Inferring Degrees from Incomplete Networks and Nonlinear Dynamics
IJCAI 2020(2020)
摘要
Inferring topological characteristics of complex networks from observed data is critical to understand the dynamical behavior of networked systems, ranging from the Internet and the World Wide Web to biological networks and social networks. Prior studies usually focus on the structure-based estimation to infer network sizes, degree distributions, average degrees, and more. Little effort attempted to estimate the specific degree of each vertex from a sampled induced graph, which prevents us from measuring the lethality of nodes in protein networks and influencers in social networks. The current approaches dramatically fail for a tiny sampled induced graph and require a specific sampling method and a large sample size. These approaches neglect information of the vertex state, representing the dynamical behavior of the networked system, such as the biomass of species or expression of a gene, which is useful for degree estimation. We fill this gap by developing a framework to infer individual vertex degrees using both information of the sampled topology and vertex state. We combine the mean-field theory with combinatorial optimization to learn vertex degrees. Experimental results on real networks with a variety of dynamics demonstrate that our framework can produce reliable degree estimates and dramatically improve existing link prediction methods by replacing the sampled degrees with our estimated degrees.
更多查看译文
关键词
incomplete networks,nonlinear dynamics,degrees
AI 理解论文
溯源树
样例
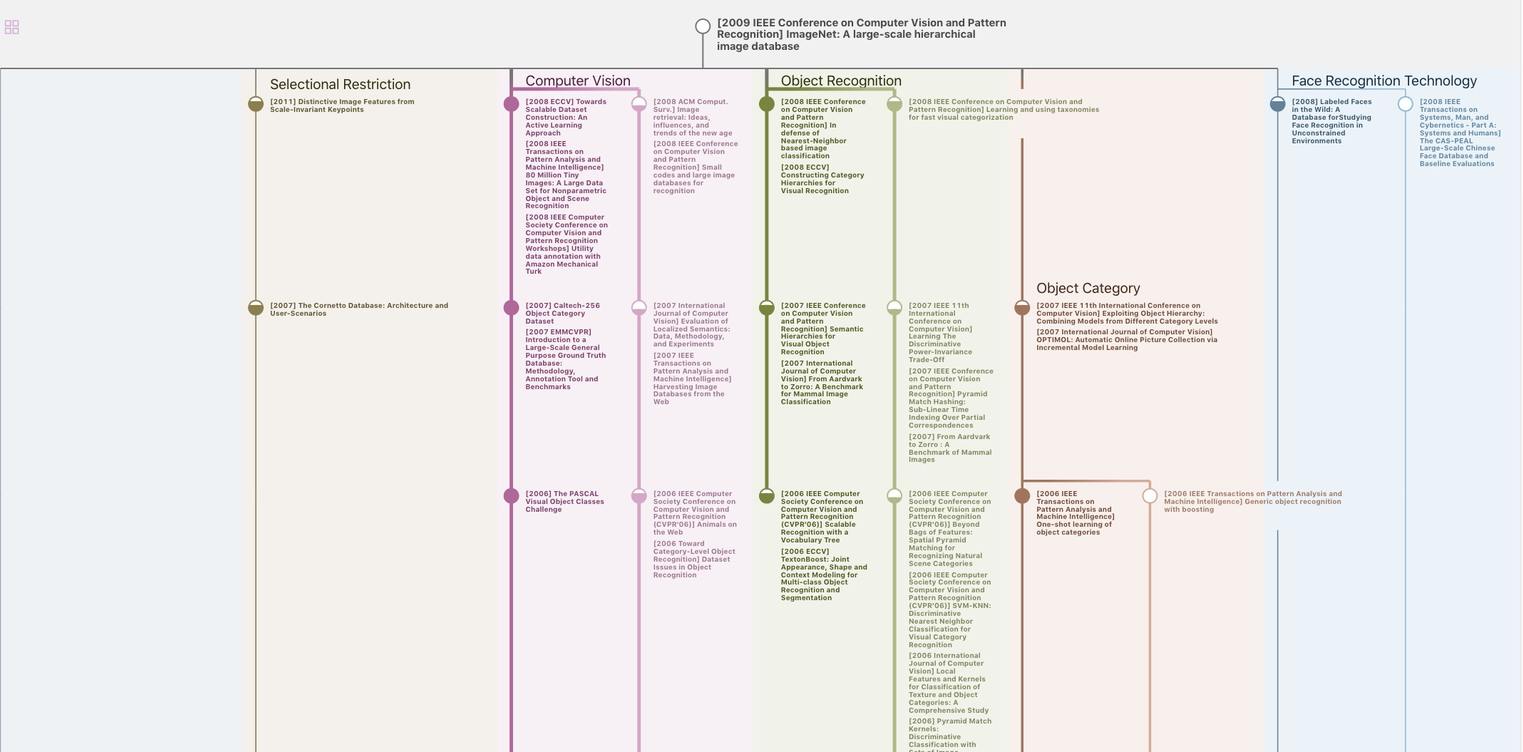
生成溯源树,研究论文发展脉络
Chat Paper
正在生成论文摘要