Passive Underwater Target Tracking: Conditionally Minimax Nonlinear Filtering with Bearing-Doppler Observations.
SENSORS(2020)
摘要
The paper presents an application of the Conditionally-Minimax Nonlinear Filtering (CMNF) algorithm to the online estimation of underwater vehicle movement given a combination of sonar and Doppler discrete-time noisy sensor observations. The proposed filter postulates recurrent "prediction-correction" form with some predefined basic prediction and correction terms, and then they are optimally fused. The CMNF estimates have the following advantageous features. First, the obtained estimates are unbiased. Second, the theoretical covariance matrix of CMNF errors meets the real values. Third, the CMNF algorithm gives a possibility to choose the preliminary observation transform, basic prediction, and correction functions in any specific case of the observation system to improve the estimate accuracy significantly. All the features of conditionally-minimax estimates are demonstrated by the regression example of random position estimate given the noisy bearing observations. The contribution of the paper is the numerical study of the CMNF algorithm applied to the underwater target tracking given bearing-only and bearing-Doppler observations.
更多查看译文
关键词
underwater target tracking,bearing-only measurements,bearing-Doppler measurements,port-starboard ambiguity,nonlinear filtering,conditionally minimax nonlinear filter,machine learning
AI 理解论文
溯源树
样例
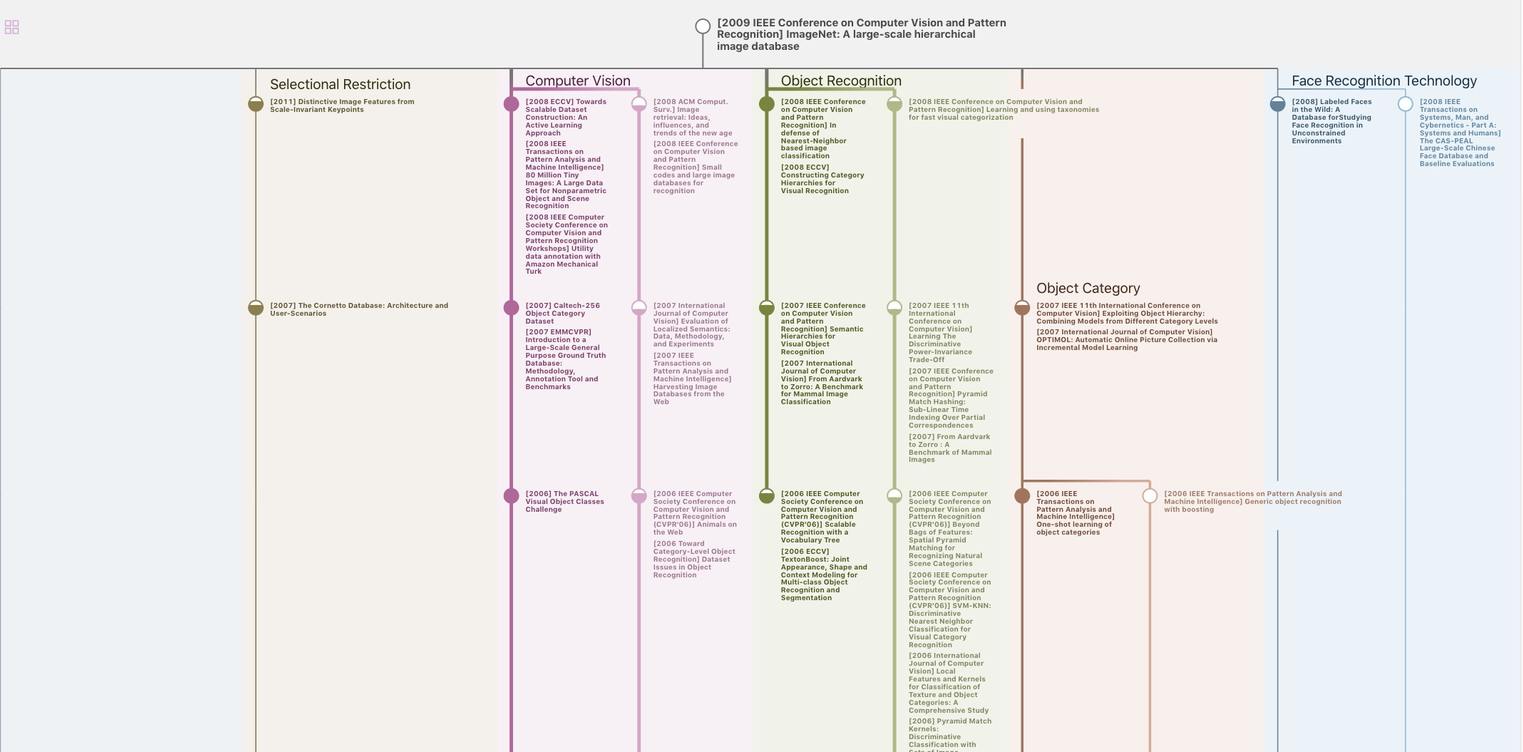
生成溯源树,研究论文发展脉络
Chat Paper
正在生成论文摘要