Alleviating the Incompatibility between Cross Entropy Loss and Episode Training for Few-shot Skin Disease Classification
medical image computing and computer assisted intervention(2020)
摘要
Skin disease classification from images is crucial to dermatological diagnosis. However, identifying skin lesions involves a variety of aspects in terms of size, color, shape, and texture. To make matters worse, many categories only contain very few samples, posing great challenges to conventional machine learning algorithms and even human experts. Inspired by the recent success of Few-Shot Learning (FSL) in natural image classification, we propose to apply FSL to skin disease identification to address the extreme scarcity of training samples. However, directly applying FSL to this task does not work well in practice, and we find that the problem should be largely attributed to the incompatibility between Cross Entropy (CE) and episode training, which are both commonly used in FSL. Based on a detailed analysis, we propose the Query-Relative (QR) loss, which proves superior to CE under episode training and is closely related to recently proposed mutual information estimation. Moreover, we further strengthen the proposed QR loss with a novel adaptive hard margin strategy. Comprehensive experiments validate the effectiveness of the proposed FSL scheme and the possibility to diagnosis rare skin disease with a few labeled samples.
更多查看译文
关键词
Few-shot skin disease classification, Query-relative loss
AI 理解论文
溯源树
样例
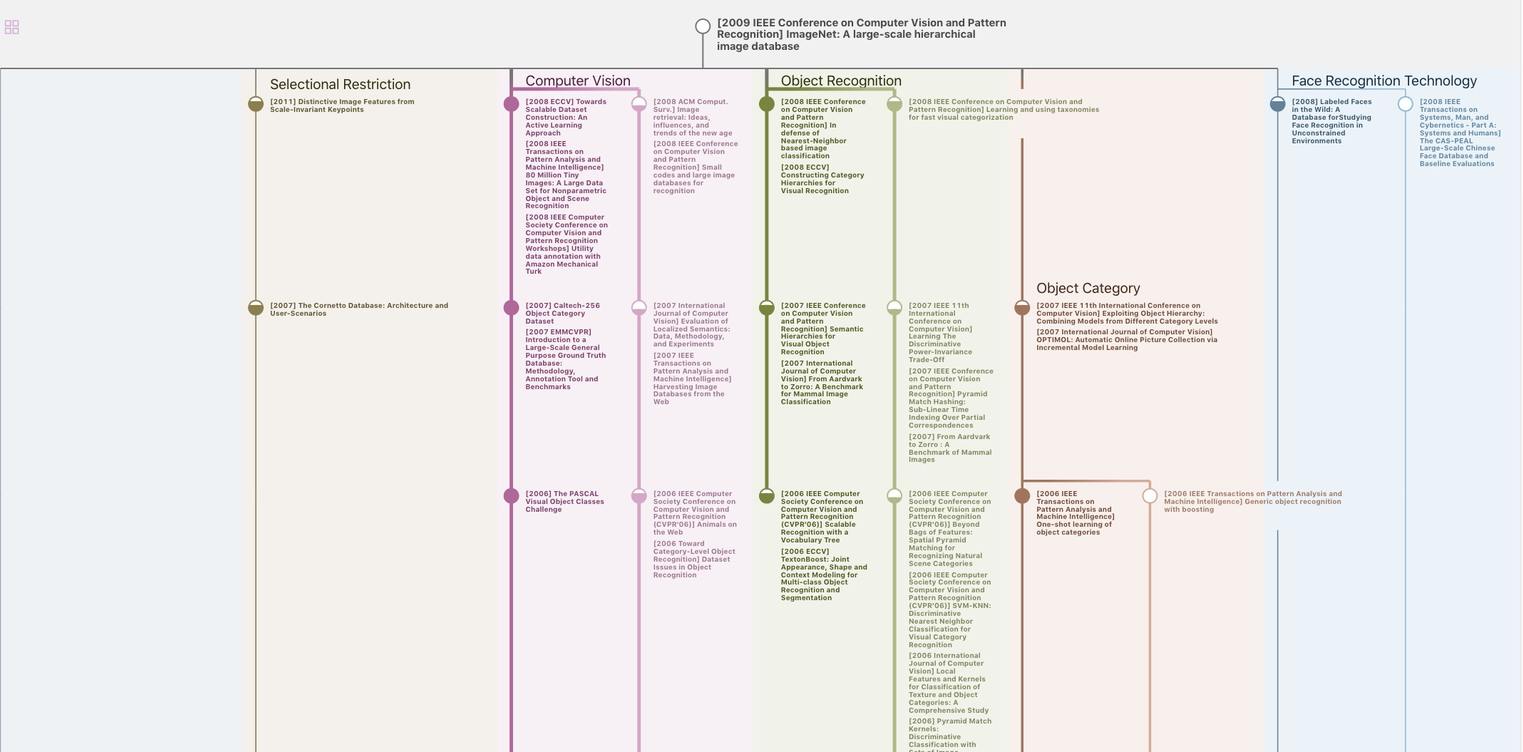
生成溯源树,研究论文发展脉络
Chat Paper
正在生成论文摘要