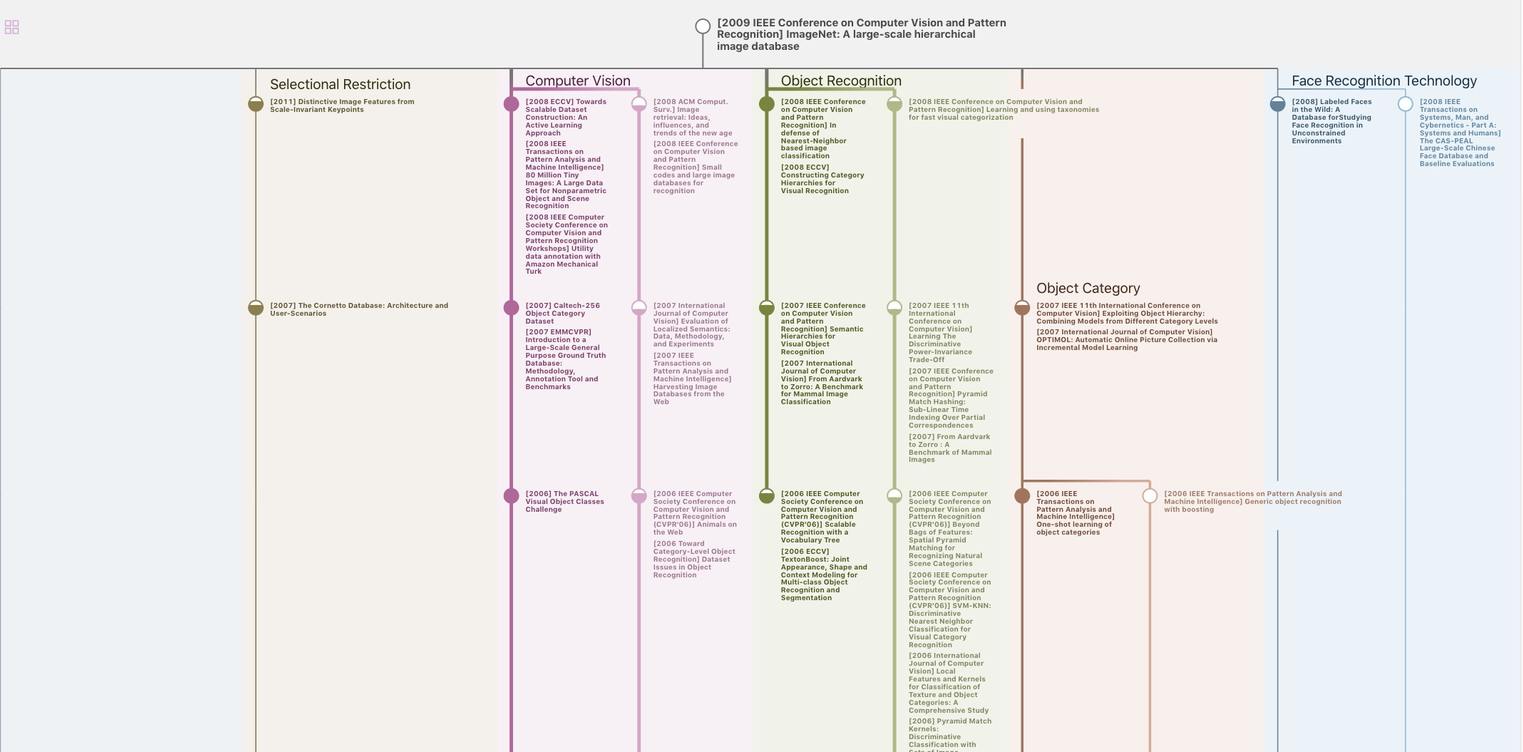
生成溯源树,研究论文发展脉络
Chat Paper
正在生成论文摘要