TAEN: Temporal Aware Embedding Network for Few-Shot Action Recognition
2021 IEEE/CVF CONFERENCE ON COMPUTER VISION AND PATTERN RECOGITION WORKSHOPS (CVPRW 2021)(2021)
摘要
Classification of new class entities requires collecting and annotating hundreds or thousands of samples that is often prohibitively costly. Few-shot learning suggests learning to classify new classes using just a few examples. Only a small number of studies address the challenge of few-shot learning on spatio-temporal patterns such as videos. In this paper, we present the Temporal Aware Embedding Network (TAEN) for few-shot action recognition, that learns to represent actions, in a metric space as a trajectory, conveying both short term semantics and longer term connectivity between action parts. We demonstrate the effectiveness of TAEN on two few shot tasks, video classification and temporal action detection and evaluate our method on the Kinetics-400 and on ActivityNet 1.2 few-shot benchmarks. With training of just a few fully connected layers we reach comparable results to prior art on both few shot video classification and temporal detection tasks, while reaching state-of-the-art in certain scenarios.
更多查看译文
关键词
longer term connectivity,action parts,TAEN,shot tasks,temporal action detection,few-shot benchmarks,shot video classification,temporal detection tasks,Temporal Aware Embedding Network,few-shot action recognition,class entities,few-shot learning,spatio-temporal patterns,short term semantics
AI 理解论文
溯源树
样例
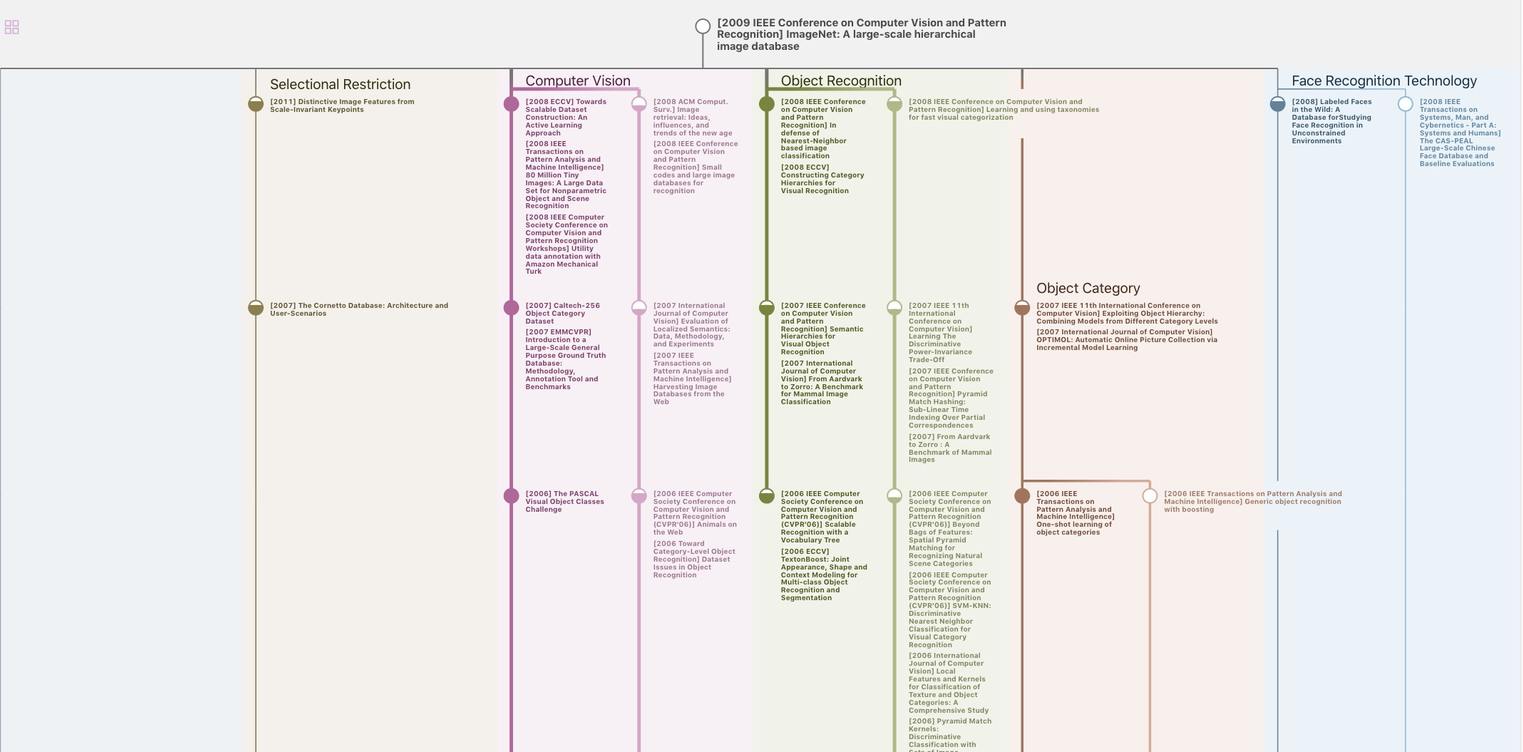
生成溯源树,研究论文发展脉络
Chat Paper
正在生成论文摘要