Trading Off Diversity and Quality in Natural Language Generation
Proceedings of the Workshop on Human Evaluation of NLP Systems (HumEval)(2020)
摘要
For open-ended language generation tasks such as storytelling or dialogue, choosing the right decoding algorithm is vital for controlling the tradeoff between generation quality and diversity. However, there presently exists no consensus on which decoding procedure is best or even the criteria by which to compare them. In this paper, we cast decoding as a tradeoff between response quality and diversity, and we perform the first large-scale evaluation of decoding methods along the entire quality-diversity spectrum. Our experiments confirm the existence of the likelihood trap: the counter-intuitive observation that high likelihood sequences are often surprisingly low quality. We also find that when diversity is a priority, all methods perform similarly, but when quality is viewed as more important, nucleus sampling (Holtzman et al., 2019) outperforms all other evaluated decoding algorithms.
更多查看译文
关键词
generation,off diversity,language
AI 理解论文
溯源树
样例
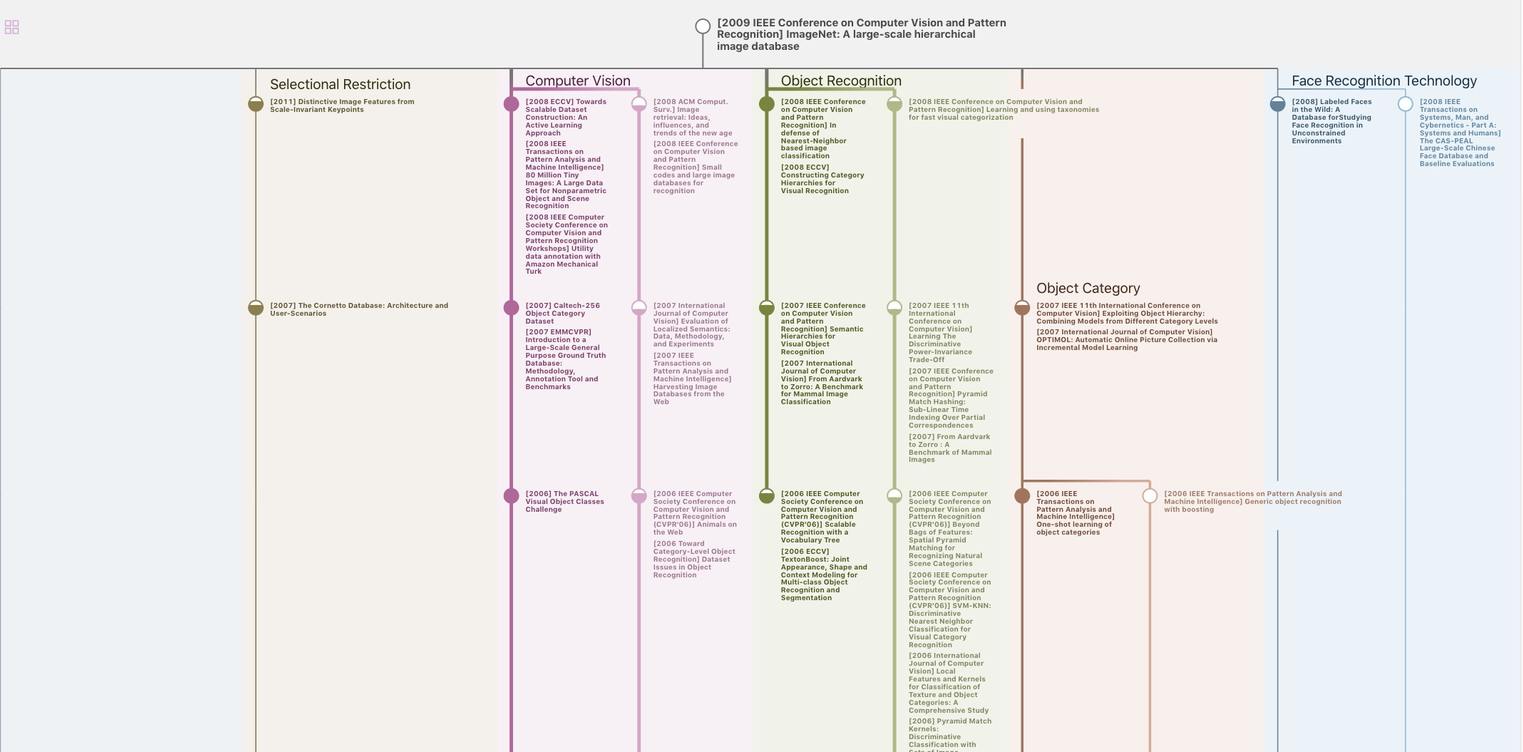
生成溯源树,研究论文发展脉络
Chat Paper
正在生成论文摘要