Provably robust deep generative models
arxiv(2020)
摘要
Recent work in adversarial attacks has developed provably robust methods for training deep neural network classifiers. However, although they are often mentioned in the context of robustness, deep generative models themselves have received relatively little attention in terms of formally analyzing their robustness properties. In this paper, we propose a method for training provably robust generative models, specifically a provably robust version of the variational auto-encoder (VAE). To do so, we first formally define a (certifiably) robust lower bound on the variational lower bound of the likelihood, and then show how this bound can be optimized during training to produce a robust VAE. We evaluate the method on simple examples, and show that it is able to produce generative models that are substantially more robust to adversarial attacks (i.e., an adversary trying to perturb inputs so as to drastically lower their likelihood under the model).
更多查看译文
关键词
robust deep generative models
AI 理解论文
溯源树
样例
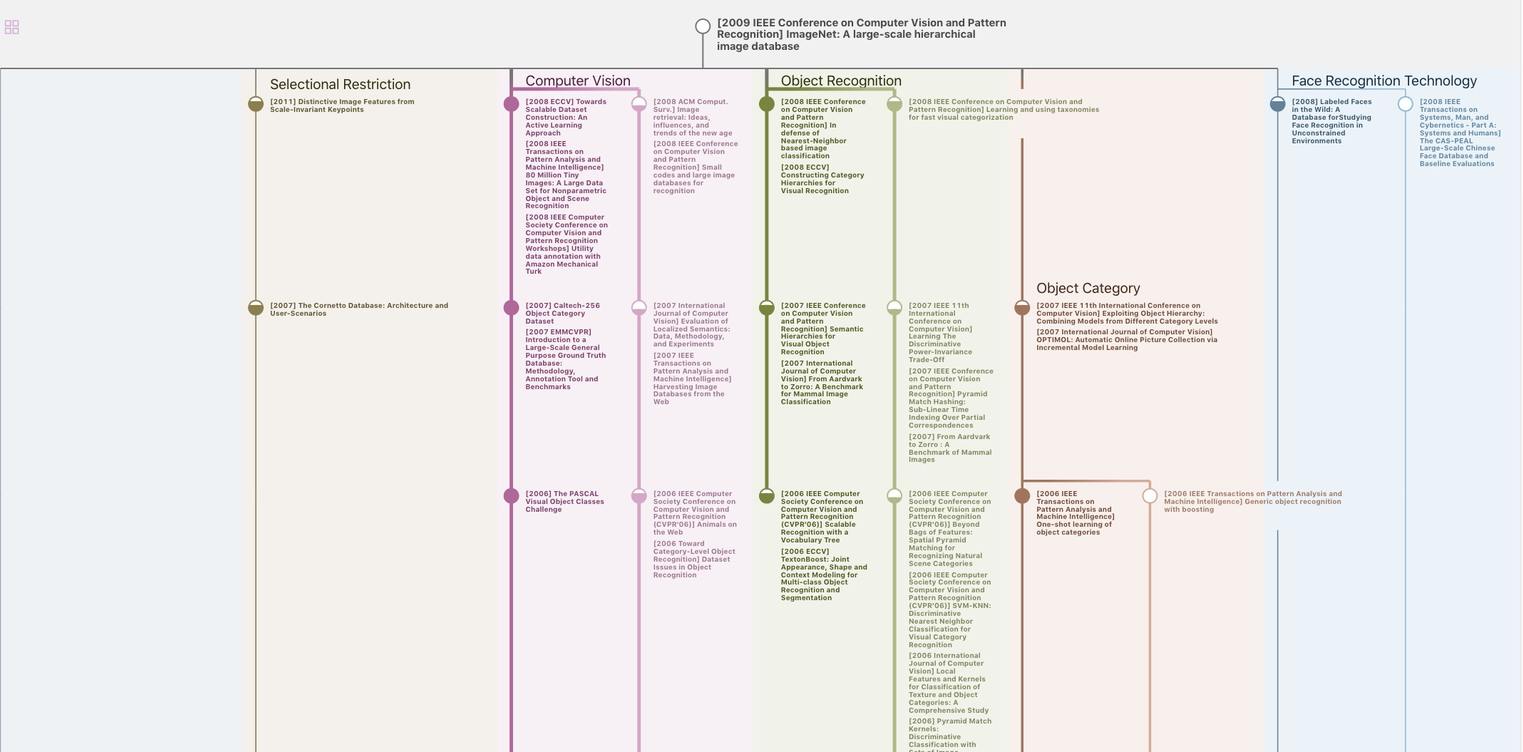
生成溯源树,研究论文发展脉络
Chat Paper
正在生成论文摘要